Deep Reinforcement Learning for Quantum Hamiltonian Engineering
PHYSICAL REVIEW APPLIED(2022)
摘要
Engineering desired Hamiltonians in quantum many-body systems is essential for applications such as quantum simulation, computation, and sensing. Many widely used quantum Hamiltonian engineering sequences are designed using human intuition based on perturbation theory, which may not describe the optimal solution and is unable to accommodate complex experimental imperfections. Here we numerically search for Hamiltonian engineering sequences using deep reinforcement learning (DRL) techniques and experimentally demonstrate that they outperform celebrated decoupling sequences on a solid-state nuclear magnetic resonance quantum simulator. As an example, we aim at decoupling strongly interacting spin-1/2 systems. We train DRL agents in the presence of different experimental imperfections and verify robustness of the output sequences both in simulations and experiments. Surprisingly, many of the learned sequences exhibit a common pattern that had not been discovered before, to our knowledge, but has a meaningful analytical description. We can thus restrict the searching space based on this control pattern, allowing us to search for longer sequences, ultimately leading to sequences that are robust against dominant imperfections in our experiments. Our results not only demonstrate a general method for quantum Hamiltonian engineering, but also highlight the importance of combining black-box artificial intelligence with an understanding of the physical system in order to realize experimentally feasible applications.
更多查看译文
关键词
reinforcement learning,quantum,hamiltonian
AI 理解论文
溯源树
样例
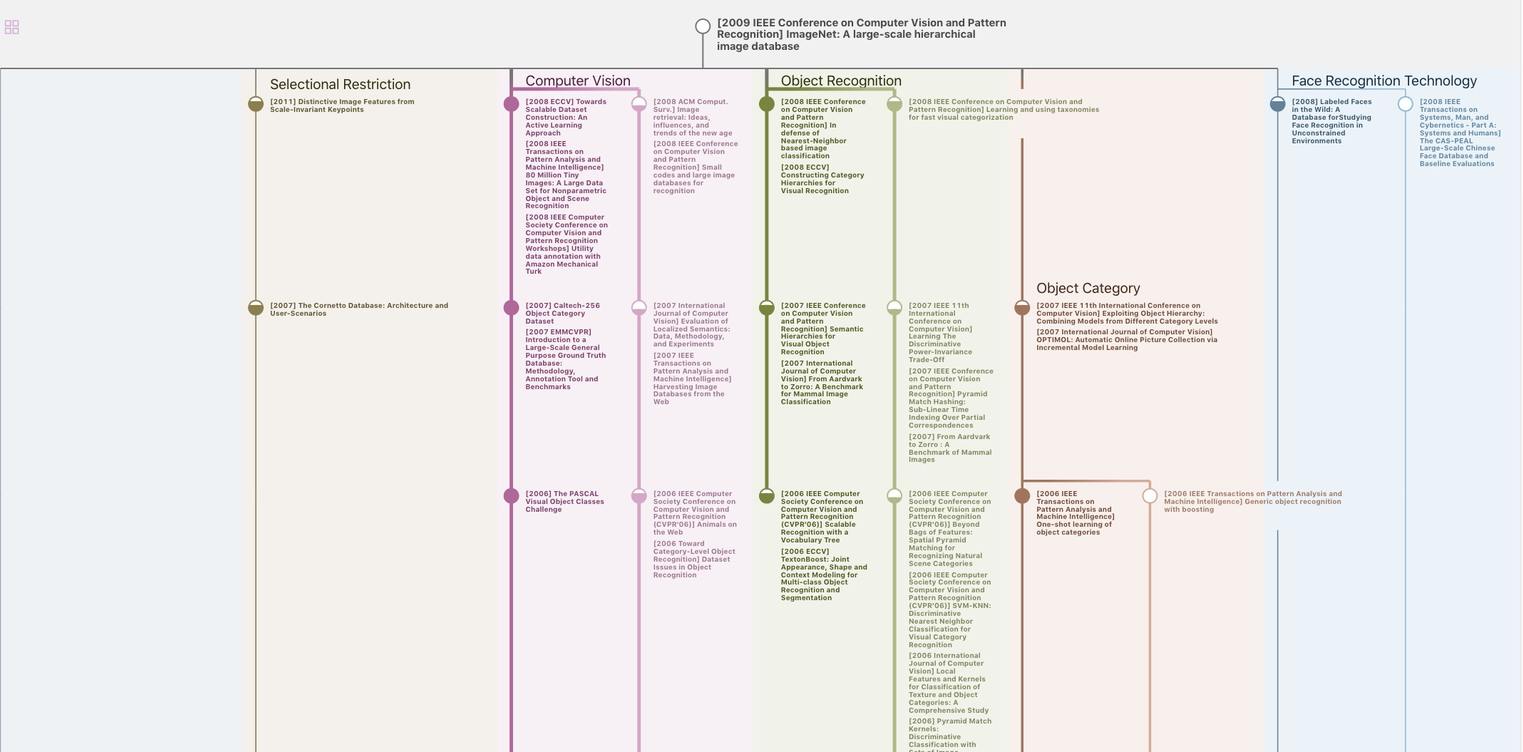
生成溯源树,研究论文发展脉络
Chat Paper
正在生成论文摘要