A data-driven predictome for cognitive, psychiatric, medical, and lifestyle factors on the brain
user-5f8411ab4c775e9685ff56d3(2020)
摘要
ABSTRACT Most neuroimaging studies individually provide evidence on a narrow aspect of the human brain function, on distinct data sets that often suffer from small sample sizes. More generally, the high technical and cost demands of neuroimaging studies (combined with the statistical unreliability of neuroimaging pilot studies) may lead to observational bias, discouraging discovery of less obvious associations that nonetheless have important neurological implications. To address these problems, we built a machine-learning based classification framework, NeuroPredictome, optimized for the reliability and robustness of its associations. NeuroPredictome is grounded in a large-scale dataset, UK-Biobank (N=19,831), which includes resting and task functional MRI as well as structural T1-weighted and diffusion tensor imaging. Participants were assessed with respect to a comprehensive set of 5,034 phenotypes, including the physical and lifestyle factors most relevant to general medicine. Results generated by data-driven classifiers were then cross-validated, using deep-learning textual analyses, against 14,371 peer-reviewed research articles, providing an unbiased hypothesis-generator of linkages between diverse phenotypes and the brain. Our results show that neuroimaging reveals as many neurological links to physical and lifestyle factors as to cognitive factors, supporting a more integrative approach to medicine that considers disease interactions between multiple organs and systems.
更多查看译文
关键词
Neuroimaging,Cognition,Observational study,Disease,Cognitive psychology,Diffusion MRI,Human brain,Data set,Data-driven,Psychology
AI 理解论文
溯源树
样例
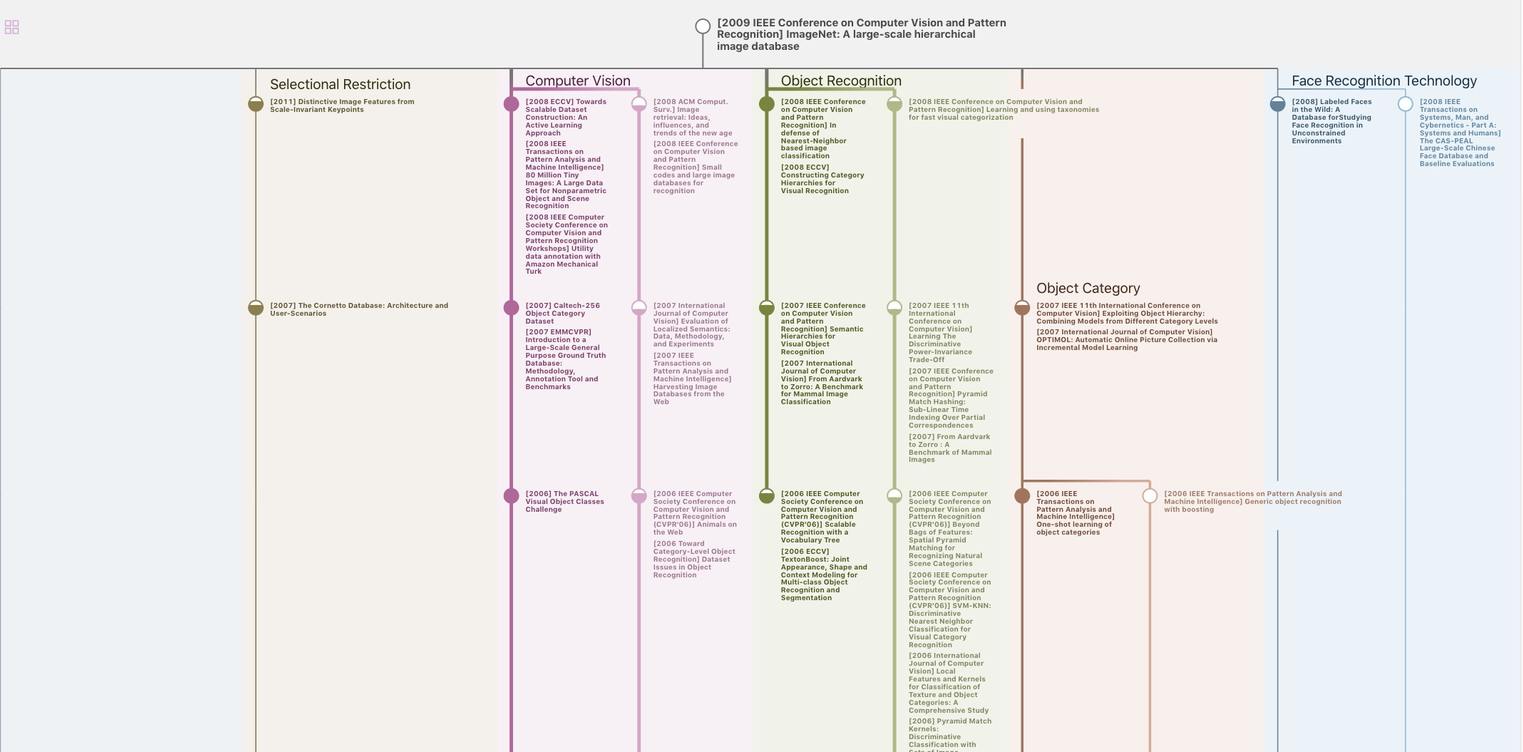
生成溯源树,研究论文发展脉络
Chat Paper
正在生成论文摘要