Dynamic-Static-based Spatiotemporal Multi-Graph Neural Networks for Passenger Flow Prediction
2020 IEEE 26th International Conference on Parallel and Distributed Systems (ICPADS)(2020)
摘要
Various sensing and computing technologies have gradually outlined the future of the intelligent city. Passenger flow prediction of public transports has become an important task in Intelligent Transportation System (ITS), which is the prerequisite for traffic management and urban planning. There exist many methods based on deep learning for learning the spatiotemporal features from high non-linearity and complexity of traffic flows. However, they only utilize temporal correlation and static spatial correlation, such as geographical distance, which is insufficient in the mining of dynamic spatial correlation. In this paper, we propose the Dynamic-Static-based Spatiotemporal Multi-Graph Neural Networks model (DSSTMG) for predicting traffic passenger flows, which can concurrently incorporate the temporal and multiple static and dynamic spatial correlations. Firstly, we exploit the multiple static spatial correlations by multi-graph fusion convolution operator, including adjacent relation, station functional zone similarity and geographical distance. Secondly, we exploit the spatial dynamic correlations by calculating the similarity between the flow pattern of stations over a period of time, and build the dynamic spatial attention. Moreover, we use time attention and encoder-decoder architecture to capture temporal correlation. The experimental results on two realworld datasets show that the proposed DSSTMG outperforms state-of-the-art methods.
更多查看译文
关键词
Passenger Flow Prediction,Multi-Graph Fusion,Spatiotemporal Correlations Mining,Attention
AI 理解论文
溯源树
样例
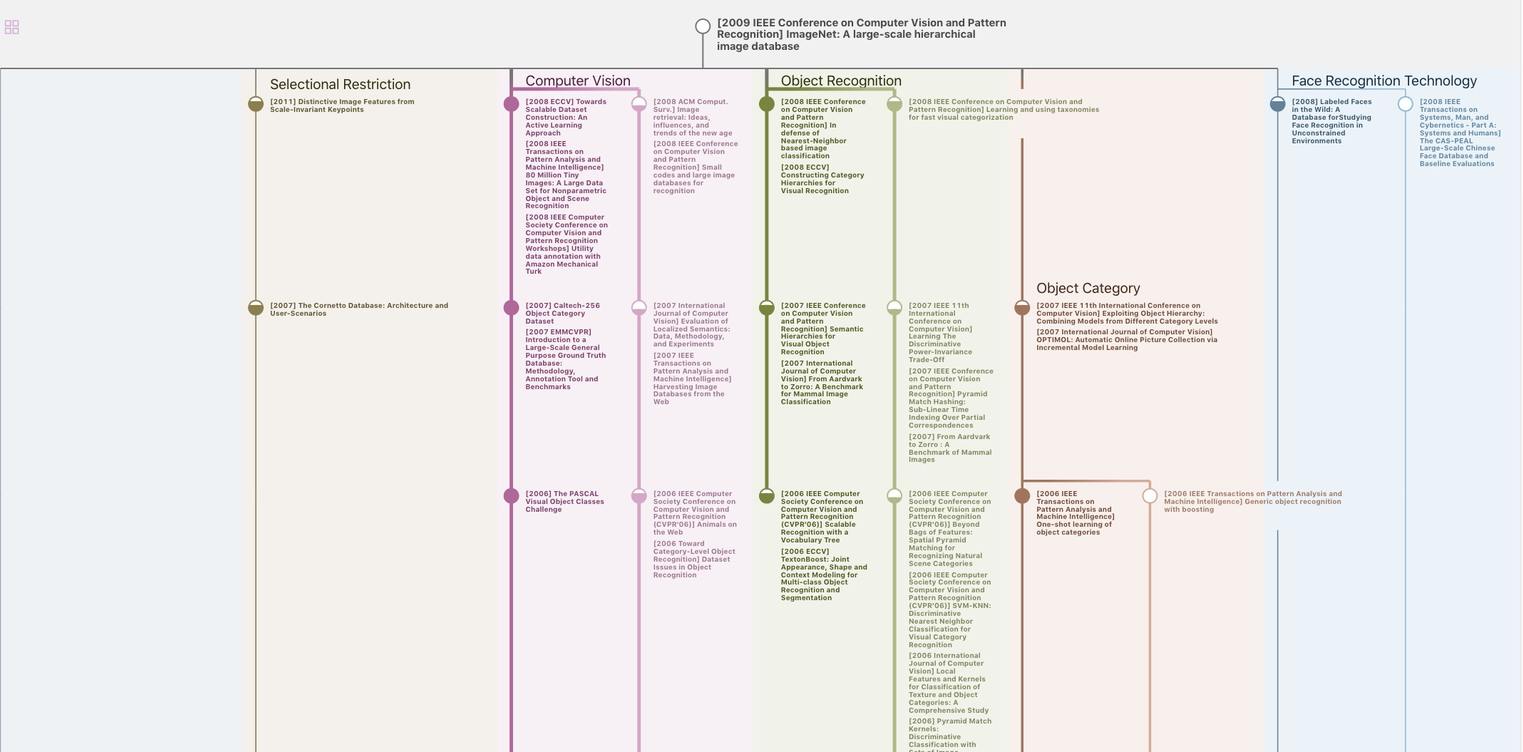
生成溯源树,研究论文发展脉络
Chat Paper
正在生成论文摘要