Adversarial Robustness with Non-uniform Perturbations.
Annual Conference on Neural Information Processing Systems(2021)
摘要
Robustness of machine learning models is critical for security related applications, where real-world adversaries are uniquely focused on evading neural network based detectors. Prior work mainly focus on crafting adversarial examples (AEs) with small uniform norm-bounded perturbations across features to maintain the requirement of imperceptibility. However, uniform perturbations do not result in realistic AEs in domains such as malware, finance, and social networks. For these types of applications, features typically have some semantically meaningful dependencies. The key idea of our proposed approach is to enable non-uniform perturbations that can adequately represent these feature dependencies during adversarial training. We propose using characteristics of the empirical data distribution, both on correlations between the features and the importance of the features themselves. Using experimental datasets for malware classification, credit risk prediction, and spam detection, we show that our approach is more robust to real-world attacks. Finally, we present robustness certification utilizing non-uniform perturbation bounds, and show that non-uniform bounds achieve better certification.
更多查看译文
关键词
perturbations,non-uniform
AI 理解论文
溯源树
样例
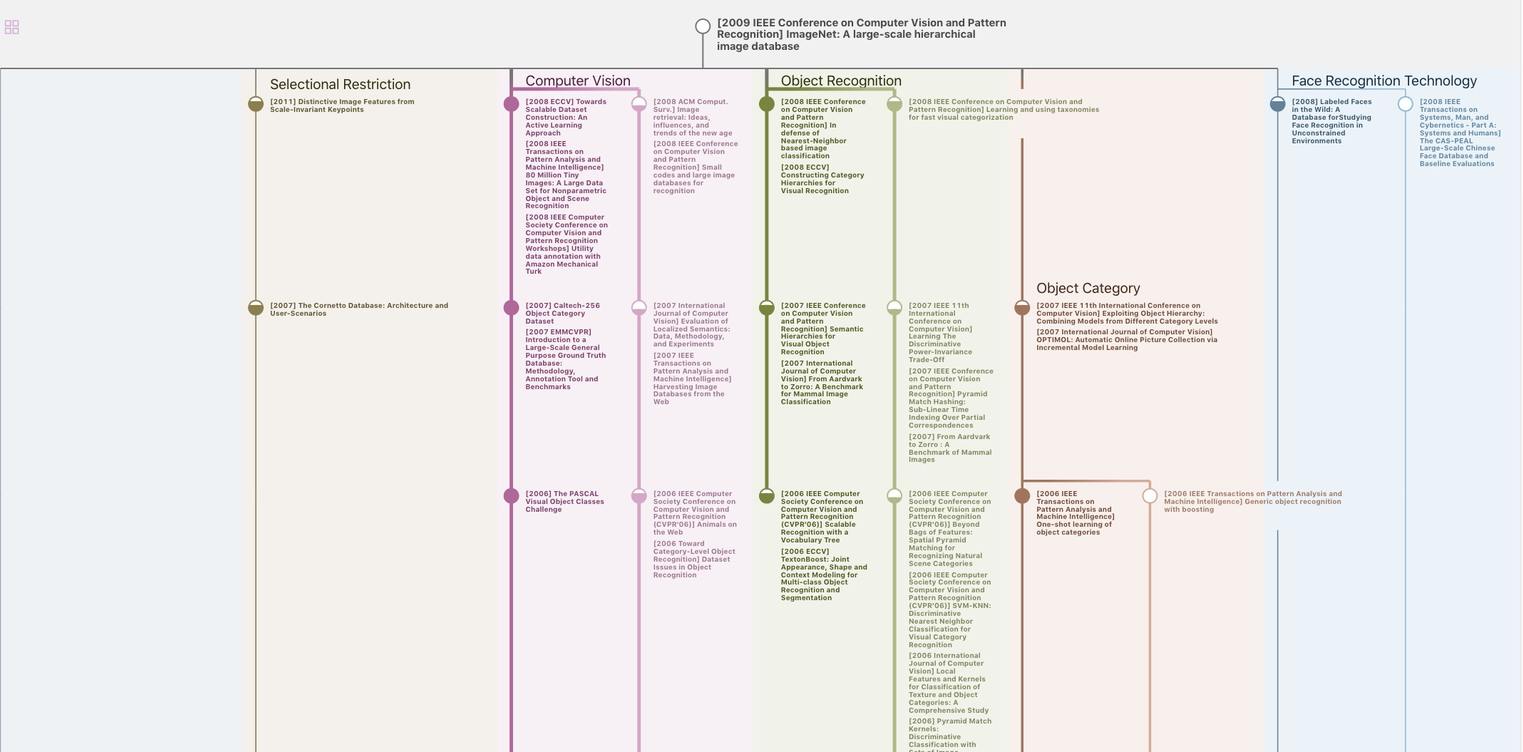
生成溯源树,研究论文发展脉络
Chat Paper
正在生成论文摘要