Identifying untrustworthy predictions in neural networks by geometric gradient analysis.
UAI(2021)
摘要
The susceptibility of deep neural networks to untrustworthy predictions, including out-of-distribution (OOD) data and adversarial examples, still prevent their widespread use in safety-critical applications. Most existing methods either require a re-training of a given model to achieve robust identification of adversarial attacks or are limited to out-of-distribution sample detection only. In this work, we propose a geometric gradient analysis (GGA) to improve the identification of untrustworthy predictions without retraining of a given model. GGA analyzes the geometry of the loss landscape of neural networks based on the saliency maps of their respective input. To motivate the proposed approach, we provide theoretical connections between gradients' geometrical properties and local minima of the loss function. Furthermore, we demonstrate that the proposed method outperforms prior approaches in detecting OOD data and adversarial attacks, including state-of-the-art and adaptive attacks.
更多查看译文
关键词
untrustworthy predictions,neural networks,gradient
AI 理解论文
溯源树
样例
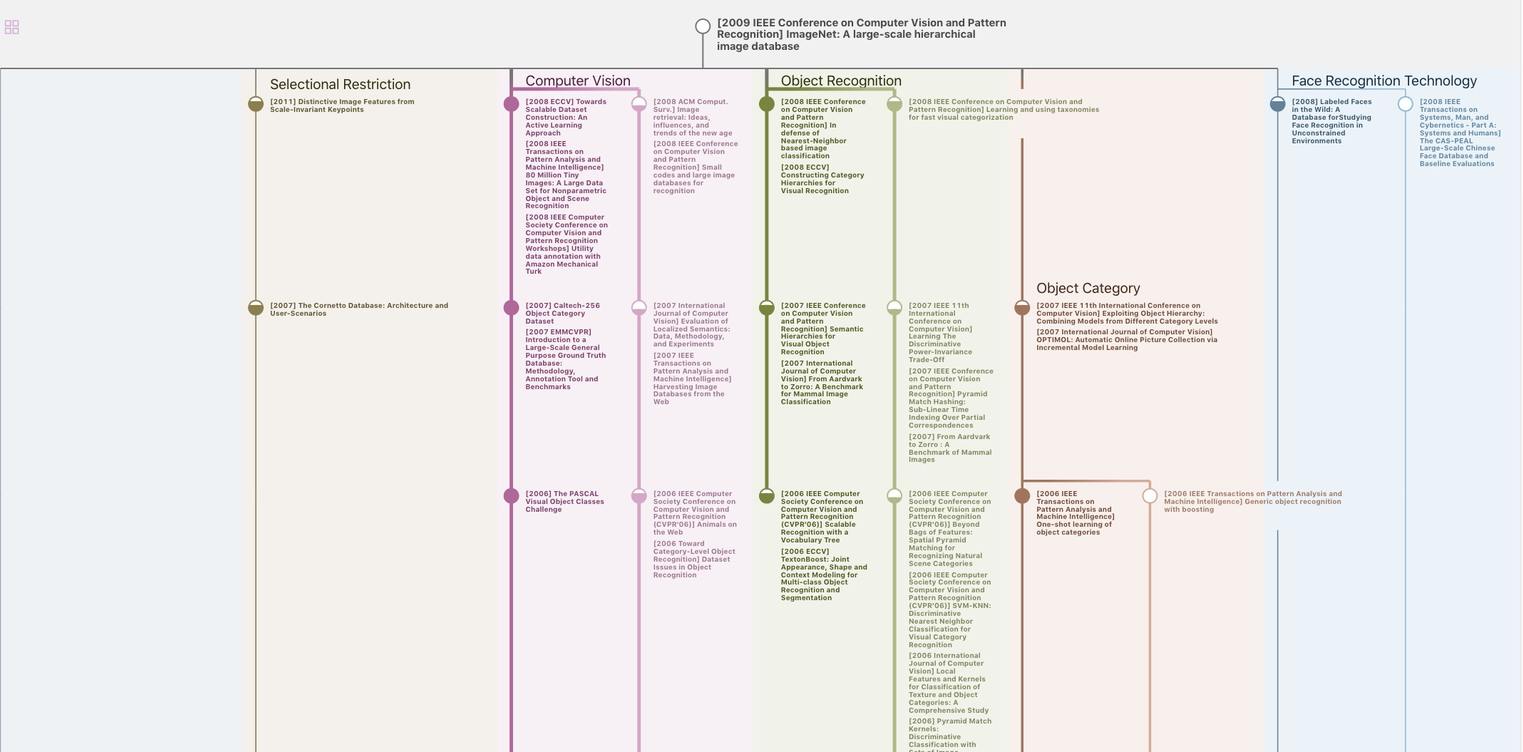
生成溯源树,研究论文发展脉络
Chat Paper
正在生成论文摘要