Deep Learning Type Convolution Neural Network Architecture For Multiclass Classification Of Alzheimer'S Disease
BIOIMAGING: PROCEEDINGS OF THE 14TH INTERNATIONAL JOINT CONFERENCE ON BIOMEDICAL ENGINEERING SYSTEMS AND TECHNOLOGIES - VOL. 2: BIOIMAGING(2021)
摘要
Alzheimer's disease (AD) is one of the common medical issues that the world is facing today. This disease has a high prevalence of memory loss and cognitive decline primarily in the elderly. At present, there is no specific treatment for this disease, but it is thought that identification of it at an early stage can help to manage it in a better way. Several studies used machine learning (ML) approaches for AD diagnosis and classification. In this study, we considered the Open Access Series of Imaging Studies-3 (OASIS-3) dataset with 2,168 Magnetic Resonance Imaging (MRI) images of patients with very mild to different stages of cognitive decline. We applied deep learning-based convolution neural networks (CNN) which are well-known approaches for diagnosis-based studies. The model training was done by 70% of images and applied 10-fold cross-validation to validate the model. The developed architecture model has successfully classified the different stages of dementia images and achieved 83.3% accuracy which is higher than other traditional classification techniques like support vectors and logistic regression.
更多查看译文
关键词
Alzheimer's Disease (AD), OASIS-3, MRI Images, Deep Learning, CNN
AI 理解论文
溯源树
样例
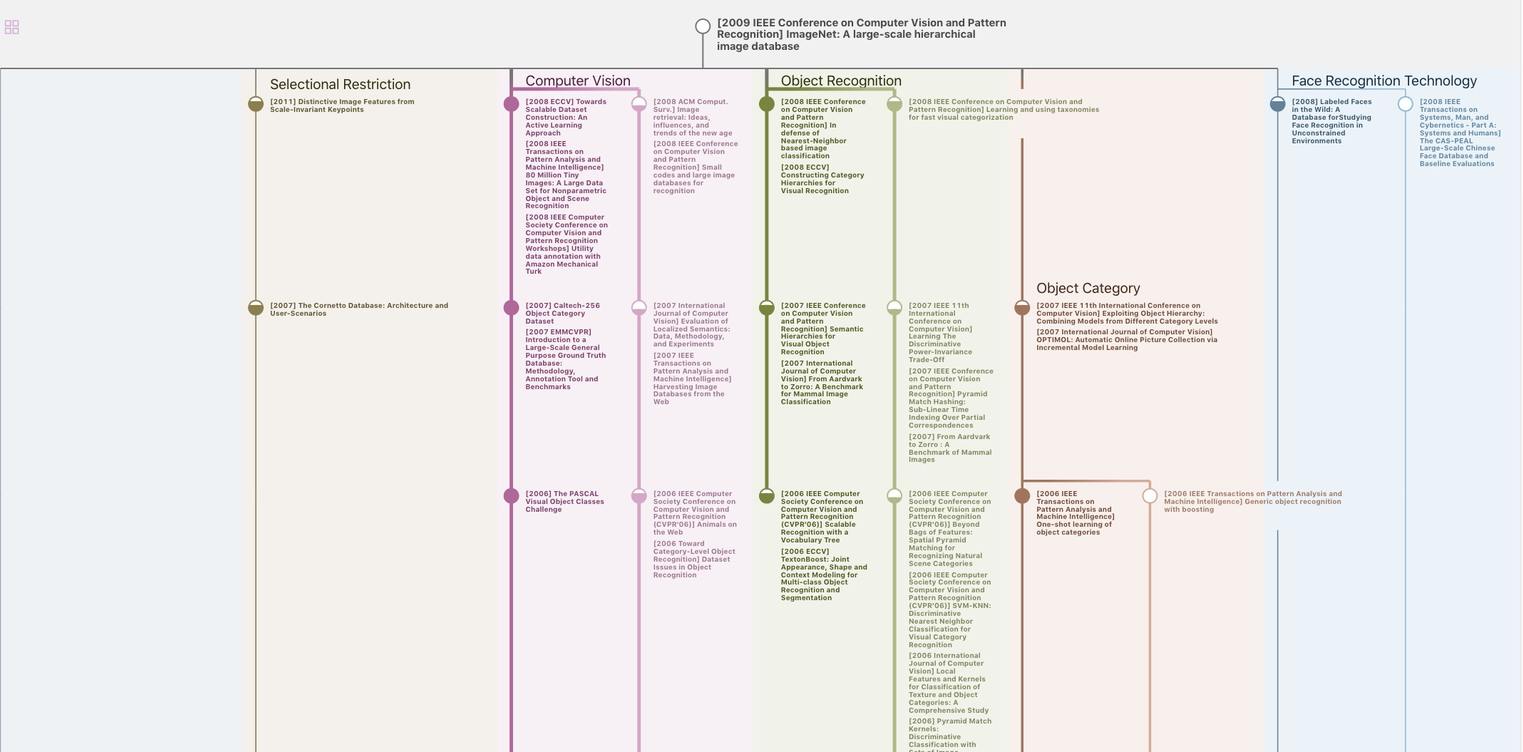
生成溯源树,研究论文发展脉络
Chat Paper
正在生成论文摘要