Deep Residual Network-Based Fusion Framework For Hyperspectral And Lidar Data
IEEE JOURNAL OF SELECTED TOPICS IN APPLIED EARTH OBSERVATIONS AND REMOTE SENSING(2021)
摘要
This article presents a deep residual network-based fusion framework for hyperspectral and LiDAR data. In this framework, three new fusion methods are proposed, which are the residual network-based deep feature fusion (RNDFF), the residual network-based probability reconstruction fusion (RNPRF) and the residual network-based probability multiplication fusion (RNPMF). The three methods use extinction profile (EP), local binary pattern (LBP), and deep residual network. Specifically, EP and LBP features are extracted from two sources and stacked as spatial features. For RNDFF, the deep features of each source are extracted by a deep residual network, and then the deep features are stacked to create the fusion features which are classified by softmax classifier. For RNPRF, the deep features of each source are input to the softmax classifier to obtain the probability matrices, and then the probability matrices are fused by weighted addition to producing the final label assignment. For RNPMF, the probability matrices are fused by array multiplication. Experimental results demonstrate that the classification performance of the proposed methods significantly outperform existing methods in hyperspectral and LiDAR data fusion.
更多查看译文
关键词
Laser radar, Feature extraction, Hyperspectral imaging, Residual neural networks, Stacking, Data mining, Training, Deep residual network, extinction profile, Goddard &apos, s LiDAR, hyperspectral, hyperspectral and thermal (G-LiHT) data, image fusion, local binary pattern (LBP), probability fusion, light detection and ranging (LiDAR)
AI 理解论文
溯源树
样例
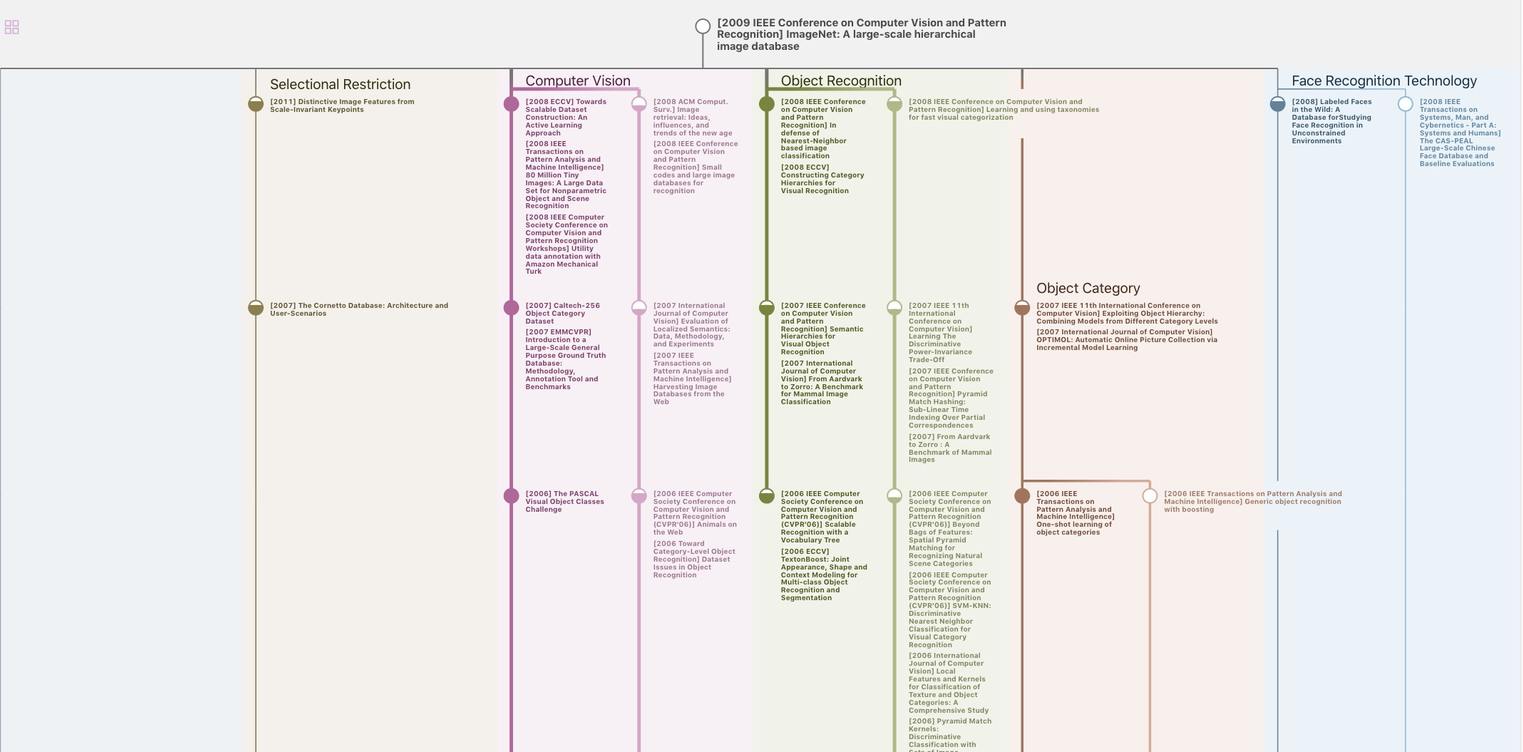
生成溯源树,研究论文发展脉络
Chat Paper
正在生成论文摘要