Virtual Network Function Embedding under Nodal Outage using Reinforcement Learning
2020 IEEE INTERNATIONAL CONFERENCE ON ADVANCED NETWORKS AND TELECOMMUNICATIONS SYSTEMS (IEEE ANTS)(2020)
Abstract
With the emergence of various types of applications such as delay-sensitive applications, future communication networks are expected to be increasingly complex and dynamic. Network Function Virtualization (NFV) provides the necessary support towards efficient management of such complex networks, by disintegrating the dependency on the hardware devices via virtualizing the network functions and placing them on shared data centres. However, one of the main challenges of the NFV paradigm is the resource allocation problem which is known as NFV-Resource Allocation (NFV-RA). NFV-RA is a method of deploying software-based network functions on the substrate nodes, subject to the constraints imposed by the underlying infrastructure and the agreed Service Level Agreement (SLA). This work investigates the potential of Reinforcement Learning (RL) as a fast yet accurate means (as compared to integer linear programming) for deploying the softwarized network functions onto substrate networks under several Quality of Service (QoS) constraints. In addition to the regular resource constraints and latency constraints, we introduced the concept of a complete outage of certain nodes in the network. This outage can be either due to a disaster or unavailability of network topology information due to proprietary and ownership issues. We have analyzed the network performance on different network topologies, different capacities of the nodes and the links, and different degrees of the nodal outage. The computational time escalated with the increase in the network density to achieve the optimal solutions; this is because Q-Learning is an iterative process which results in a slow exploration. Our results also show that for certain topologies and a certain combination of resources, we can achieve between 70-90% service acceptance rate even with a 40% nodal outage.
MoreTranslated text
Key words
network performance,network density,Q-Learning,40% nodal outage,network topology information,complete outage,latency constraints,regular resource constraints,Service constraints,substrate networks,softwarized network,fast yet accurate means,agreed Service Level Agreement,substrate nodes,software-based network functions,NFV-RA,NFV-Resource Allocation,resource allocation problem,NFV paradigm,shared data centres,hardware devices,complex networks,necessary support,Network Function Virtualization,future communication networks,delay-sensitive applications,Reinforcement Learning,virtual network Function embedding
AI Read Science
Must-Reading Tree
Example
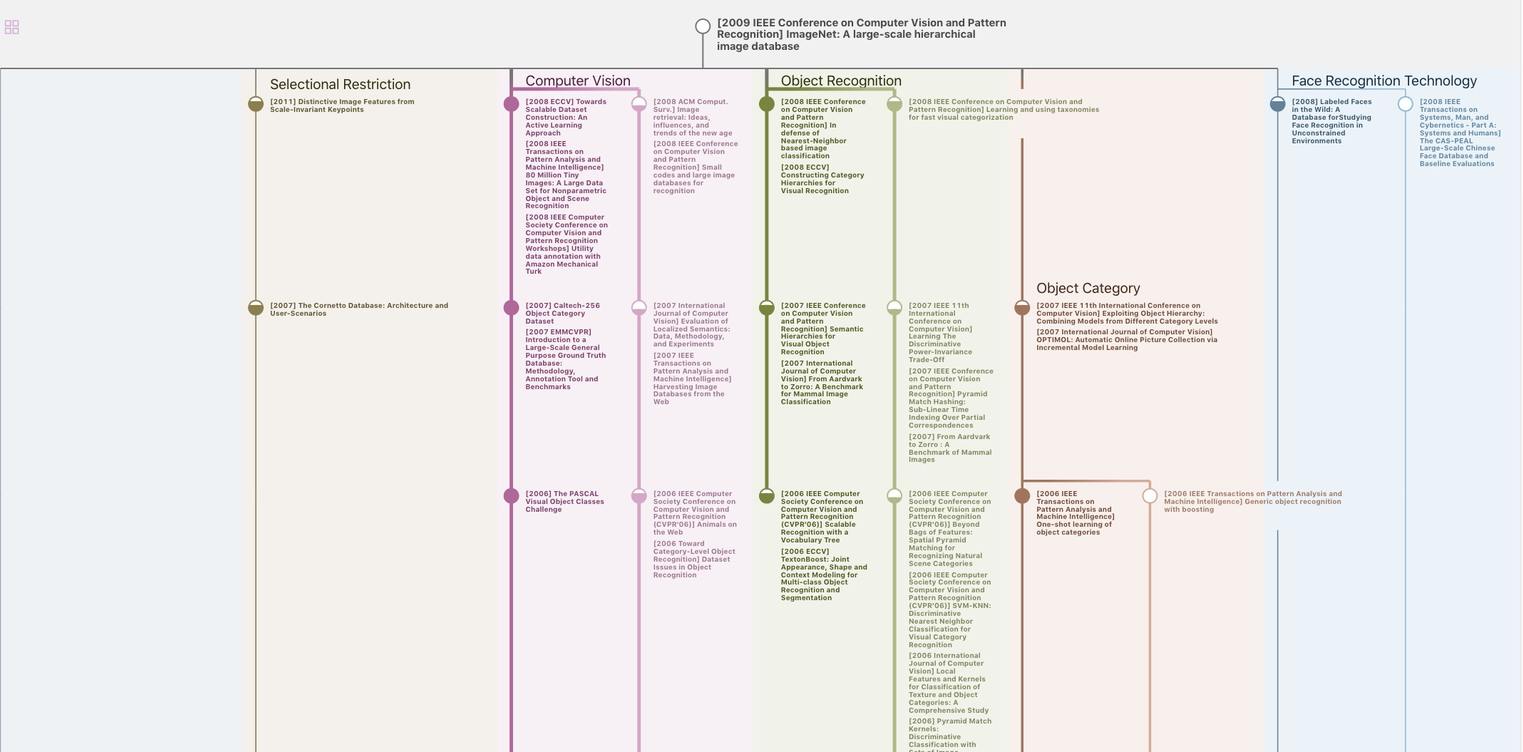
Generate MRT to find the research sequence of this paper
Chat Paper
Summary is being generated by the instructions you defined