Medical Image Super-Resolution Using A Relativistic Average Generative Adversarial Network
NUCLEAR INSTRUMENTS & METHODS IN PHYSICS RESEARCH SECTION A-ACCELERATORS SPECTROMETERS DETECTORS AND ASSOCIATED EQUIPMENT(2021)
摘要
The medical imaging technique, e.g., positron emission tomography (PET), X-ray computed tomography (CT), and magnetic resonance imaging (MRI) is essential for clinical diagnosis and nuclear medicine. However, due to the hardware limitations of scanners, it is always clinically challenging to obtain high-resolution (HR) medical images. With the development of artificial intelligence, image super-resolution has been an effective technique to enhance the spatial resolution of medical images. In this paper, we propose a novel medical image super-resolution method using a relativistic average generative adversarial network (GAN), which consists of a generator and a discriminator for enhancing medical imaging quality in terms of both numerical criteria and visual results. The generator is trained to reconstruct HR images according to low-resolution (LR) counterparts. In contrast, the discriminator is trained to discriminate the probability of whether real HR images are more realistic than reconstructed images, further enhancing visual results. We apply our proposed method to two different public medical datasets, and experimental results show that our proposed method outperforms in terms of visual results, peak signal-to-noise ratio (PSNR), structural similarity index (SSIM), model complexity and an additional non-reference image quality assessment metric, compared with other state-of-the-art medical image super-resolution methods.
更多查看译文
关键词
Medical image super-resolution, Relativistic average generative adversarial network, Residual neural network, Attention mechanism
AI 理解论文
溯源树
样例
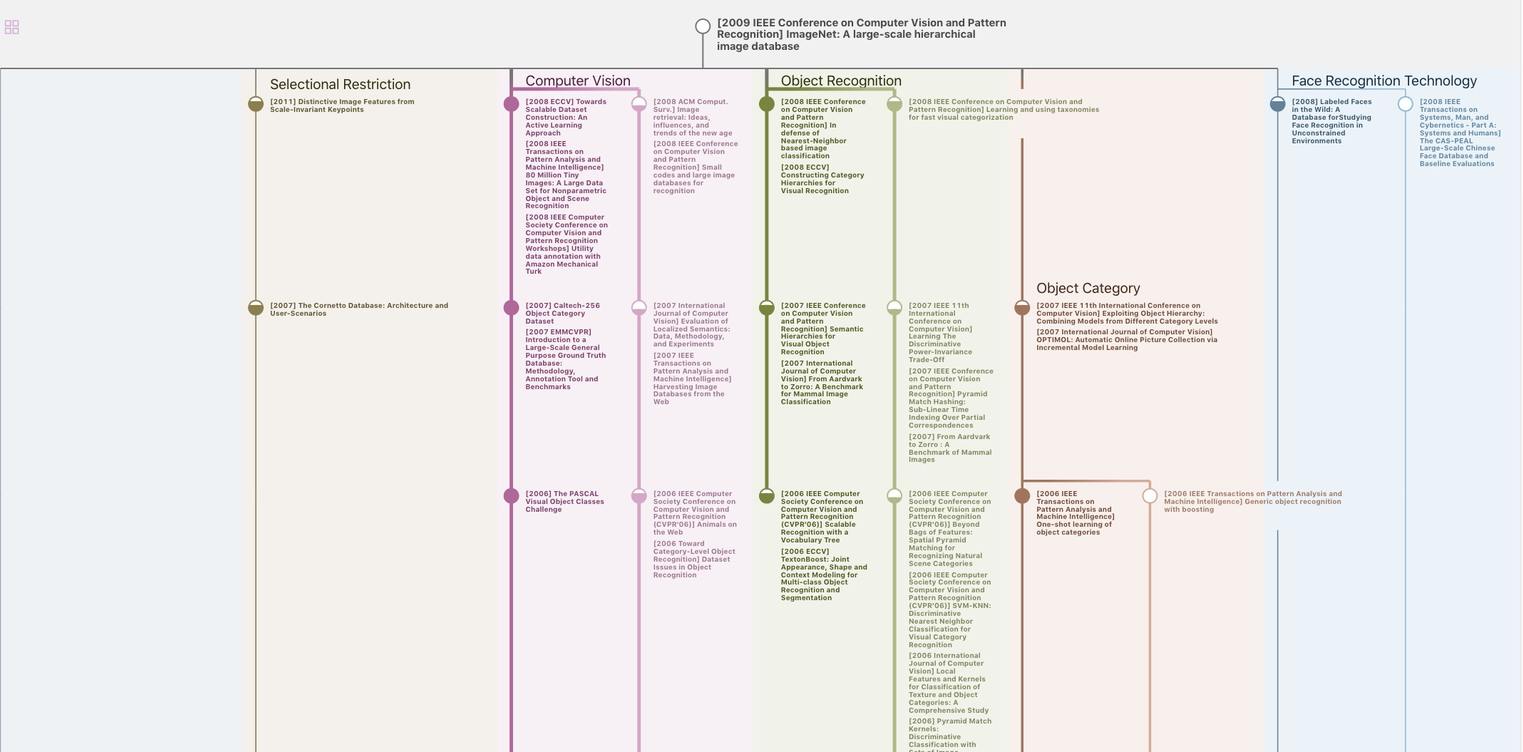
生成溯源树,研究论文发展脉络
Chat Paper
正在生成论文摘要