When Attention Meets Fast Recurrence - Training Language Models with Reduced Compute.
EMNLP(2021)
Abstract
Large language models have become increasingly difficult to train because of the required computation time and cost. In this work, we present SRU++, a recurrent unit with optional built-in attention that exhibits state-of-the-art modeling capacity and training efficiency. On standard language modeling benchmarks such as enwik8 and Wiki-103 datasets, our model obtains better perplexity and bits-per-character (bpc) while using 2.5x-10x less training time and cost compared to top-performing Transformer models. Our results reaffirm that attention is not all we need and can be complementary to other sequential modeling modules. Moreover, fast recurrence with little attention can be a leading model architecture.
MoreTranslated text
Key words
training language models,fast recurrence,attention
AI Read Science
Must-Reading Tree
Example
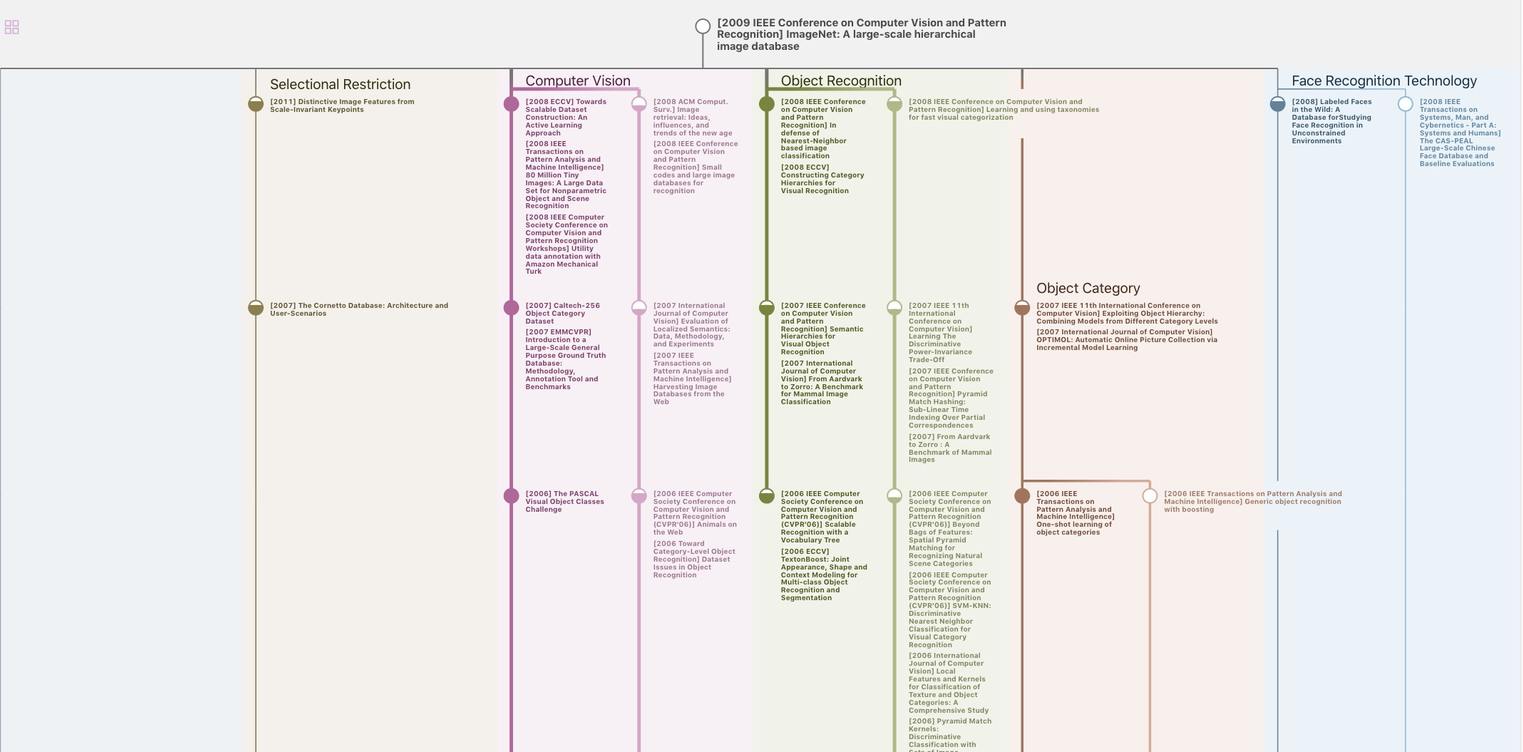
Generate MRT to find the research sequence of this paper
Chat Paper
Summary is being generated by the instructions you defined