A Hybrid Model For Financialtime-Seriesforecasting Based On Mixed Methodologies
EXPERT SYSTEMS(2021)
Abstract
This paper proposes a hybrid model that combines ensemble empirical mode decomposition (EEMD), autoregressive integrated moving average (ARIMA), and Taylor expansion using a tracking differentiator to forecast financial time series. Specifically, the financial time series is decomposed by EEMD into some subseries. Then, the linear portion of each subseries is forecasted by the linear ARIMA model, while the nonlinear portion is predicted by the nonlinear Taylor expansion model. The forecasting results of the linear and nonlinear models are combined into the predicted result of each subseries. The final prediction result is obtained by combining the prediction values of all the subseries. The empirical results with real financial time series data demonstrate that this new hybrid approach outperforms the benchmark hybrid models considered in this paper.
MoreTranslated text
Key words
ARIMA, EEMD, financial time series, forecasting, Taylor expansion
AI Read Science
Must-Reading Tree
Example
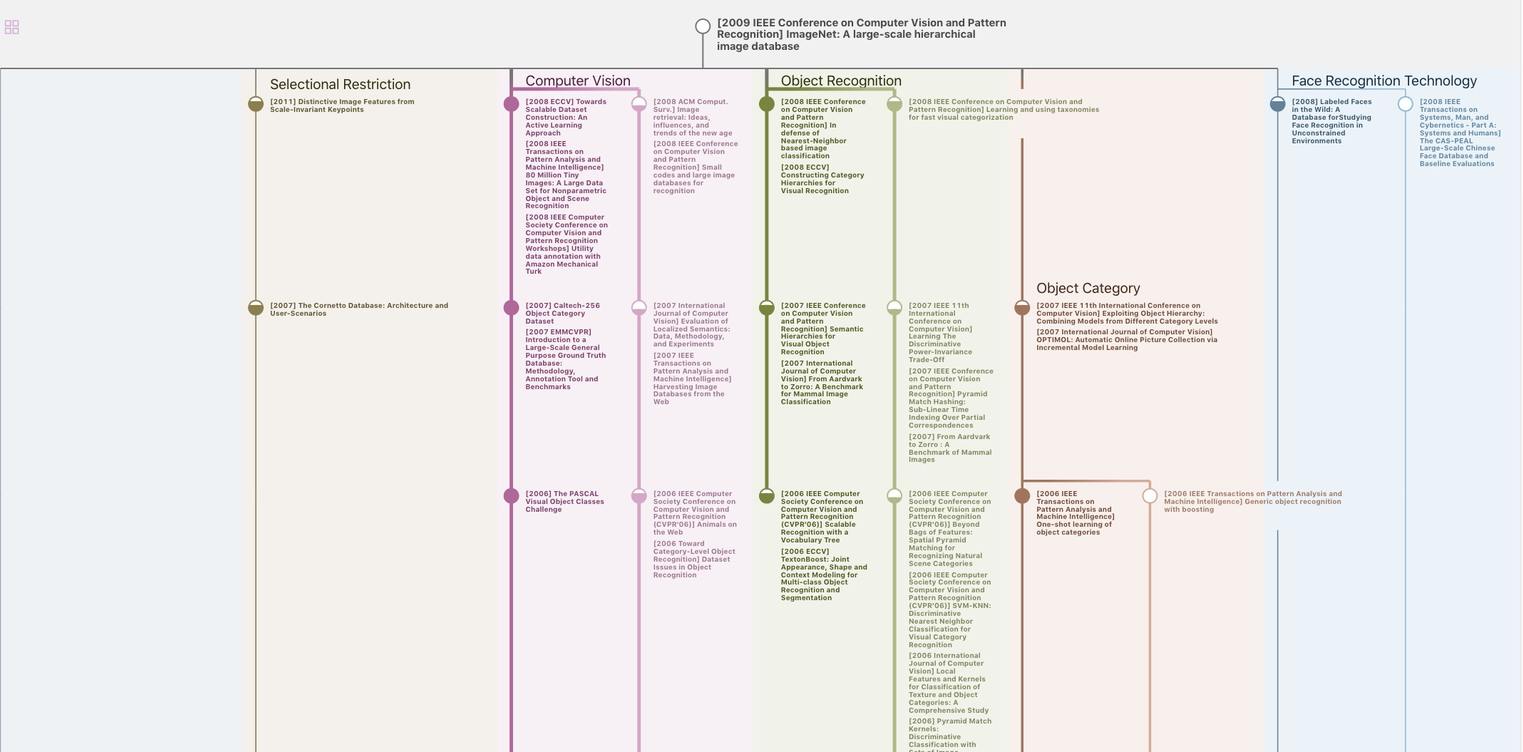
Generate MRT to find the research sequence of this paper
Chat Paper
Summary is being generated by the instructions you defined