LSTM-DNN Based Autoencoder Network for Nonlinear Hyperspectral Image Unmixing
IEEE Journal of Selected Topics in Signal Processing(2021)
摘要
Blind hyperspectral unmixing is an important technique in hyperspectral image analysis, aiming at estimating endmembers and their respective fractional abundances. Consider the limitations of using the linear model, nonlinear unmixing methods have been studied under different model assumptions. However, existing nonlinear unmixing algorithms do not fully exploit spectral and spatial correlation information. This paper proposes a nonsymmetric autoencoder network to overcome this issue. The proposed scheme benefits from the universal modeling ability of deep neural networks and enables to learn the nonlinear relation from the data. Particularly, the long short-term memory network (LSTM) structure is included to capture spectral correlation information, and a spatial regularization is introduced to improve the spatial continuity of results. An attention mechanism is also used to further enhance the unmixing performance. Experiments with synthetic and real data are conducted to illustrate the effectiveness of the proposed method.
更多查看译文
关键词
Hyperspectral unmixing,attention recurrent neural network,autoencoder network,nonlinear unmixing
AI 理解论文
溯源树
样例
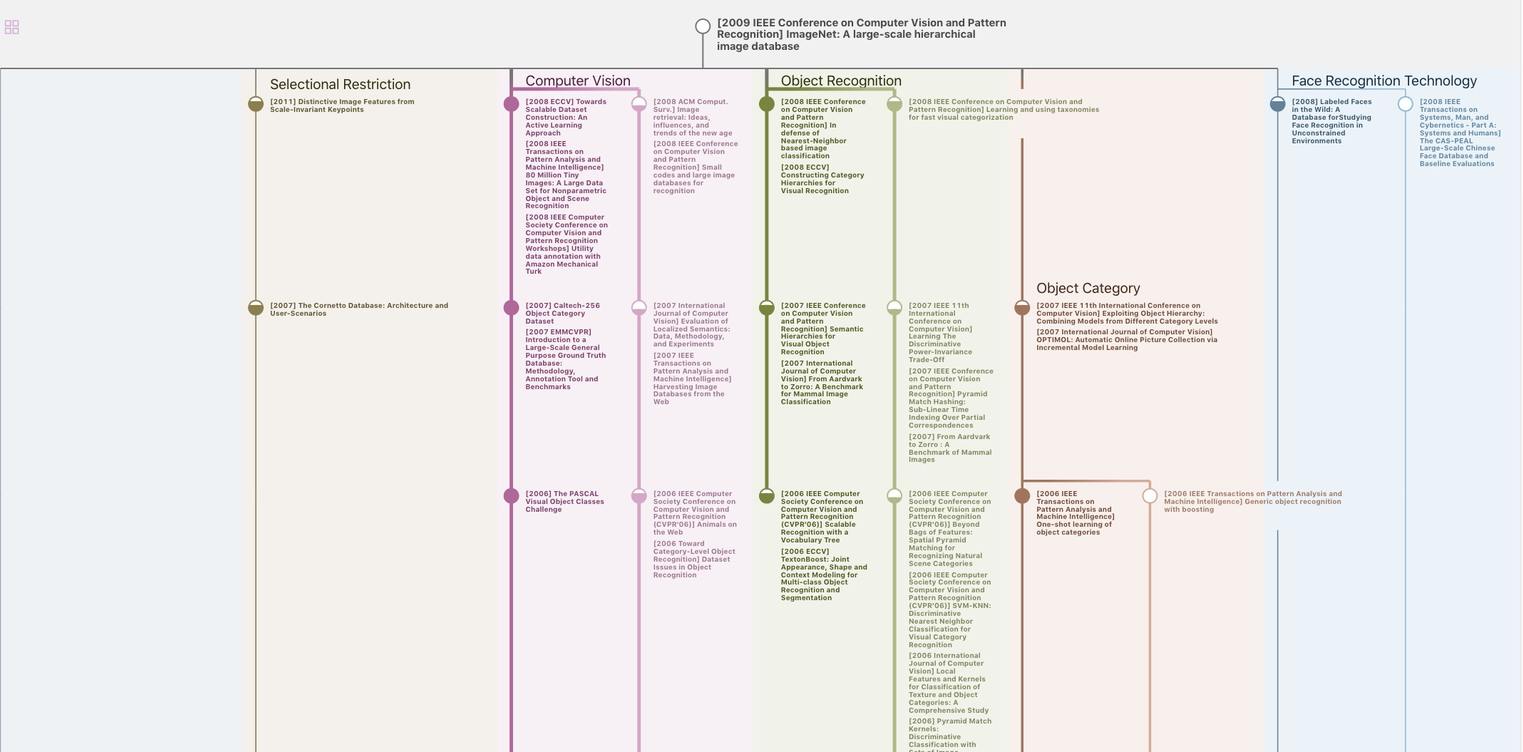
生成溯源树,研究论文发展脉络
Chat Paper
正在生成论文摘要