Low Energy Ecg Features Extraction For Atrial Fibrillation Detection In Wearable Sensors
PROCEEDINGS OF THE 10TH INTERNATIONAL CONFERENCE ON SENSOR NETWORKS (SENSORNETS)(2021)
摘要
The Internet of Health Things plays a key role in the transformation of health care systems as it enables wearable health monitoring systems to ensure continuous and non-invasive tracking of vital body parameters. To successfully detect the cardiac problem of Atrial Fibrillation (AF) wearable sensors are required to continuously sense and transmit ECG signals. The traditional approach of ECG streaming over energy-consuming wireless links can overwhelm the limited energy resources of wearable sensors. This paper proposes a low-energy features' extraction method that combines the RR interval and P wave features for higher AF detection accuracy. In the proposed scheme, instead of streaming raw ECG signals , local AF features extraction is executed on the sensors. Results have shown that combining time-domain features with wavelet extracted features, achieved a sensitivity of 98.59% and a specificity of 97.61%. In addition, compared to ECG streaming, on-sensor AF detection achieved a 92% gain in energy savings.
更多查看译文
关键词
ECG Signal Processing, Atrial Fibrillation, Wavelet Analysis, Features Extraction, WBSN
AI 理解论文
溯源树
样例
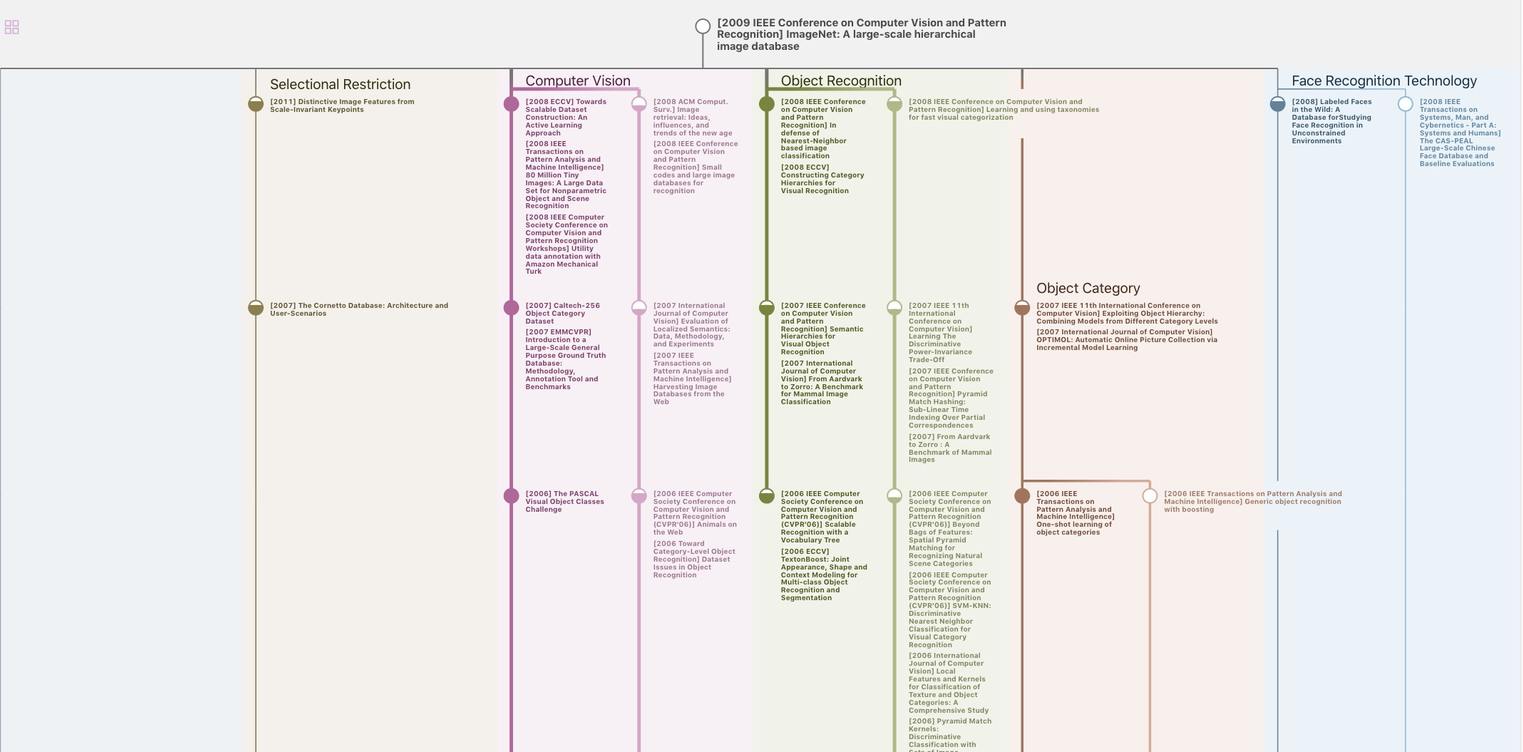
生成溯源树,研究论文发展脉络
Chat Paper
正在生成论文摘要