Joint Depth And Defocus Estimation From A Single Image Using Physical Consistency
IEEE TRANSACTIONS ON IMAGE PROCESSING(2021)
摘要
Estimating depth and defocus maps are two fundamental tasks in computer vision. Recently, many methods explore these two tasks separately with the help of the powerful feature learning ability of deep learning and these methods have achieved impressive progress. However, due to the difficulty in densely labeling depth and defocus on real images, these methods are mostly based on synthetic training dataset, and the performance of learned network degrades significantly on real images. In this paper, we tackle a new task that jointly estimates depth and defocus from a single image. We design a dual network with two subnets respectively for estimating depth and defocus. The network is jointly trained on synthetic dataset with a physical constraint to enforce the physical consistency between depth and defocus. Moreover, we design a simple method to label depth and defocus order on real image dataset, and design two novel metrics to measure accuracies of depth and defocus estimation on real images. Comprehensive experiments demonstrate that joint training for depth and defocus estimation using physical consistency constraint enables these two subnets to guide each other, and effectively improves their depth and defocus estimation performance on real defocused image dataset.
更多查看译文
关键词
Estimation, Task analysis, Cameras, Feature extraction, Training, Neural networks, Sun, Depth estimation, defocus estimation, physical consistency, real dataset, joint network training
AI 理解论文
溯源树
样例
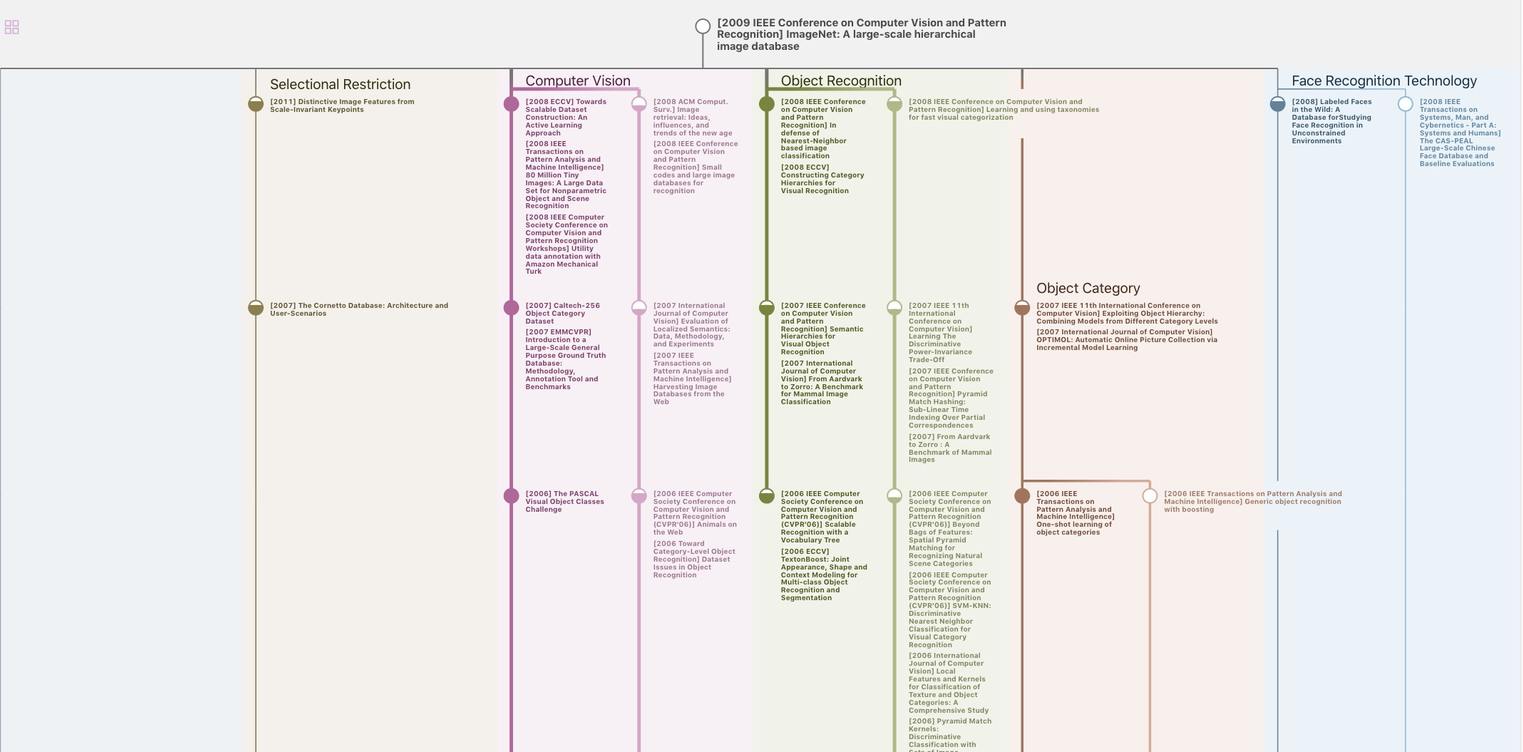
生成溯源树,研究论文发展脉络
Chat Paper
正在生成论文摘要