Online Learning-Based Co-task Dispatching with Function Configuration in Edge Computing.
PDCAT(2020)
摘要
Edge computing is a promising cloud computing paradigm that reduces computing latency by deploying edge servers near data sources and users, which is of great importance to implement delay-sensitive applications like AR, Cloud Gaming and Auto Driving. Due to the limited resources of edge servers, task dispatching and function configuration are the key to fully utilize edge servers. Moreover, a typical task request in edge computing (called a co-task) is consisted of a set of subtasks, where the task completion time is determined by the latest completed subtask. In this work, we propose a scheme named OnDisco, which combines reinforcement learning and heuristic methods to minimize the average completion time of co-tasks. Compared with heuristic algorithm, deep reinforcement learning can learn the inherent characteristics of the environment without any prior knowledge, and OnDisco is therefore well adapted to varying environments. Simulations on Alibaba traces shows that OnDisco reduces the average task completion time by 58 % and 76 % compared with the heuristic and random algorithm, respectively. Moreover, OnDisco outperforms the baselines consistently in various data environments and parameter settings.
更多查看译文
关键词
function configuration,learning-based,co-task
AI 理解论文
溯源树
样例
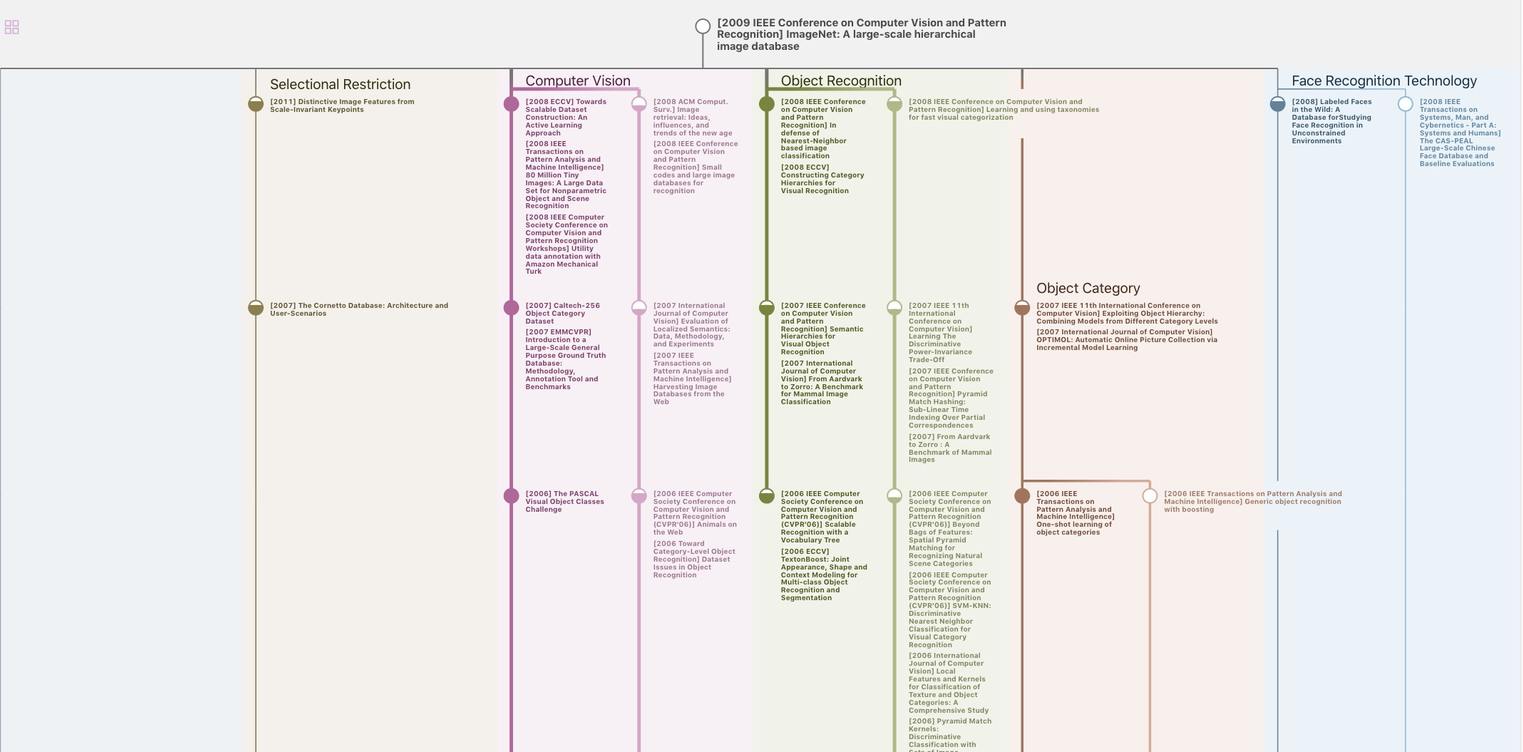
生成溯源树,研究论文发展脉络
Chat Paper
正在生成论文摘要