Ensemble Bootstrapping for Q-Learning
INTERNATIONAL CONFERENCE ON MACHINE LEARNING, VOL 139(2021)
Abstract
Q-learning (QL), a common reinforcement learning algorithm, suffers from over-estimation bias due to the maximization term in the optimal Bellman operator. This bias may lead to suboptimal behavior. Double-Q-learning tackles this issue by utilizing two estimators, yet results in an under-estimation bias. Similar to over-estimation in Q-learning, in certain scenarios, the under-estimation bias may degrade performance. In this work, we introduce a new bias-reduced algorithm called Ensemble Bootstrapped Q-Learning (EBQL), a natural extension of Double-Q-learning to ensembles. We analyze our method both theoretically and empirically. Theoretically, we prove that EBQL-like updates yield lower MSE when estimating the maximal mean of a set of independent random variables. Empirically, we show that there exist domains where both over and under-estimation result in sub-optimal performance. Finally, We demonstrate the superior performance of a deep RL variant of EBQL over other deep QL algorithms for a suite of ATARI games.
MoreTranslated text
Key words
ensemble bootstrapping,q-learning
AI Read Science
Must-Reading Tree
Example
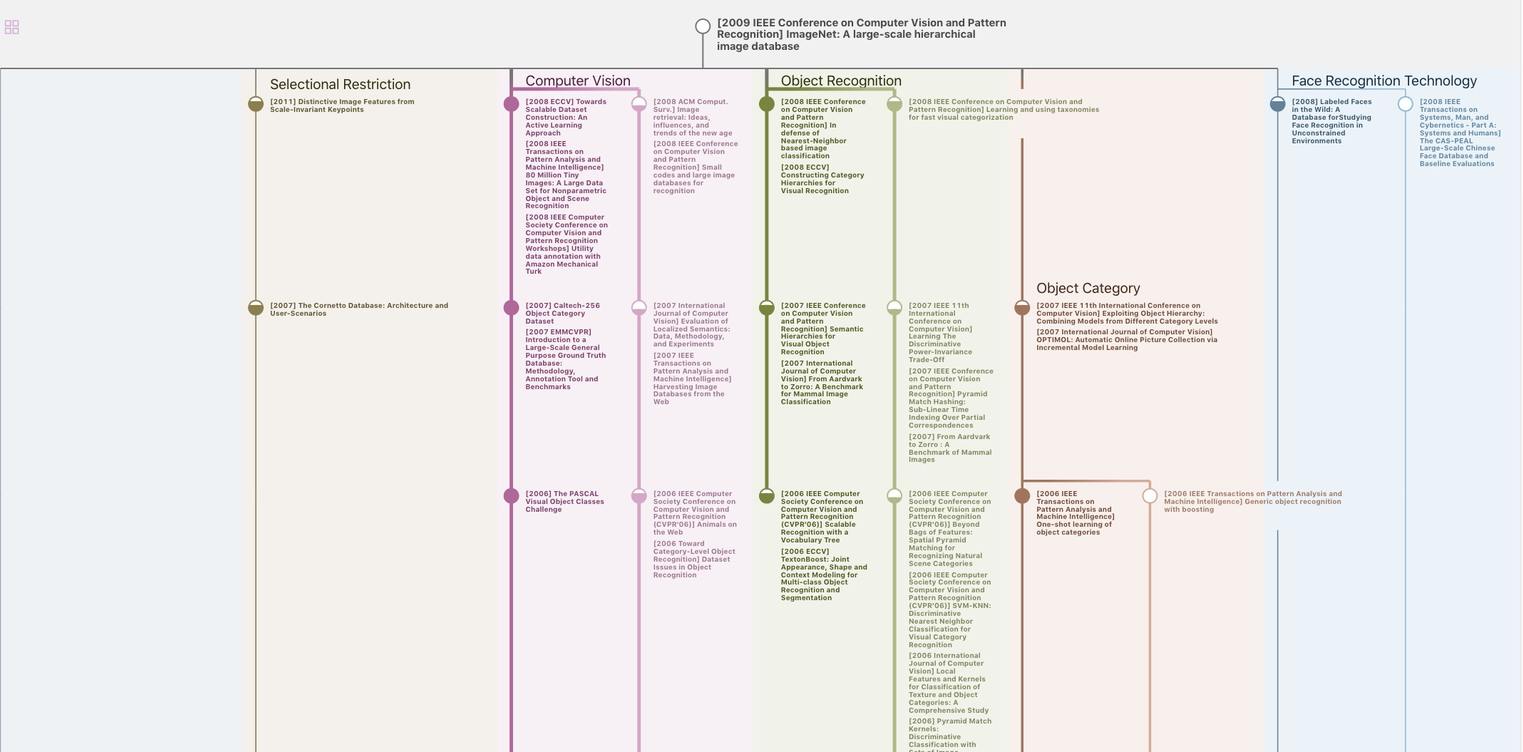
Generate MRT to find the research sequence of this paper
Chat Paper
Summary is being generated by the instructions you defined