What Do You Mean, Doctor? A Knowledge-based Approach for Word Sense Disambiguation of Medical Terminology.
Proceedings of the 14th International Joint Conference on Biomedical Engineering Systems and Technologies(2021)
Key words
Medical Word Sense Disambiguation,Knowledge-based,Semantic Similarity,Word Embeddings,Data Understanding
AI Read Science
Must-Reading Tree
Example
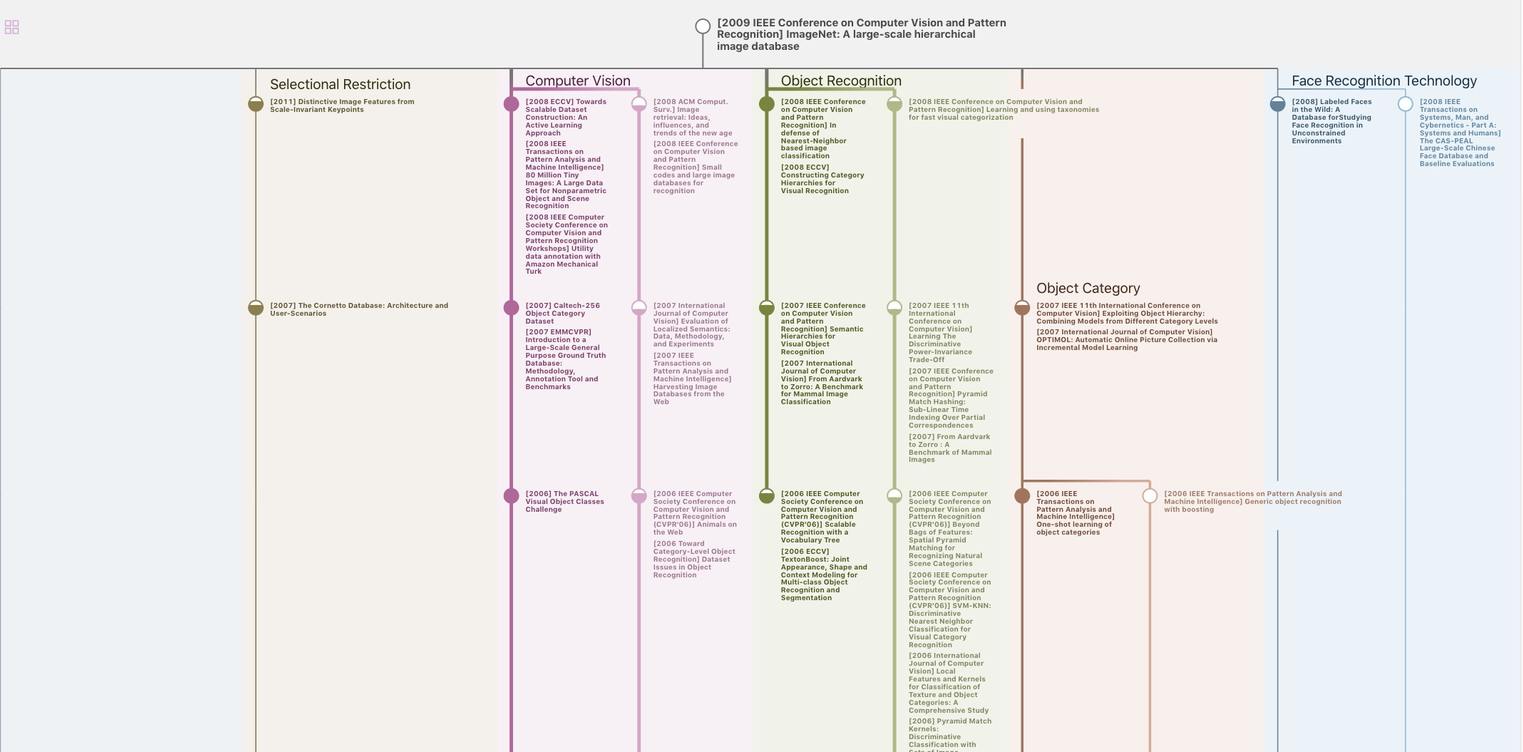
Generate MRT to find the research sequence of this paper
Chat Paper
Summary is being generated by the instructions you defined