Optimal Planning Over Long And Infinite Horizons For Achieving Independent Partially-Observable Tasks That Evolve Over Time
arXiv (Cornell University)(2021)
摘要
We focus on long-sighted planning for a class of problems with multiple independent tasks that are partially observable and evolve over time. An example problem that falls into this class is a robot waiting multiple tables, referred to as tasks, in a restaurant where customers' satisfaction is partially observable and evolves over time. Our recent work exploits the structure found in these problems, namely the independence between the tasks, to optimally and efficiently plan for a short fixed planning horizon. Selecting the right planning horizon can be challenging since an overly short horizon may result in a low-quality solution while supporting a longer horizon quickly becomes computationally impractical. In this paper, we address this challenge. In particular, we extend the recent work to provide efficient planning over long fixed-length horizons without discounting and infinite-length horizons with discounting. The key idea we exploit to achieve efficiency is to compute lower and upper-bounds on the value of an optimal solution for variable horizons which allow us to terminate the search early while guaranteeing optimality. We present the algorithm, analyze its theoretical properties, and demonstrate its efficiency on the waiting tables domain.
更多查看译文
关键词
AI-enabled robotics, task planning, planning under uncertainty, POMDP planning
AI 理解论文
溯源树
样例
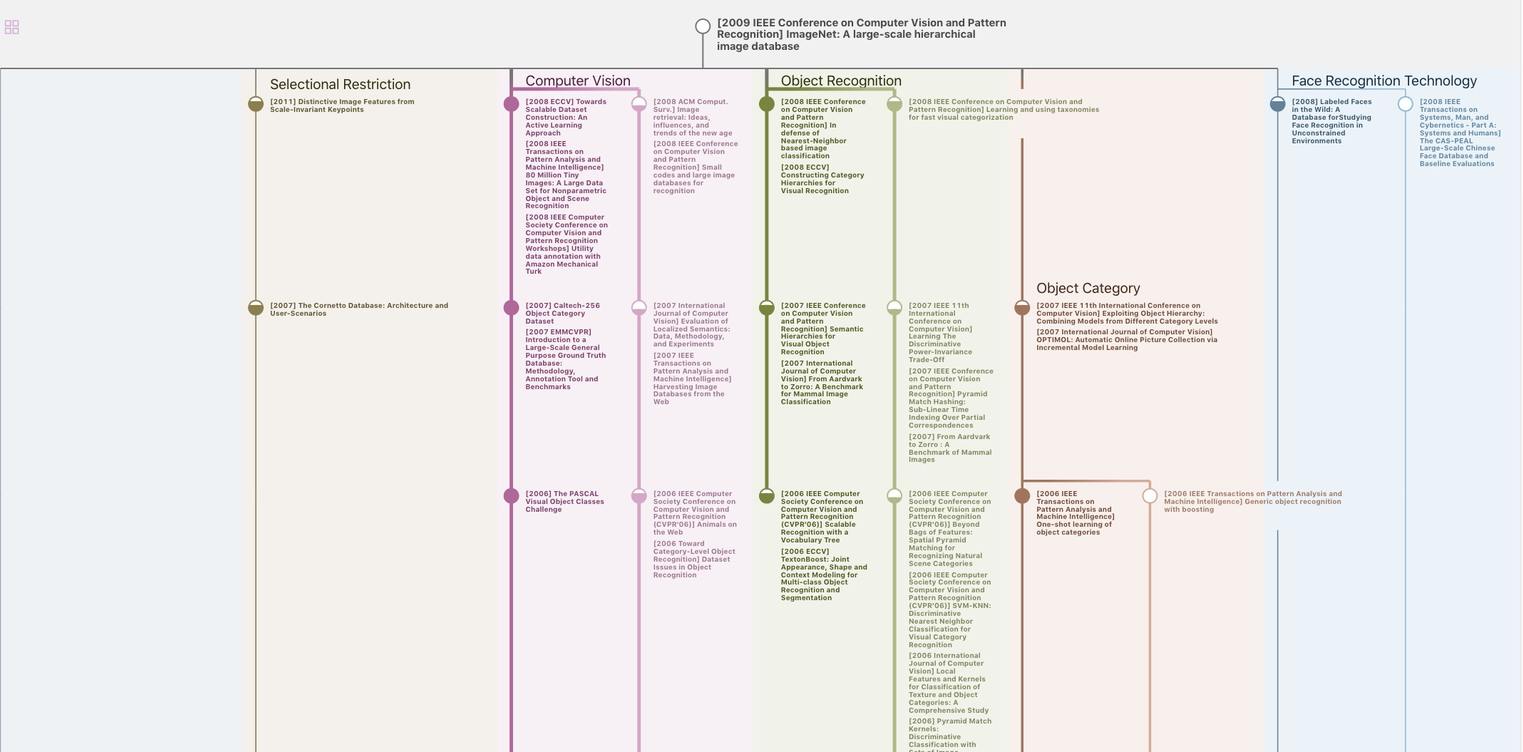
生成溯源树,研究论文发展脉络
Chat Paper
正在生成论文摘要