A Novel Multi-Object Distinction Method using deep learning
The Transactions of the Korean Institute of Electrical Engineers(2021)
Abstract
In this paper, we propose a novel multi-object distinction method with class-agnostic object detection and class retreival. Multi-object distinction is usually divided into the processes of detecting and classifying an object. Since it is common for industrial applications to add new kinds of objects to be recognized, it is inefficient to re-train the system every time the new object is added. Thus, the propose method employs two deep learning models to solve this problem. 1) Class agnostic object detection model to predict the bounding boxes regardless of the classes of objects and 2) Class retrieval model to determine the classes of the objects. The experimental results show that the proposed method successfully detects and classifies the both experienced and inexperienced objects: the final classification accuracy for 15 learned objects was 98.0%, and for the other 30 new objects that had not been learned. the accuracy was 87.7% on average.
MoreTranslated text
Key words
Detection,Surface Defect Detection,Fabric Defect Detection,Deep Learning
AI Read Science
Must-Reading Tree
Example
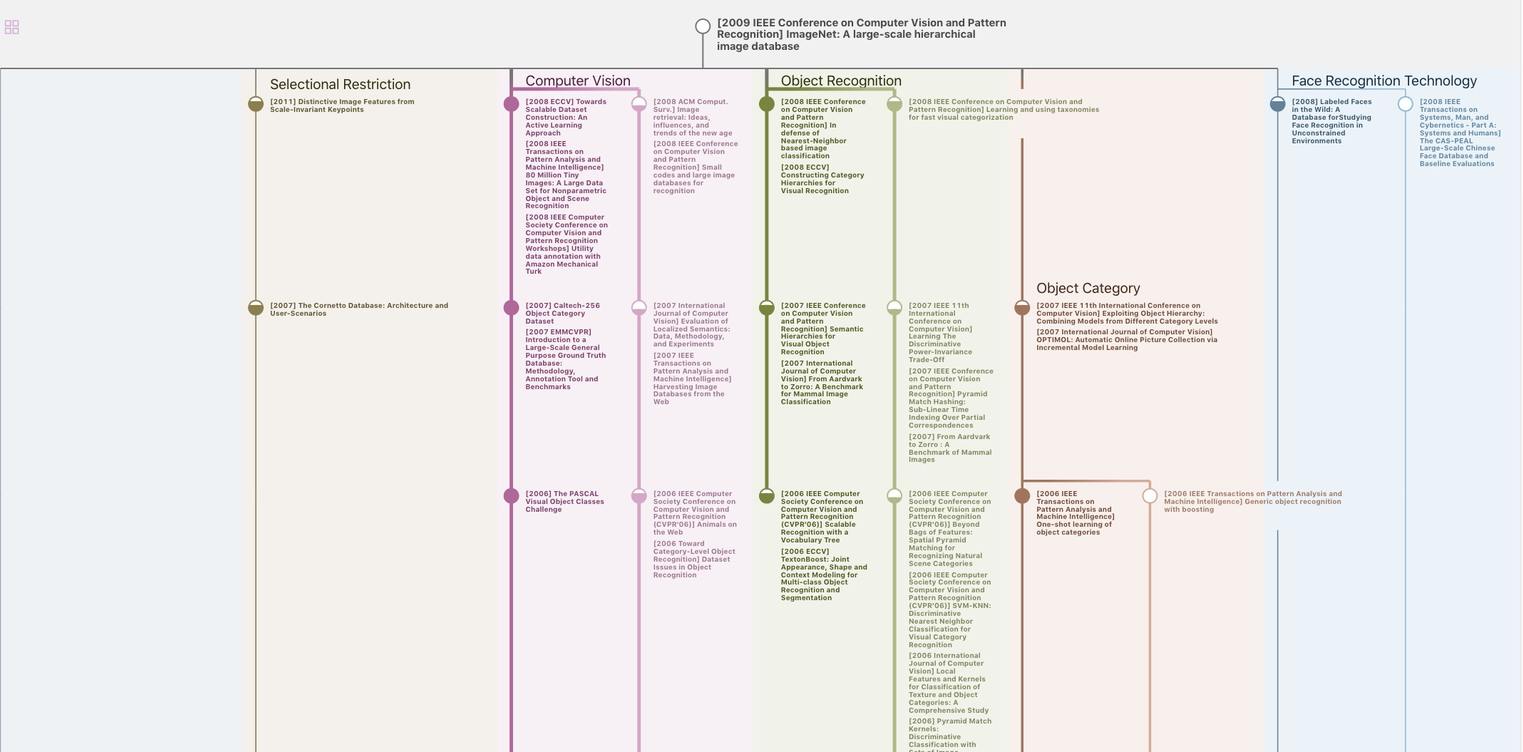
Generate MRT to find the research sequence of this paper
Chat Paper
Summary is being generated by the instructions you defined