A simple method for improving the accuracy of Chung-Lu random graph generation
arxiv(2021)
摘要
Random graph models play a central role in network analysis. The Chung-Lu model, which connects nodes based on their expected degrees is of particular interest. It is widely used to generate null-graph models with expected degree sequences as well as implicitly define network measures such as modularity. Despite its popularity, practical methods for generating instances of Chung-Lu model-based graphs do relatively poor jobs in terms of accurately realizing many degree sequences. We introduce a simple method for improving the accuracy of Chung-Lu graph generation. Our method uses a Poisson approximation to define a linear system describing the expected degree sequence to be output from the model using standard generation techniques. We then use the inverse of this system to determine an appropriate input corresponding to the desired output. We give a closed form expression for this inverse and show that it may be used to drastically reduce error for many degree distributions.
更多查看译文
AI 理解论文
溯源树
样例
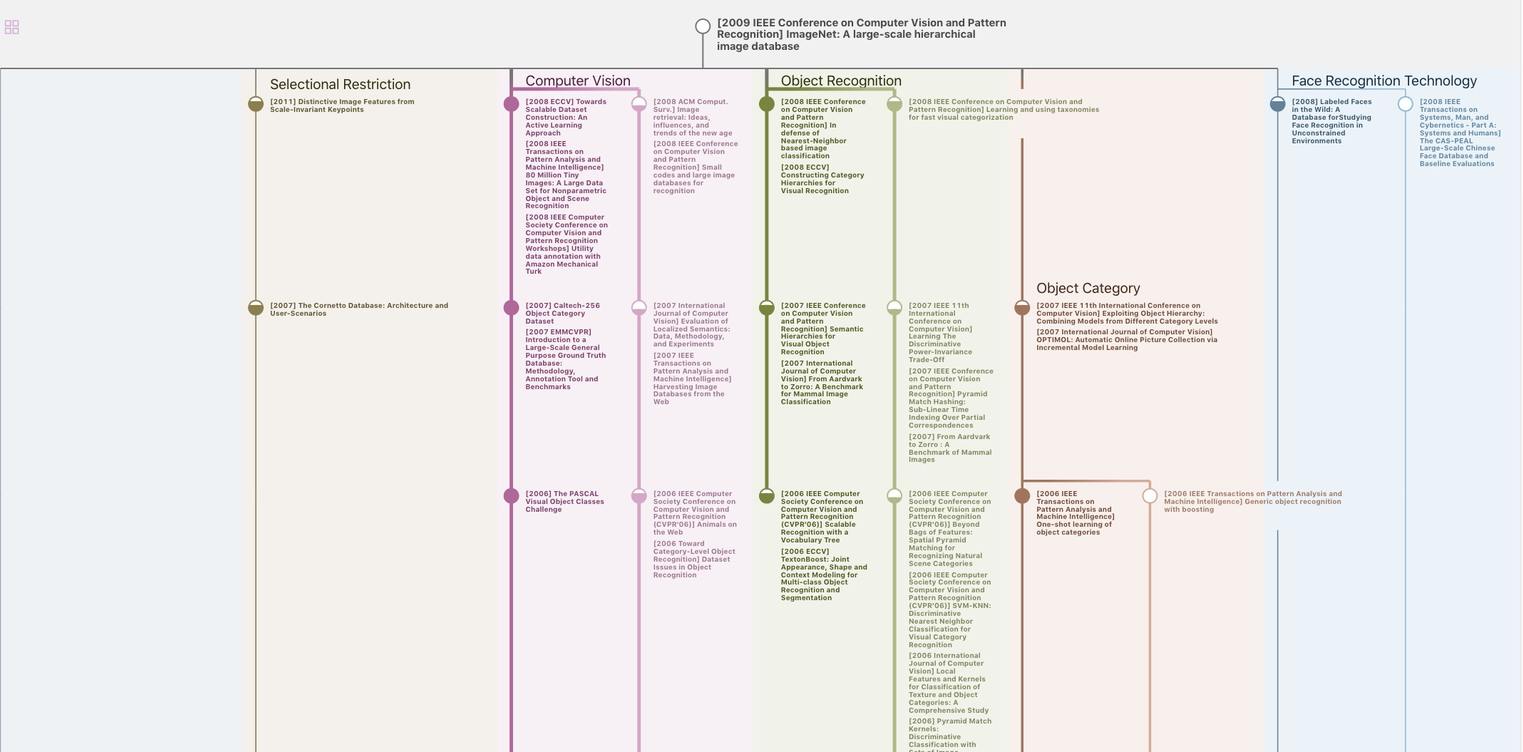
生成溯源树,研究论文发展脉络
Chat Paper
正在生成论文摘要