Learning Multimodal Contact-Rich Skills from Demonstrations Without Reward Engineering
2021 IEEE INTERNATIONAL CONFERENCE ON ROBOTICS AND AUTOMATION (ICRA 2021)(2021)
摘要
Everyday contact-rich tasks, such as peeling, cleaning, and writing, demand multimodal perception for effective and precise task execution. However, these present a novel challenge to robots as they lack the ability to combine these multimodal stimuli for performing contact-rich tasks. Learning-based methods have attempted to model multi-modal contact-rich tasks, but they often require extensive training examples and task-specific reward functions which limits their practicality and scope. Hence, we propose a generalizable model-free learning-from-demonstration framework for robots to learn contact-rich skills without explicit reward engineering. We present a novel multi-modal sensor data representation which improves the learning performance for contact-rich skills. We performed training and experiments using the real-life Sawyer robot for three everyday contact-rich skills – cleaning, writing, and peeling. Notably, the framework achieves a success rate of 100% for the peeling and writing skill, and 80% for the cleaning skill. Hence, this skill learning framework can be extended for learning other physical manipulation skills.
更多查看译文
关键词
demonstrations,skills,reward engineering,learning,contact-rich
AI 理解论文
溯源树
样例
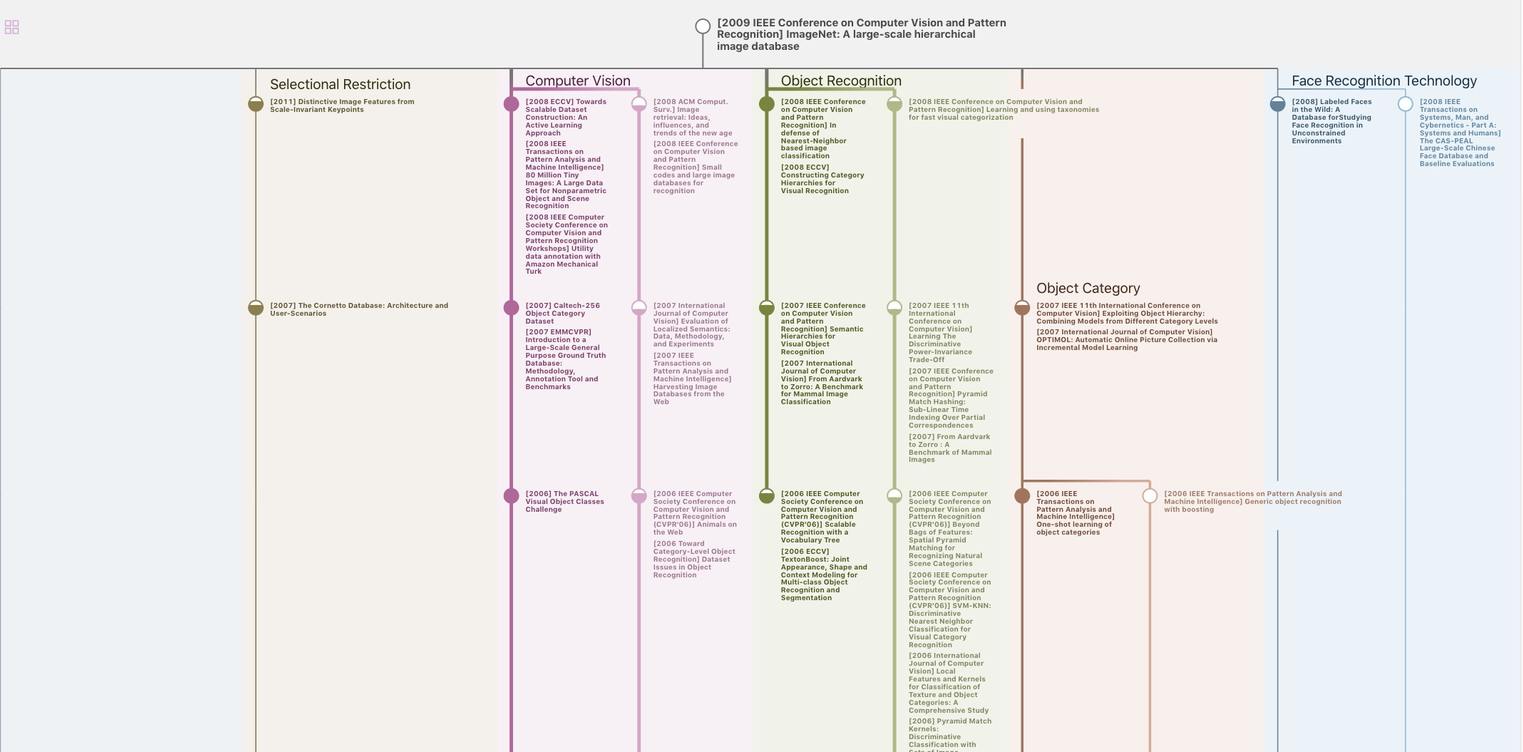
生成溯源树,研究论文发展脉络
Chat Paper
正在生成论文摘要