Automatic Feature Extraction for Heartbeat Anomaly Detection
arXiv (Cornell University)(2021)
摘要
We focus on automatic feature extraction for raw audio heartbeat sounds, aimed at anomaly detection applications in healthcare. We learn features with the help of an autoencoder composed by a 1D non-causal convolutional encoder and a WaveNet decoder trained with a modified objective based on variational inference, employing the Maximum Mean Discrepancy (MMD). Moreover we model the latent distribution using a Gaussian chain graphical model to capture temporal correlations which characterize the encoded signals. After training the autoencoder on the reconstruction task in a unsupervised manner, we test the significance of the learned latent representations by training an SVM to predict anomalies. We evaluate the methods on a problem proposed by the PASCAL Classifying Heart Sounds Challenge and we compare with results in the literature.
更多查看译文
关键词
heartbeat anomaly detection,feature extraction
AI 理解论文
溯源树
样例
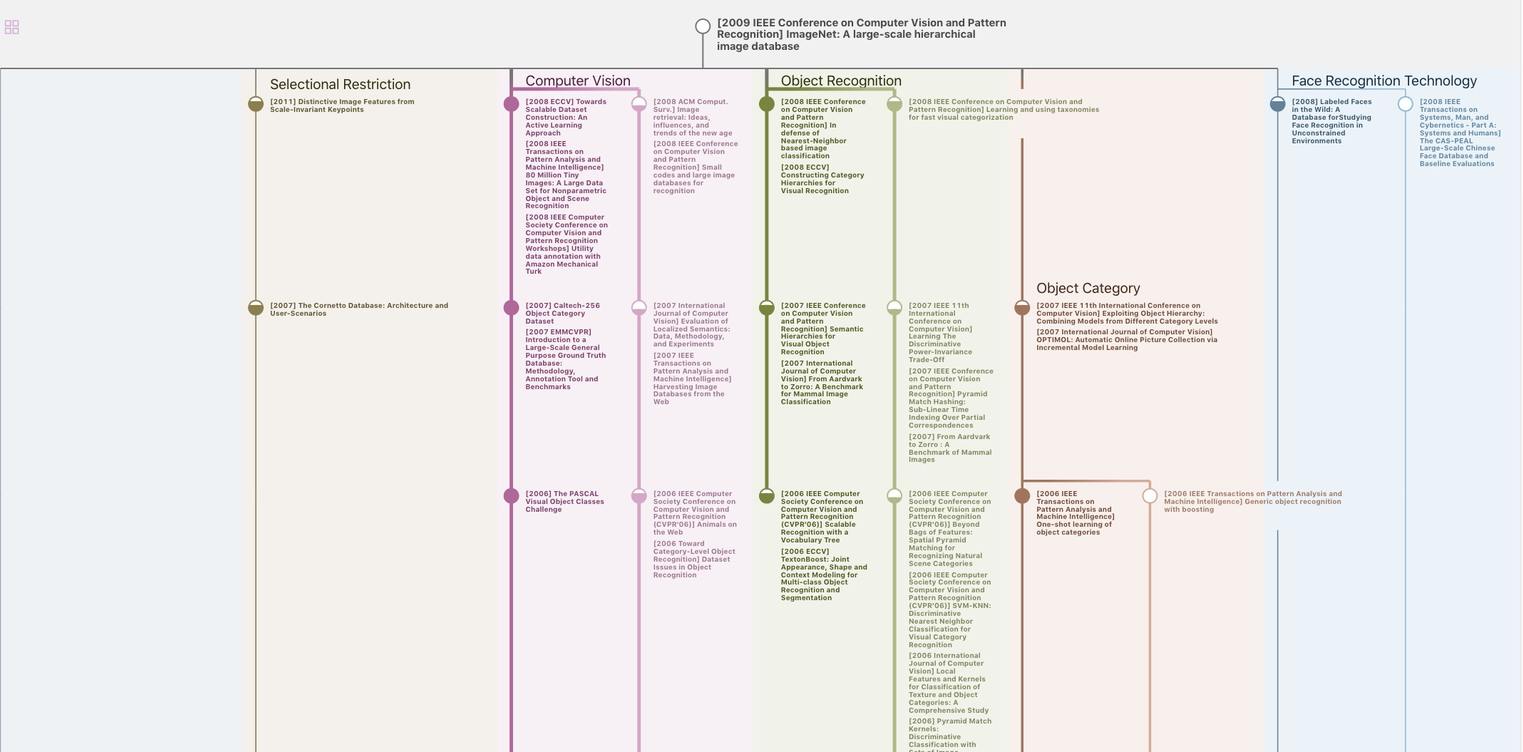
生成溯源树,研究论文发展脉络
Chat Paper
正在生成论文摘要