Automated Semantic Segmentation of Cardiac Magnetic Resonance Images with Deep Learning.
ICMLA(2020)
摘要
Machine learning algorithms, especially deep learning architectures, have demonstrated immense potential for biomedical segmentation, often surpassing expert-level performance. For cardiac magnetic resonance (CMR) imaging, semantic segmentation is critical to deriving clinical measures such as myocardial mass and volume. However, challenges still exist. Manual delineation by domain experts is time-consuming and subject to human errors. With semi-automated segmentation techniques, it is challenging to analyze images of the same subject twice, end-diastole and end-systole of the cardiac cycle. To address these challenges, we propose a deep learning-based end-to-end analytical pipeline for automated segmentation of short-axis CMR imaging. The automated pipeline successfully avoids the problem of human subjectivity and achieves expert-level segmentation accuracy. With a large heterogeneous data inclusive of subjects with varying conditions, our model overcomes the data-homogeneity and achieves 99.9% dice similarity score, which outperforms the current state-of-art work.
更多查看译文
关键词
cardiac magnetic resonance (CMR) imaging,semantic segmentation,deep learning,U-Net,Fully Convolutional Network
AI 理解论文
溯源树
样例
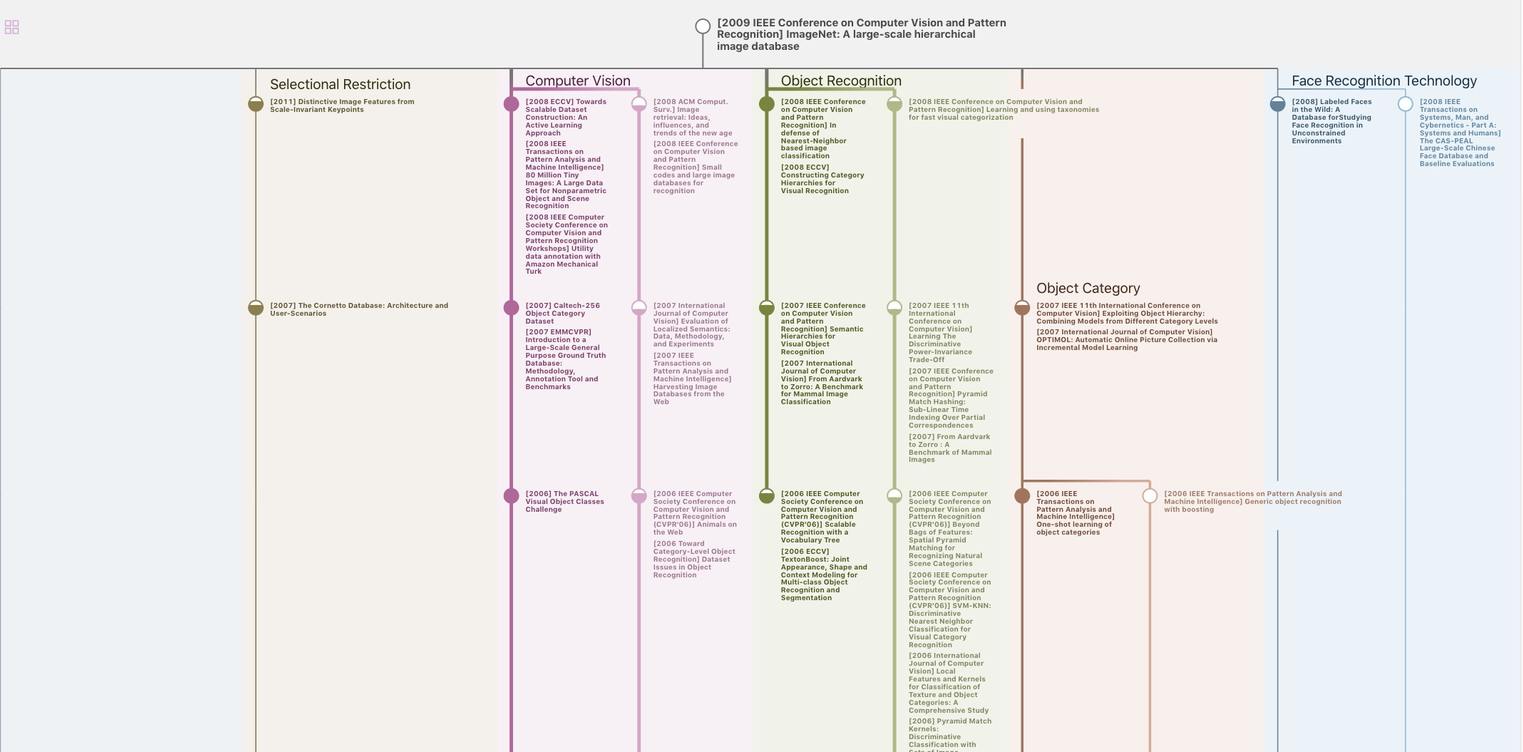
生成溯源树,研究论文发展脉络
Chat Paper
正在生成论文摘要