Machine Learning and Accelerated Stress Approaches to Differentiate Potential Causes of Aggregation in Polyclonal Antibody Formulations During Shipping
Journal of Pharmaceutical Sciences(2021)
摘要
Therapeutic proteins are among the most widely prescribed medications, with wide distribution and complex supply chains. Shipping exposes protein formulations to stresses that can trigger aggregation, although the exact mechanism(s) responsible for aggregation are unknown. To better understand how shipping causes aggregation, we compared populations of aggregates that were formed in a polyclonal antibody formulation during live shipping studies to populations observed in accelerated stability studies designed to mimic both the sporadic high g-force and continuous low g-force stresses encountered during shipping. Additionally, we compared the effects on aggregation levels generated in two types of secondary packaging, one of which was designed to mitigate the effects of large g-force stresses. Aggregation was quantified using fluorescence intensity of 4,4′-dianilino-1,1′-binaphthyl-5,5′-disulfonic acid (bis-ANS) dye, size exclusion high performance liquid chromatography (SECHPLC), and flow imaging microscopy (FIM). FIM was also combined with machine learning methods to analyze particle morphology distributions. These comparisons revealed that the morphology distributions of aggregates formed during live shipping resemble distributions that result from low g-force events, but not those observed following high g-force events, suggesting that low g-force stresses play a predominant role in shipping-induced aggregation.
更多查看译文
关键词
Protein aggregation,Machine learning,Protein formulation,Antibodies
AI 理解论文
溯源树
样例
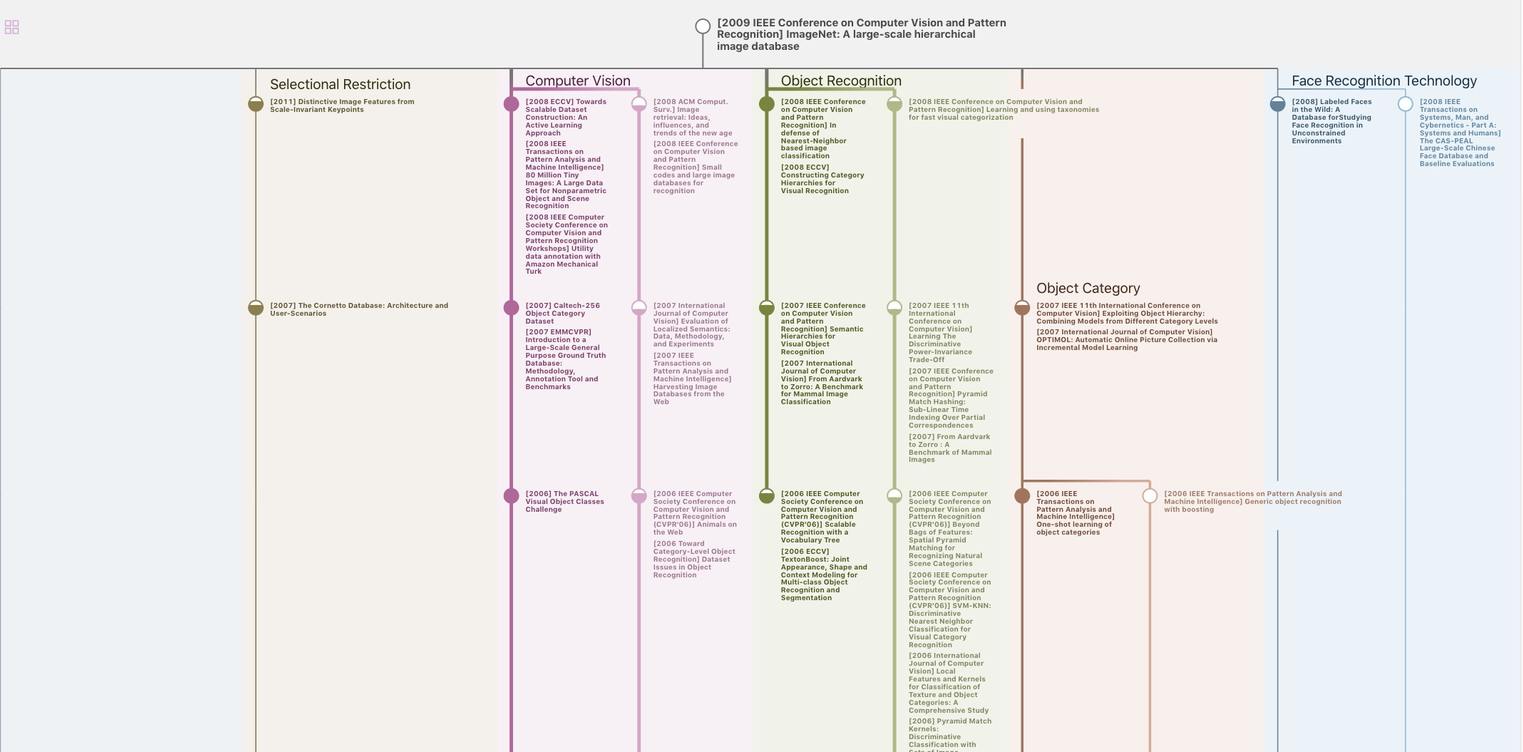
生成溯源树,研究论文发展脉络
Chat Paper
正在生成论文摘要