Automated Data-Driven Approach for Gap Filling in the Time Series Using Evolutionary Learning
16TH INTERNATIONAL CONFERENCE ON SOFT COMPUTING MODELS IN INDUSTRIAL AND ENVIRONMENTAL APPLICATIONS (SOCO 2021)(2022)
摘要
In the paper, we propose an adaptive data-driven model-based approach for filling the gaps in time series. The approach is based on the automated evolutionary identification of the optimal structure for a composite data-driven model. It allows adapting the model for the effective gap-filling in a specific dataset without the involvement of the data scientist. As a case study, both synthetic and real datasets from different fields (environmental, economic, etc.) are used. The experiments confirm that the proposed approach allows achieving the higher quality of the gap restoration and improve the effectiveness of forecasting models.
更多查看译文
关键词
Time series forecasting, Gap filling, Machine learning, AutoML
AI 理解论文
溯源树
样例
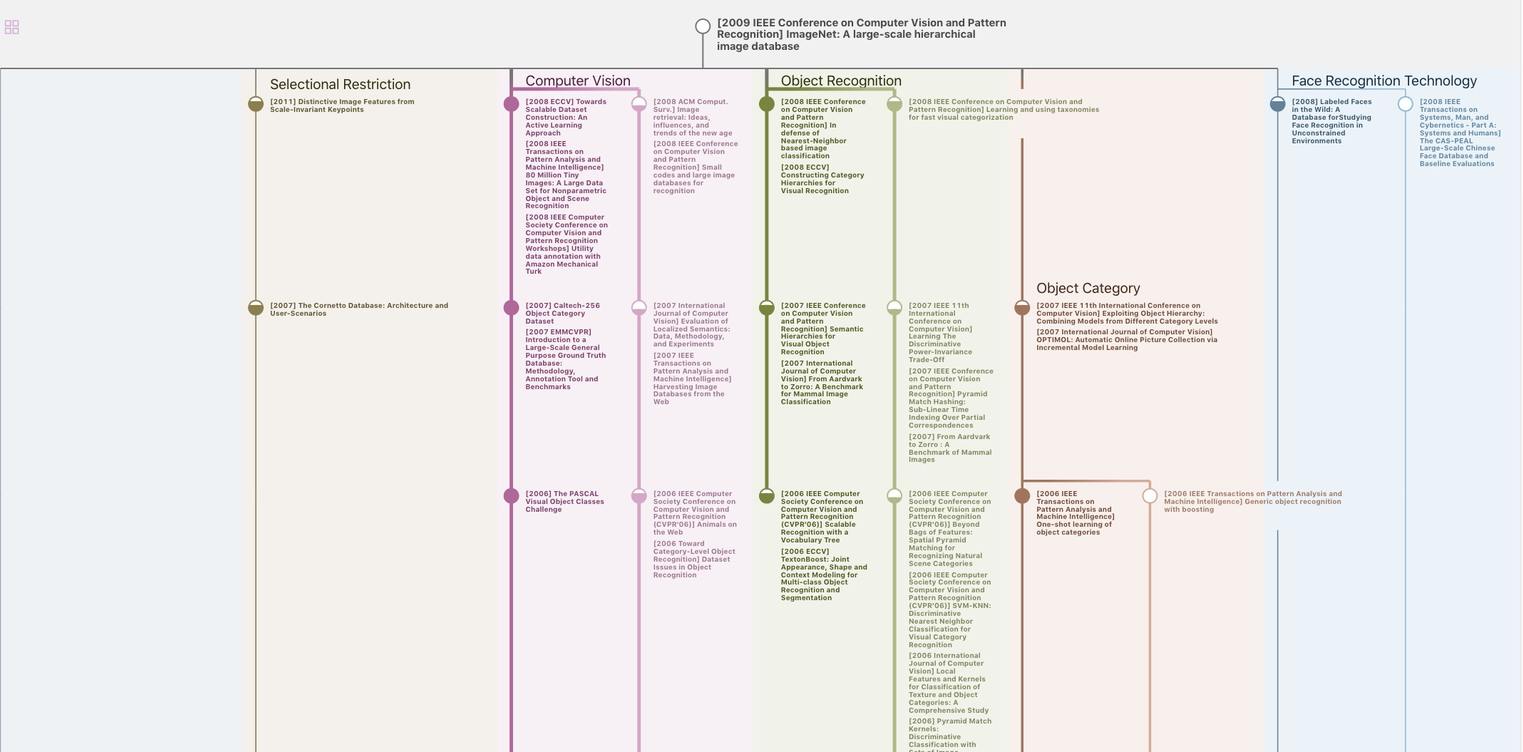
生成溯源树,研究论文发展脉络
Chat Paper
正在生成论文摘要