Implementation Of Quantum Machine Learning For Electronic Structure Calculations Of Periodic Systems On Quantum Computing Devices
JOURNAL OF CHEMICAL INFORMATION AND MODELING(2021)
摘要
Quantum machine learning algorithms, the extensions of machine learning to quantum regimes, are believed to be more powerful as they leverage the power of quantum properties. Quantum machine learning methods have been employed to solve quantum many-body systems and have demonstrated accurate electronic structure calculations of lattice models, molecular systems, and recently periodic systems. A hybrid approach using restricted Boltzmann machines and a quantum algorithm to obtain the probability distribution that can be optimized classically is a promising method due to its efficiency and ease of implementation. Here, we implement the benchmark test of the hybrid quantum machine learning on the IBM-Q quantum computer to calculate the electronic structure of typical two-dimensional crystal structures: hexagonal-boron nitride and graphene. The band structures of these systems calculated using the hybrid quantum machine learning approach are in good agreement with those obtained by the conventional electronic structure calculations. This benchmark result implies that the hybrid quantum machine learning method, empowered by quantum computers, could provide a new way of calculating the electronic structures of quantum many-body systems.
更多查看译文
关键词
quantum machine learning,quantum computing devices,electronic structure
AI 理解论文
溯源树
样例
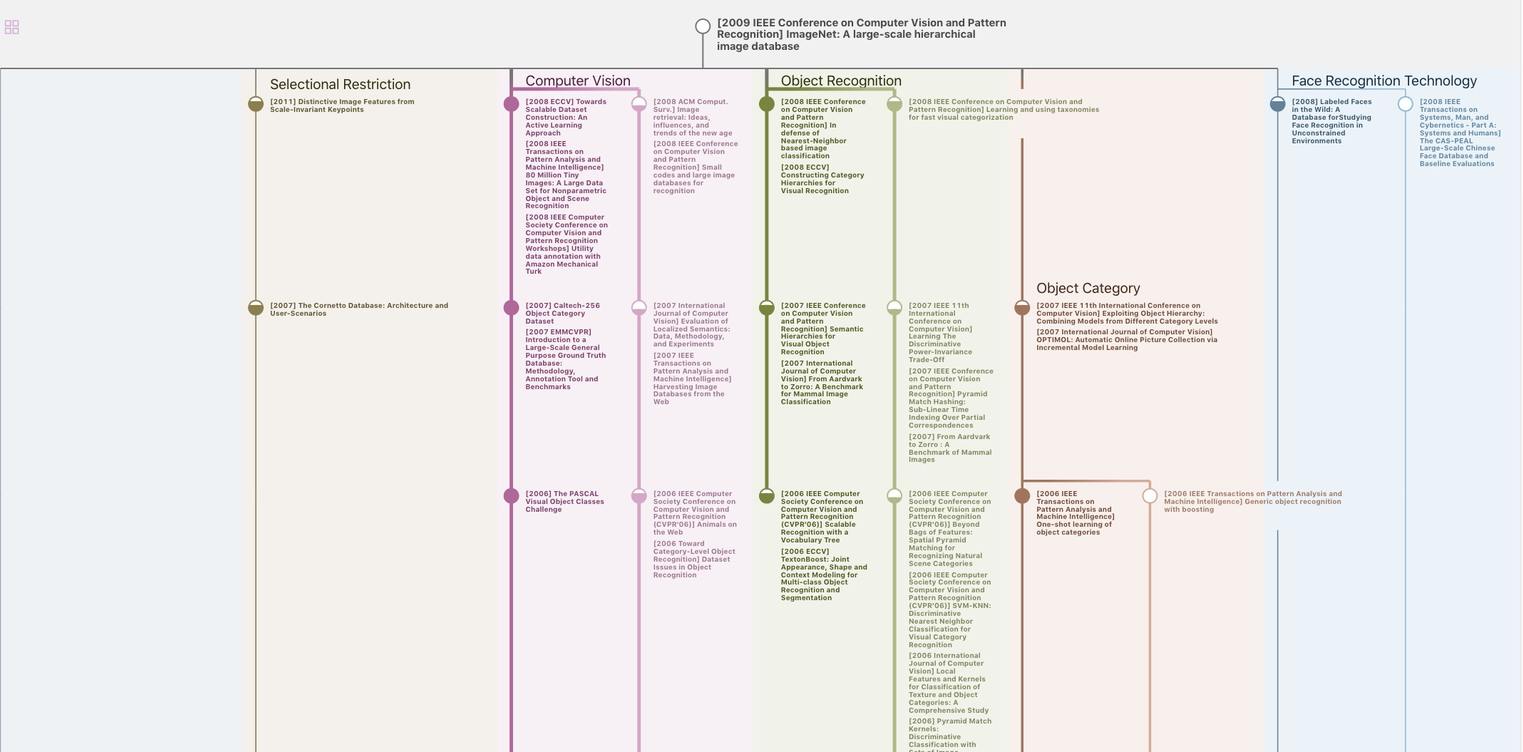
生成溯源树,研究论文发展脉络
Chat Paper
正在生成论文摘要