Uncertainty Quantification In Fault Detection Using Convolutional Neural Networks
GEOPHYSICS(2021)
摘要
Segmentation of faults based on seismic images is an important step in reservoir characterization. With the recent developments of deep-learning methods and the availability of massive computing power, automatic interpretation of seismic faults has become possible. The likelihood of occurrence for a fault can be quantified using a sigmoid function. Our goal is to quantify the fault model uncertainty that is generally not captured by deeplearning tools. We have used the dropout approach, a regularization technique to prevent overfitting and coadaptation in hidden units, to approximate the Bayesian inference and estimate the principled uncertainty over functions. Particularly, the variance of the learned model has been decomposed into alea-toric and epistemic parts. Our method is applied to a real data set from the Netherlands F3 block with two different dropout ratios in convolutional neural networks. The aleatoric uncertainty is irreducible because it relates to the stochastic dependency within the input observations. As the number of Monte Carlo realizations increases, the epistemic uncertainty asymptotically converges and the model standard deviation decreases because the variability of the model parameters is better simulated or explained with a larger sample size. This analysis can quantify the confidence to use fault predictions with less uncertainty. In addition, the analysis suggests where more training data are needed to reduce the uncertainty in low-confidence regions.
更多查看译文
关键词
fault detection,uncertainty,quantification
AI 理解论文
溯源树
样例
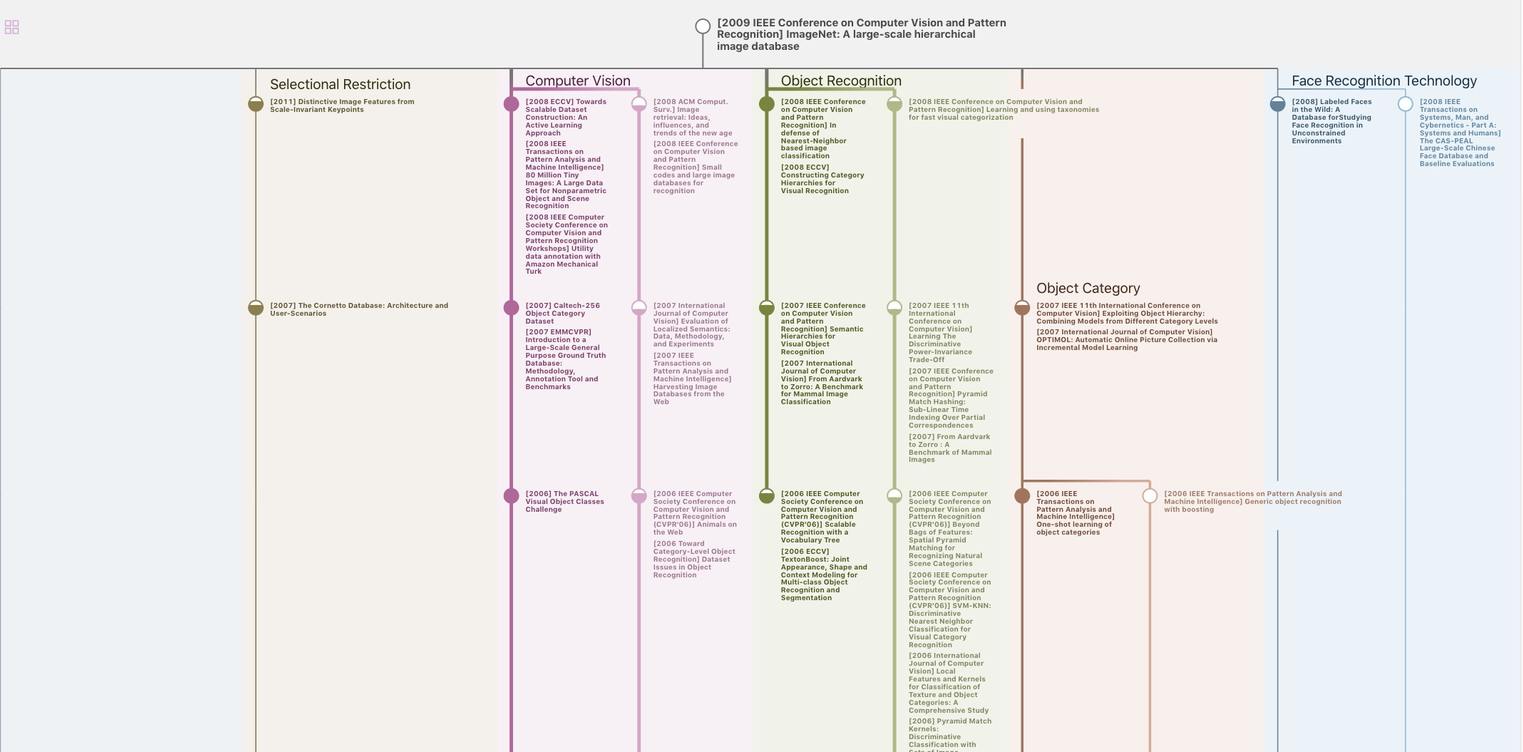
生成溯源树,研究论文发展脉络
Chat Paper
正在生成论文摘要