An FPGA Implementation of Convolutional Spiking Neural Networks for Radioisotope Identification
2021 IEEE INTERNATIONAL SYMPOSIUM ON CIRCUITS AND SYSTEMS (ISCAS)(2021)
Abstract
This paper details FPGA implementation methodology for Convolutional Spiking Neural Networks (CSNN) and applies this methodology to low-power radioisotope identification using high resolution data. A power consumption of 75 mW has been achieved on an FPGA implementation of a CSNN, with the inference accuracy of 90.62% on a synthetic dataset. The chip validation method is presented. Prototyping was accelerated by evaluating SNN parameters using SpiNNaker neuromorphic platform.
MoreTranslated text
Key words
event-based signal processing,low power,radioisotope identification,convolutional spiking neural networks,FPGA,SpiNNaker
AI Read Science
Must-Reading Tree
Example
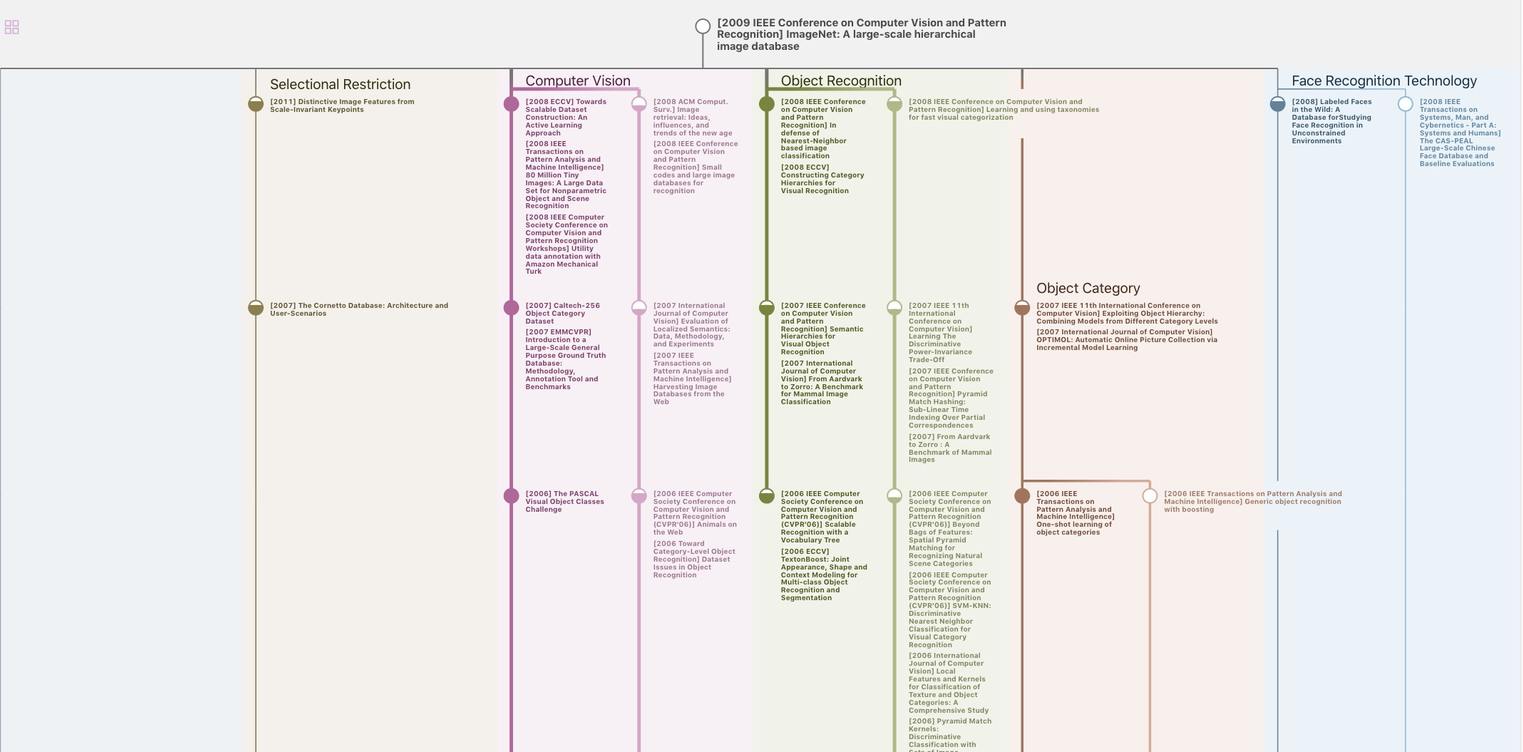
Generate MRT to find the research sequence of this paper
Chat Paper
Summary is being generated by the instructions you defined