Recurrent convolutional neural network for session-based recommendation.
Neurocomputing(2021)
摘要
The task of session-based recommendation is predicting the next recommendation item when available information only includes the anonymous behavior sequence. Previous methods of session-based recommendation usually integrate the general interest, dynamic interest, and current interest to promote recommendation performance. However, most existing methods ignore the non-monotone feature interactions when building user’s dynamic interest and model item-item transitions through a linear way when building user’s current interest, which reduces the performance of model. In this paper, we design a novel method for session-based recommendation with recurrent and convolutional neural network. Specifically, The Gated Recurrent Unit with item-level attention mechanism learns the user’s general interest, while the convolutional operation with horizontal filter and vertical filter search for user’s current interest and dynamic interest. Moreover, the outputs of recurrent operation and convolutional operation are concatenated to generate the recommendation. Furthermore, we evaluate the proposed model on three real-world datasets which come from e-commerce and music API, respectively. The experimental results show that our model outperforms the state-of-the-art methods on session-based recommendation.
更多查看译文
关键词
Session-based recommendation,General interest,Dynamic interest,Current interest
AI 理解论文
溯源树
样例
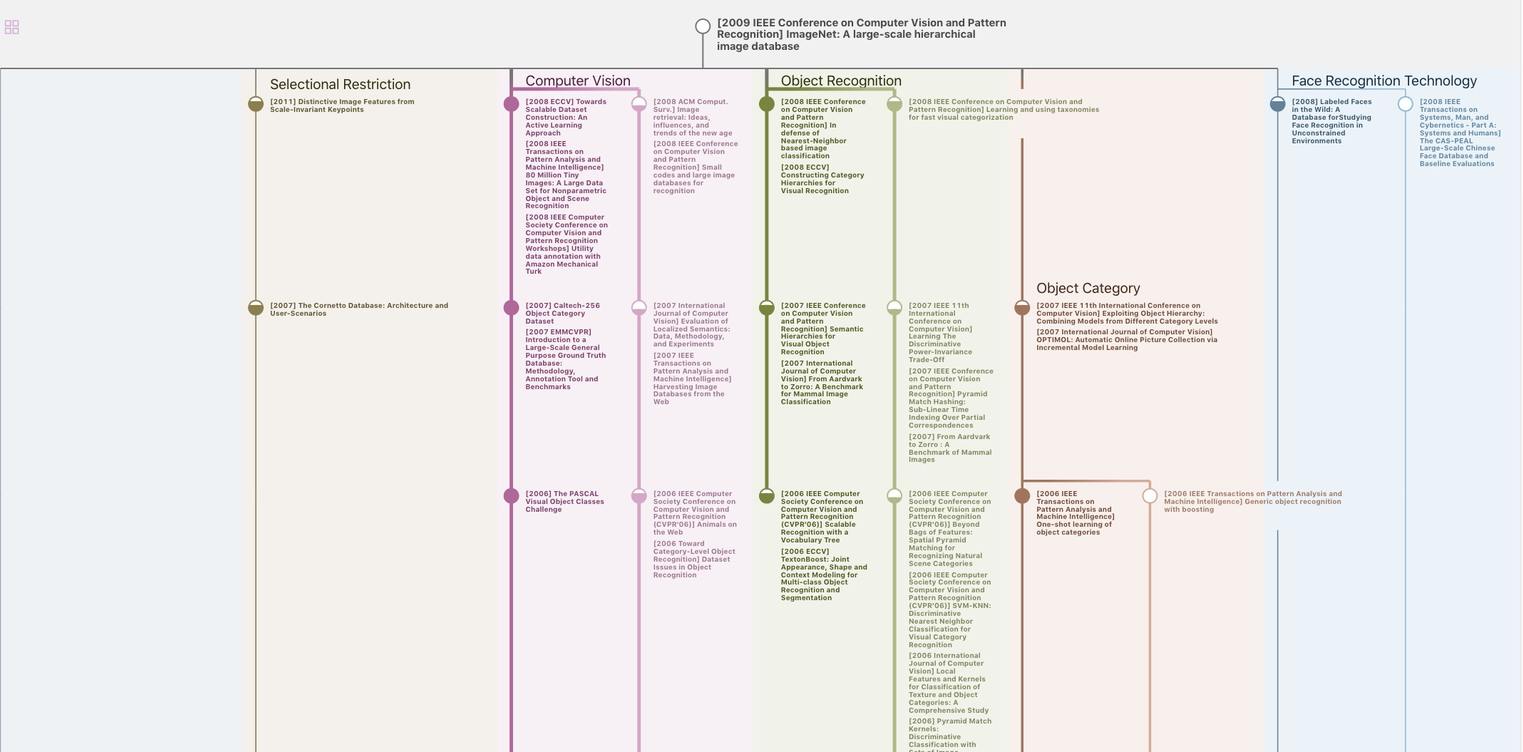
生成溯源树,研究论文发展脉络
Chat Paper
正在生成论文摘要