Transform Network Architectures for Deep Learning Based End-to-End Image/Video Coding in Subsampled Color Spaces
IEEE Open Journal of Signal Processing(2021)
摘要
Most of the existing deep learning based end-to-end image/video coding (DLEC) architectures are designed for non-subsampled RGB color format. However, in order to achieve a superior coding performance, many state-of-the-art block-based compression standards such as High Efficiency Video Coding (HEVC/H.265) and Versatile Video Coding (VVC/H.266) are designed primarily for YUV 4:2:0 format, where U and V components are subsampled by considering the human visual system. This paper investigates various DLEC designs to support YUV 4:2:0 format by comparing their performance against the main profiles of HEVC and VVC standards under a common evaluation framework. Moreover, a new transform network architecture is proposed to improve the efficiency of coding YUV 4:2:0 data. The experimental results on YUV 4:2:0 datasets show that the proposed architecture significantly outperforms naive extensions of existing architectures designed for RGB format and achieves about 10% average BD-rate improvement over the intra-frame coding in HEVC.
更多查看译文
关键词
Deep learning,neural networks,transform network,data compression,image coding,video coding,color spaces,YUV,RGB
AI 理解论文
溯源树
样例
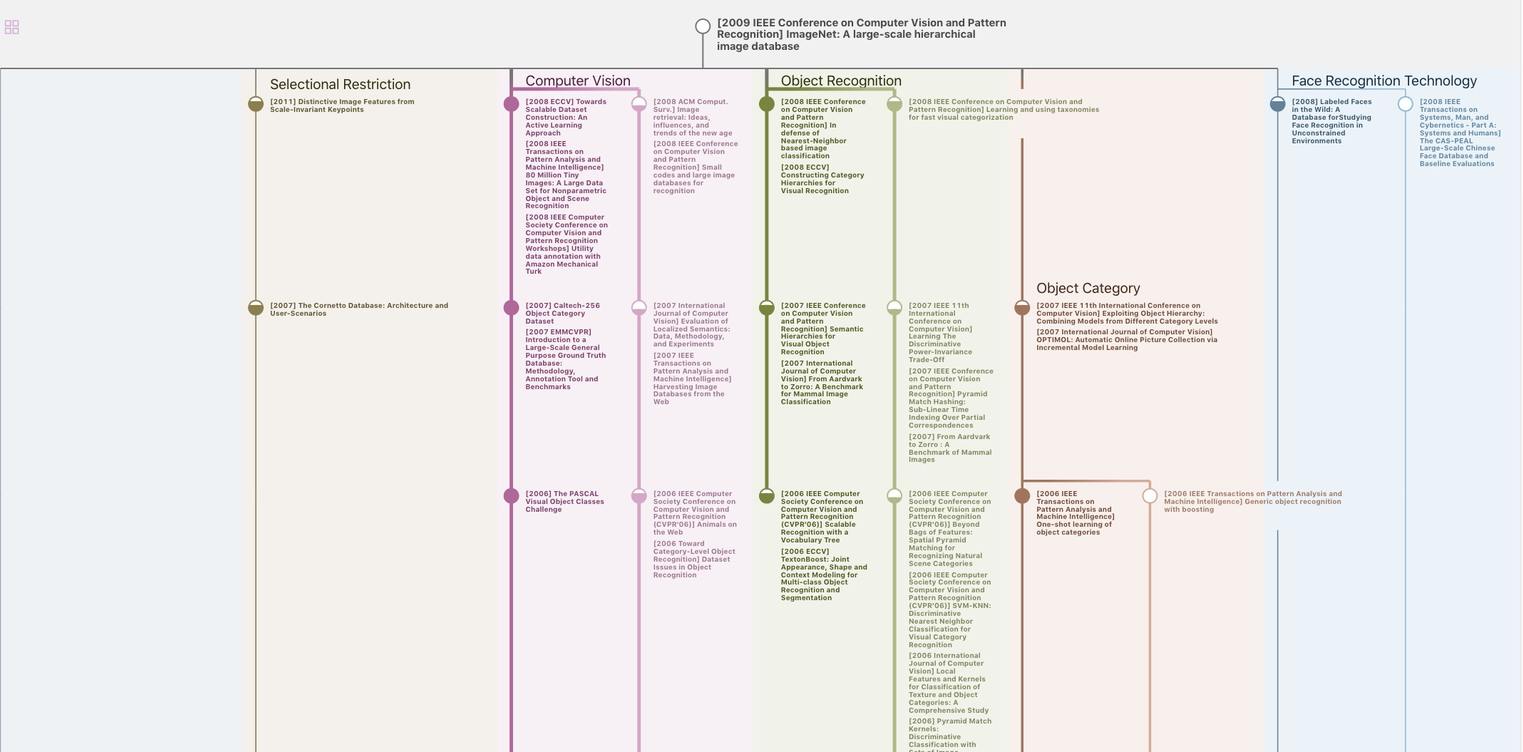
生成溯源树,研究论文发展脉络
Chat Paper
正在生成论文摘要