Ensemble Learning-Based Cnn For Textile Fabric Defects Classification
INTERNATIONAL JOURNAL OF CLOTHING SCIENCE AND TECHNOLOGY(2021)
摘要
Purpose Classifying the types of fabric defects in the textile industry requires a way to effectively detect. The traditional textile fabric defects detection method is human eyes, which performs very low efficiency and high cost. Therefore, how to improve the classification accuracy of textile fabric defects by using current artificial intelligence and to better meet the needs in the textile industry, the purpose of this article is to develop a method to improve the accuracy of textile fabric defects classification. Design/methodology/approach To improve the accuracy of textile fabric defects classification, an ensemble learning-based convolutional neural network (CNN) method in terms of textile fabric defects classification (short for ECTFDC) on an enhanced TILDA database is used. ECTFDC first adopts ensemble learning-based model to classify five types of fabric defects from TILDA. Subsequently, ECTFDC extracts features of fabric defects via an ensemble multiple convolutional neural network model and obtains parameters by using transfer learning method. Findings The authors applied ECTFDC on an enhanced TILDA database to improve the robustness and generalization ability of the proposed networks. Experimental results show that ECTFDC outperforms the other networks, the precision and recall rates are 97.8%, 97.68%, respectively. Originality/value The ensemble convolutional neural network textile fabric defect classification method in this paper can quickly and effectively classify textile fabric defect categories; it can reduce the production cost of textiles and it can alleviate the visual fatigue of inspectors working for a long time.
更多查看译文
关键词
Ensemble learning, Convolutional neural network, Transfer learning, Small simples, Textile fabric defect classification
AI 理解论文
溯源树
样例
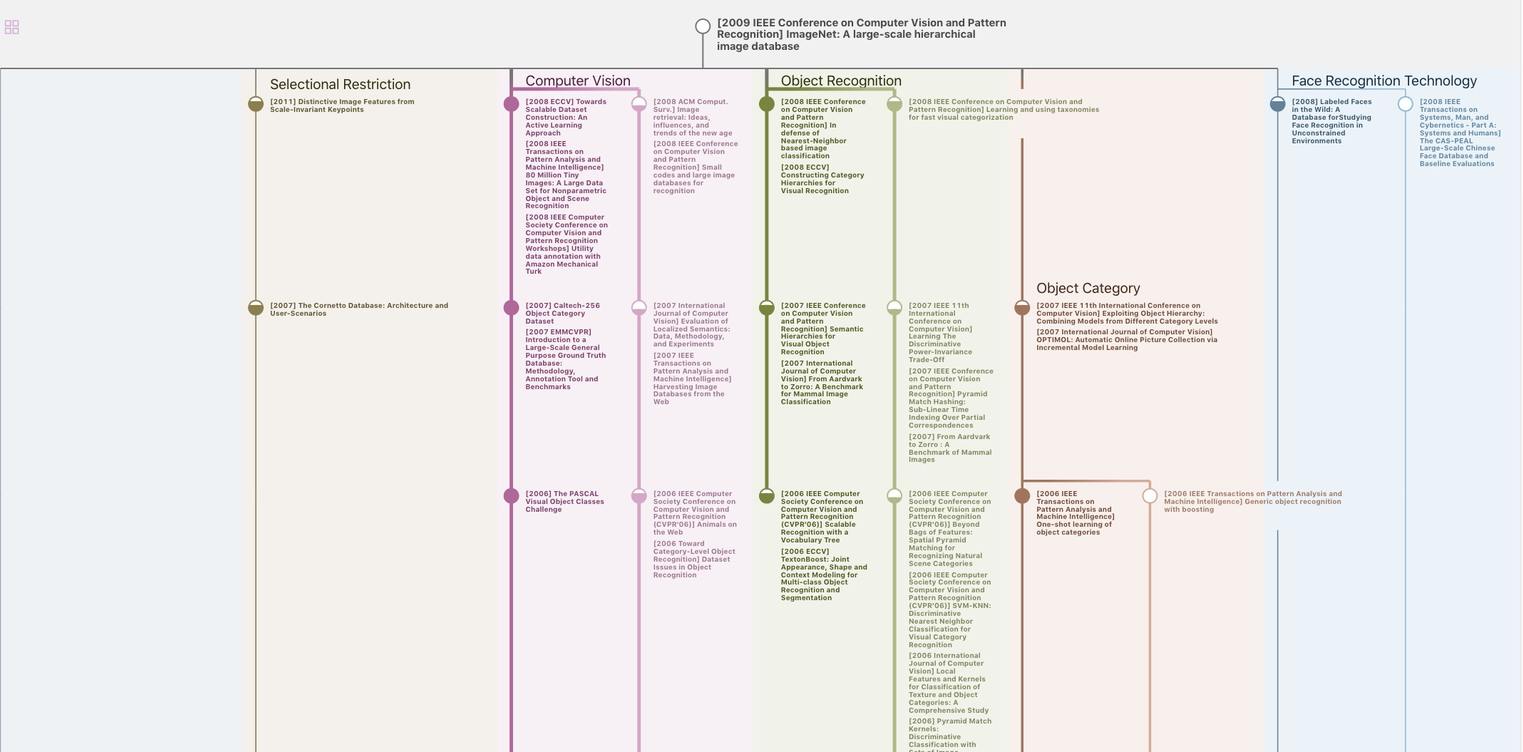
生成溯源树,研究论文发展脉络
Chat Paper
正在生成论文摘要