Learning Image-Based Receding Horizon Planning For Manipulation In Clutter
ROBOTICS AND AUTONOMOUS SYSTEMS(2021)
摘要
The manipulation of an object into a desired location in a cluttered and restricted environment requires reasoning over the long-term consequences of an action while reacting locally to the multiple physics-based interactions. We present Visual Receding Horizon Planning (VisualRHP) in a framework which interleaves real-world execution with look-ahead planning to efficiently solve a short-horizon approximation to a multi-step sequential decision making problem. VisualRHP is guided by a learned heuristic that acts on an abstract colour-labelled image-based representation of the state. With this representation, the robot can generalize its behaviours to different environment setups, that is, different number and shape of objects, while also having transferable manipulation skills that can be applied to a multitude of real-world objects. We train the heuristic with imitation and reinforcement learning in discrete and continuous actions spaces. We detail our heuristic learning process for environments with sparse rewards, and non-linear, non-continuous, dynamics. In particular, we introduce necessary changes for improving the stability of existing reinforcement learning algorithms that use neural networks with shared parameters. In a series of simulation and real-world experiments, we show the robot performing prehensile and non-prehensile actions in synergy to successfully manipulate a variety of real-world objects in real-time. (C) 2021 Elsevier B.V. All rights reserved.
更多查看译文
关键词
Manipulation in clutter, Physics-based manipulation, Heuristic learning, Receding Horizon Planning, Imitation and reinforcement learning, Abstract state representation
AI 理解论文
溯源树
样例
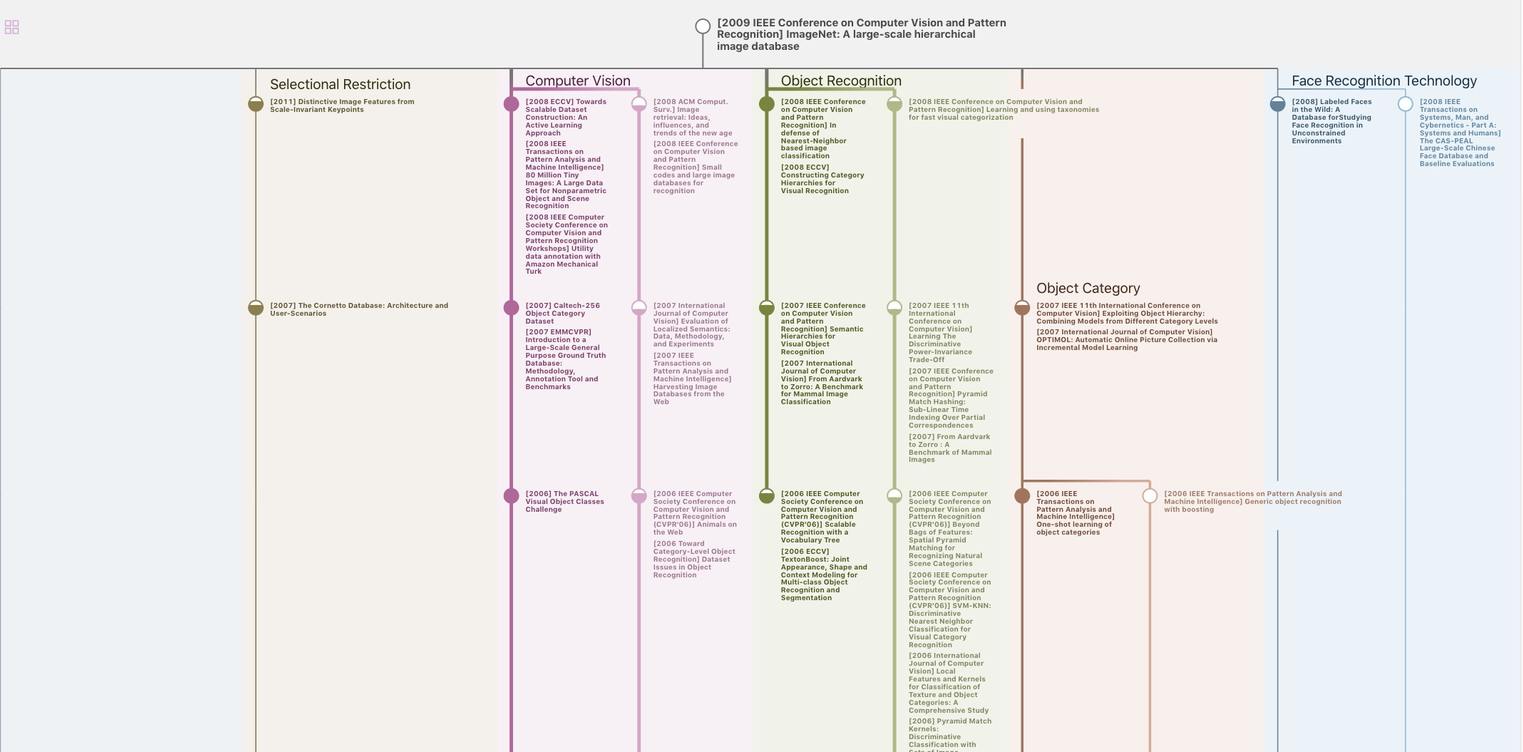
生成溯源树,研究论文发展脉络
Chat Paper
正在生成论文摘要