Tax evasion risk management using a Hybrid Unsupervised Outlier Detection method
Expert Systems with Applications(2022)
Abstract
Big data methods are becoming an important tool for tax fraud detection around the world. Unsupervised learning approach is the dominant framework due to the lack of label and ground truth in corresponding datasets, although these methods suffer from lower interpretability and precision compared with supervised approaches. In contrast to prior research works, we examine the possibility of a hybrid unsupervised method for tax evasion risk management that is able to internally validate and explain outliers detected in a given tax dataset. The proposed method, HUNOD (Hybrid UNsupervised Outlier Detection),11HUNOD is an abbreviation for “Hybrid UNsupervised Outlier Dection”. combines clustering and representation learning for robust outlier detection, additionally allowing its users to incorporate relevant domain knowledge into both constituent outlier detection approaches in order to identify outliers relevant for a given economic context. The interpretability of obtained outliers is achieved by training explainable-by-design surrogate models over internally validated outliers. The experimental evaluation of the HUNOD method is conducted on two datasets derived from the database on individual personal income tax declarations collected by the Tax Administration of Serbia. The obtained results show that the method indicates between 90% and 98% internally validated outliers depending on the clustering configuration and employed regularization mechanisms for representational learning.
MoreTranslated text
Key words
Tax evasion,Outlier detection,Unsupervised learning,Clustering,Representational learning,Explainable surrogate models
AI Read Science
Must-Reading Tree
Example
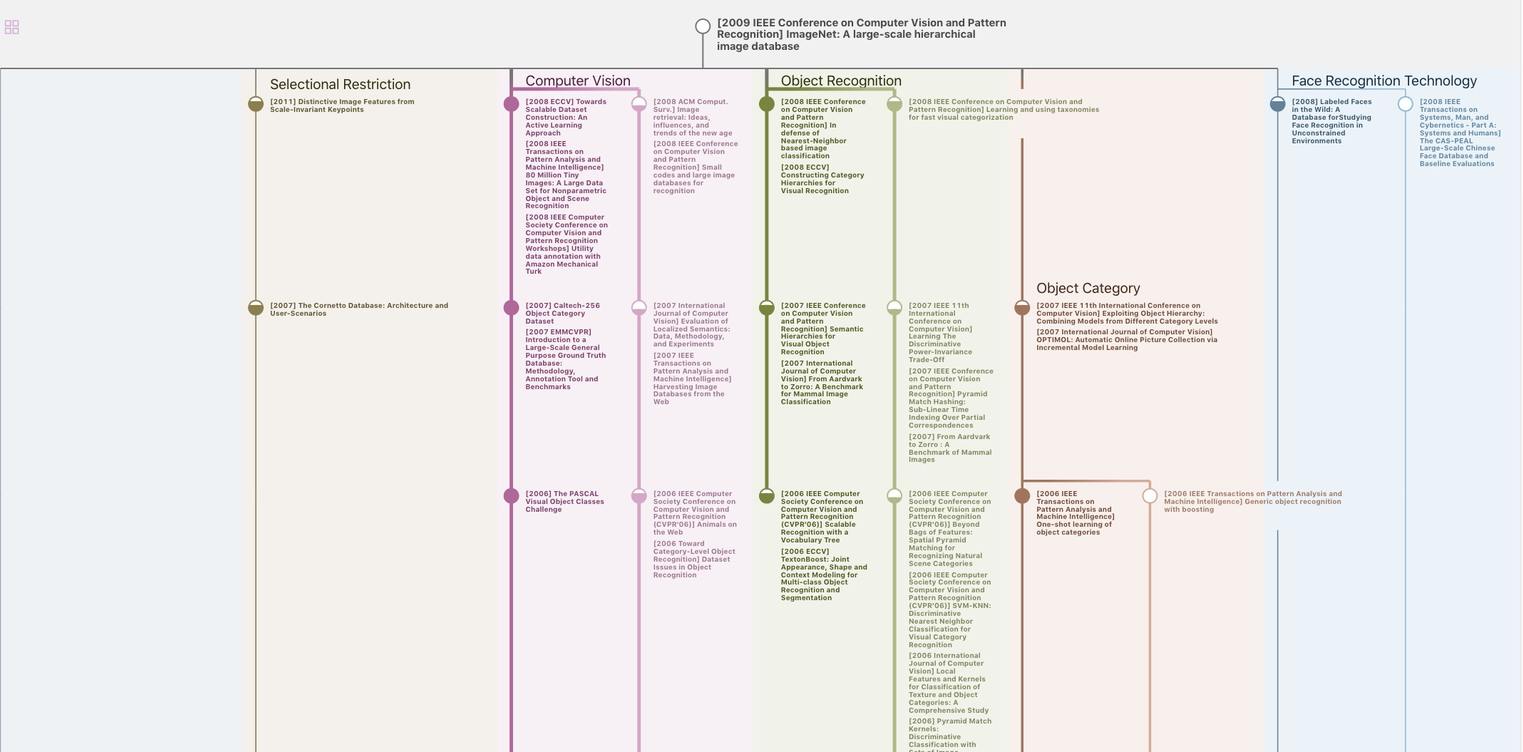
Generate MRT to find the research sequence of this paper
Chat Paper
Summary is being generated by the instructions you defined