Bit-Quantized-Net: An Effective Method for Compressing Deep Neural Networks.
Mob. Networks Appl.(2021)
摘要
Deep neural networks have achieved state-of-the-art performances in wide range scenarios, such as natural language processing, object detection, image classification, speech recognition, etc. While showing impressive results across these machine learning tasks, neural network models still suffer from computational consuming and memory intensive for parameters training/storage on mobile service scenario. As a result, how to simplify models as well as accelerate neural networks are undoubtedly to be crucial research topic. To address this issue, in this paper, we propose “Bit-Quantized-Net”(BQ-Net), which can compress deep neural networks both at the training phase and testing inference. And, the model size can be reduced by compressing bit quantized weights. Specifically, for training or testing plain neural network model, it is running tens of millions of times of y=wx+b computations. In BQ-Net, however, model approximate the computation operation y = w x + b by y = s i g n ( w )( x ≫| w |) + b during forward propagation of neural networks. That is, BQ-Net trains the networks with bit quantized weights during forwarding propagation, while retaining the full precision weights for gradients accumulating during backward propagation. Finally, we apply Huffman coding to encode the bit shifting weights which compressed the model size in some way. Extensive experiments on three real data-sets (MNIST, CIFAR-10, SVHN) show that BQ-Net can achieve 10-14× model compressibility.
更多查看译文
AI 理解论文
溯源树
样例
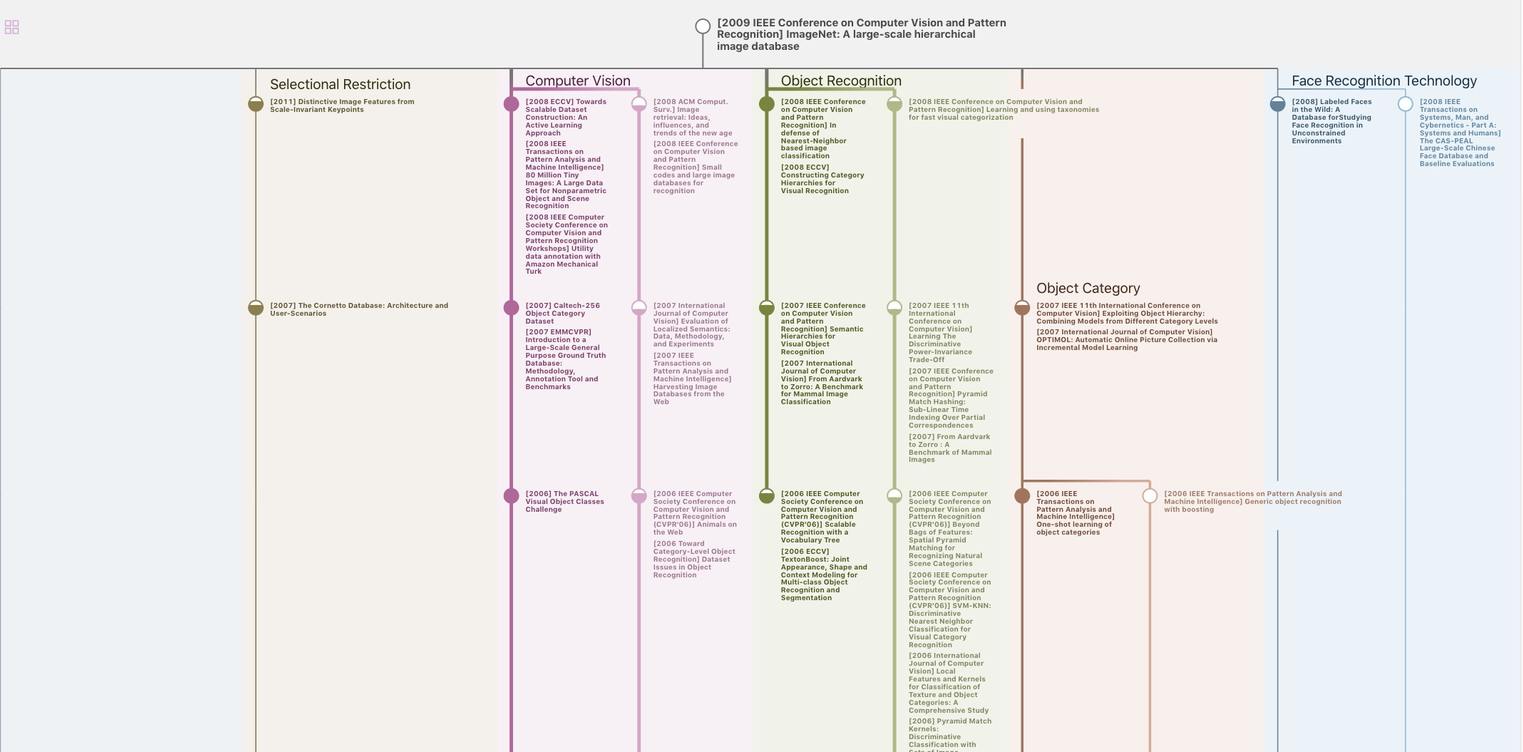
生成溯源树,研究论文发展脉络
Chat Paper
正在生成论文摘要