Machine Learning Methods' Performance In Radiative Transfer Model Inversion To Retrieve Plant Traits From Sentinel-2 Data Of A Mixed Mountain Forest
INTERNATIONAL JOURNAL OF DIGITAL EARTH(2021)
摘要
Assessment of vegetation biochemical and biophysical variables is useful when developing indicators for biodiversity monitoring and climate change studies. Here, we compared a radiative transfer model (RTM) inversion by merit function and five machine learning algorithms trained on an RTM simulated dataset predicting the three plant traits leaf chlorophyll content (LCC), canopy chlorophyll content (CCC), and leaf area index (LAI), in a mixed temperate forest. The accuracy of the retrieval methods in predicting these three plant traits with spectral data from Sentinel-2 acquired on 13 July 2017 over Bavarian Forest National Park, Germany, was evaluated usingin situmeasurements collected contemporaneously. The RTM inversion using merit function resulted in estimations of LCC (R-2 = 0.26, RMSE = 3.9 mu g/cm(2)), CCC (R-2 = 0.65, RMSE = 0.33 g/m(2)), and LAI (R-2 = 0.47, RMSE = 0.73 m(2)/m(2)), comparable to the estimations based on the machine learning method Random forest regression of LCC (R-2 = 0.34, RMSE = 4.06 mu g/cm(2)), CCC (R-2 = 0.65, RMSE = 0.34 g/m(2)), and LAI (R-2 = 0.47, RMSE = 0.75 m(2)/m(2)). Several of the machine learning algorithms also yielded accuracies and robustness similar to the RTM inversion using merit function. The performance of regression methods trained on synthetic datasets showed promise for fast and accurate mapping of plant traits accross different plant functional types from remote sensing data.
更多查看译文
关键词
Leaf area index, leaf, canopy chlorophyll content, radiative transfer model, look-up table, machine learning algorithms
AI 理解论文
溯源树
样例
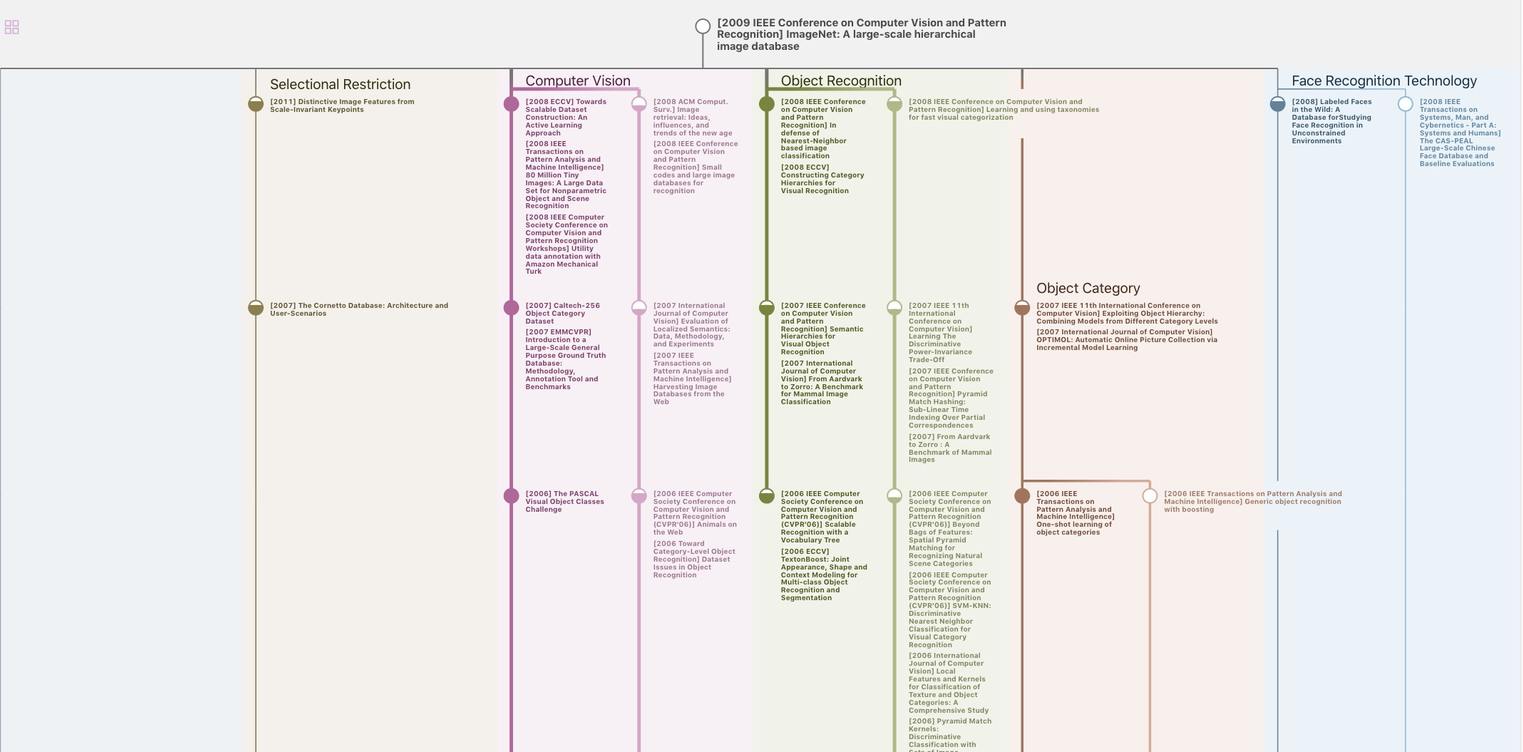
生成溯源树,研究论文发展脉络
Chat Paper
正在生成论文摘要