SAR Image Change Detection Based on Semisupervised Learning and Two-Step Training.
IEEE Geosci. Remote. Sens. Lett.(2022)
Abstract
Change detection, as an essential part of synthetic aperture radar (SAR) automatic target recognition (ATR) systems, remains a challenging problem. The lack of labeled data seriously impedes the development of deep learning-based methods in SAR applications. In this letter, we propose a patch-based semisupervised method to detect changed pixels from limited training data in the field of SAR. The complete approach includes the unsupervised pretraining stage and the iterative discrimination stage. The states of pixels are determined by comparing the corresponding image patches. First, to reduce the impact of insufficient data, we train a trapezium U-Net-like structure to extract representative and generalized features by unsupervised training on all pixels. Feature maps contain detailed and semantic information due to repetitive feature fusions. A designed feature activation module is utilized to recalibrate fused features. Finally, the discrimination is finished by a simple classification convolutional neural network (CNN) following a two-step training strategy. Ablation studies indicate the function provided by the proposed modifications. Experiments on real SAR images demonstrate that the proposed method can improve the detection accuracy by more than 1.2% compared with other state-of-the-art deep learning-based methods.
MoreTranslated text
Key words
Change detection,channel-attention,feature fusion,pseudolabels,semisupervised,synthetic aperture radar (SAR)
AI Read Science
Must-Reading Tree
Example
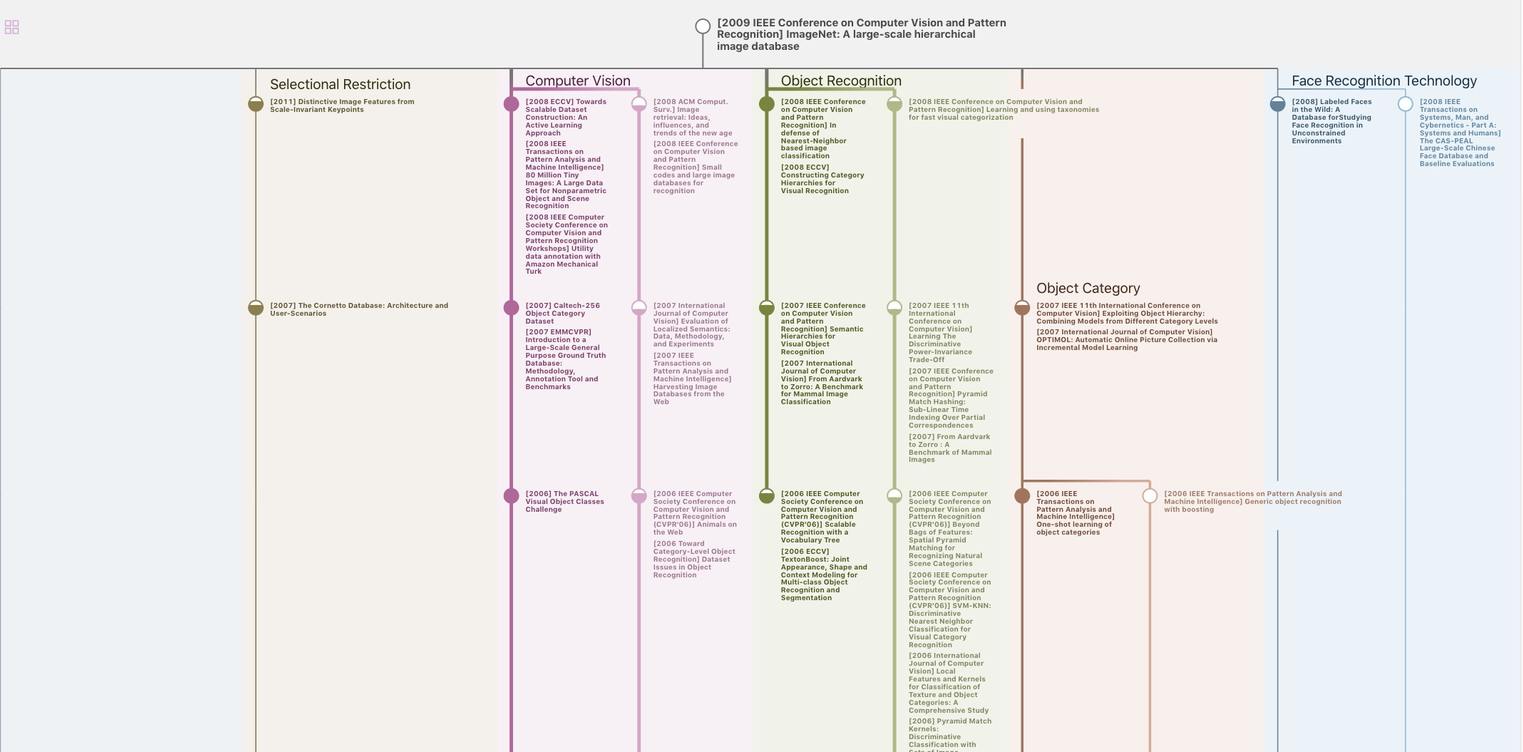
Generate MRT to find the research sequence of this paper
Chat Paper
Summary is being generated by the instructions you defined