Predicting Dynamic Cellular Protein–rna Interactions by Deep Learning Using in Vivo RNA Structures
Cell research/Cell Research(2021)
关键词
Bioinformatics,Mechanisms of disease,Molecular biology,Life Sciences,general,Cell Biology
AI 理解论文
溯源树
样例
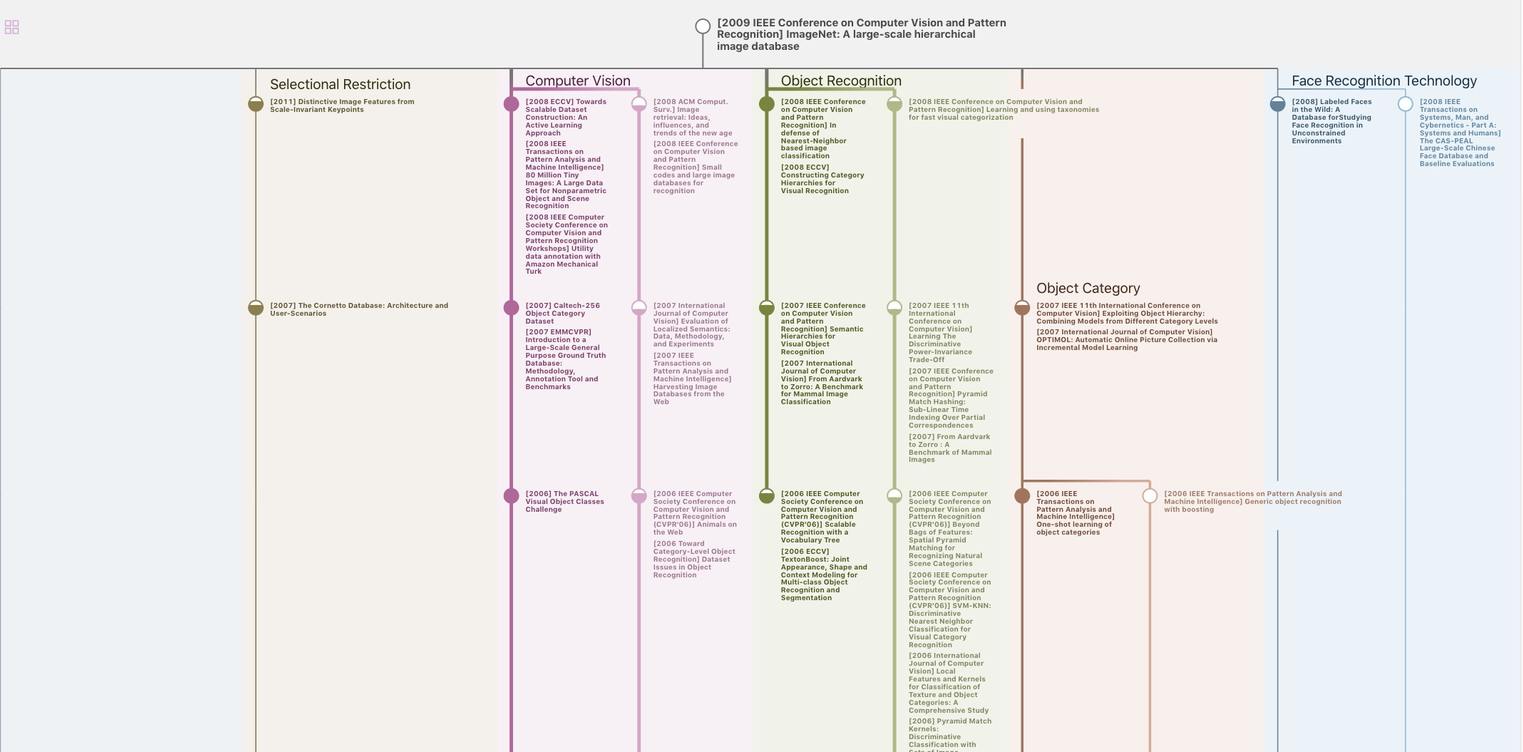
生成溯源树,研究论文发展脉络
Chat Paper
正在生成论文摘要