A Closed Form Solution to Best Rank-1 Tensor Approximation via KL divergence Minimization
ArXiv(2021)
摘要
Tensor decomposition is a fundamentally challenging problem. Even the simplest case of tensor decomposition, the rank-1 approximation in terms of the Least Squares (LS) error, is known to be NP-hard. Here, we show that, if we consider the KL divergence instead of the LS error, we can analytically derive a closed form solution for the rank-1 tensor that minimizes the KL divergence from a given positive tensor. Our key insight is to treat a positive tensor as a probability distribution and formulate the process of rank-1 approximation as a projection onto the set of rank-1 tensors. This enables us to solve rank-1 approximation by convex optimization. We empirically demonstrate that our algorithm is an order of magnitude faster than the existing rank-1 approximation methods and gives better approximation of given tensors, which supports our theoretical finding.
更多查看译文
关键词
fast tucker rank reduction,non-negative,mean-field
AI 理解论文
溯源树
样例
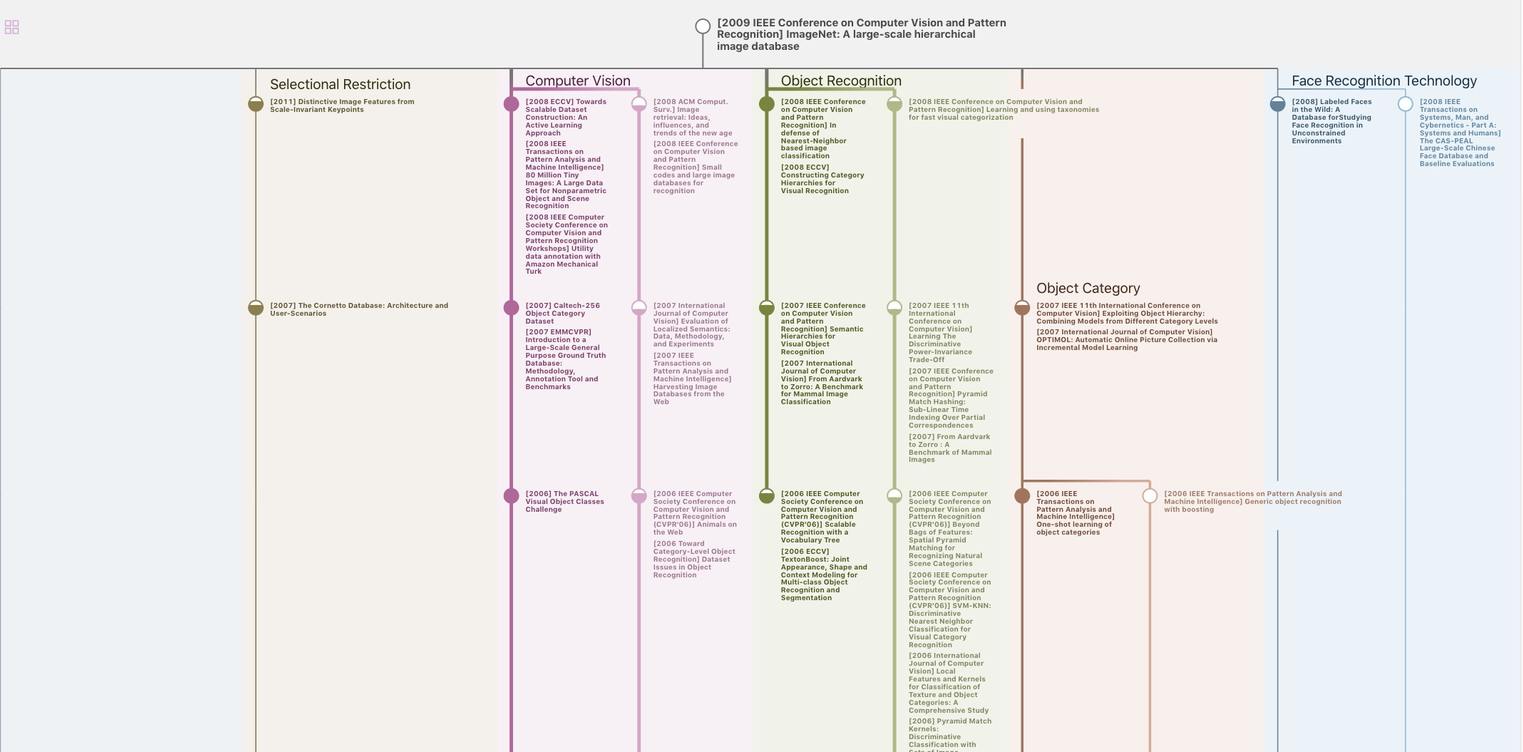
生成溯源树,研究论文发展脉络
Chat Paper
正在生成论文摘要