Measurement of real-world roadway emission rates through a fitted dispersion model (Withdrawal of Vol 12, Pg 75, 2021)
ATMOSPHERIC POLLUTION RESEARCH(2022)
摘要
This paper presents a methodology for estimating fleet emission rates from measured roadside concentrations. By filtering measurements based on meteorological conditions, including effective wind speeds above 2 m s(-1) and periods where the receptor is downwind, we find our simplified approach can compare well with the more sophisticated Research LINE source (RLINE) model. We applied our method to two years of roadside air pollution and traffic measurements at a Toronto, Canada, highway site to estimate minutely emission rates (ER, mass.m(-1).s(-1)) and emission factors (EF, mass.vehicle 1.km(-1)) for carbon dioxide (CO2), nitrogen oxides (NOX), carbon monoxide (CO), black carbon (BC), particulate matter mass less than 2.5 mu m in diameter (PM2.5), particle number (PN), and ozone (O-3) over a two-year period. Re-entering these emission rates to a multi-lane RLINE model showed favorable agreement between predicted and measured concentrations for all pollutants with 85-87% of predicted concentrations falling within a factor of two of measured. A multiple-input linear regression was used to determine light-duty vehicle (LDV) and medium/heavy-duty vehicle (MDV + HDV)-specific emission factors, which fell in or near ranges previously reported for all pollutants. More generally, the method proposed here can allow researchers to easily measure emission rates and factors from roadways using near-road concentration measurements and simple analysis methods, and can exclude some or all micro-meteorological inputs, allowing researchers to perform inverse dispersion modeling in regions where such inputs are unavailable. The results also provide updated data on Canadian vehicle emissions and refine the relationships between emissions and traffic composition and speed.
更多查看译文
关键词
Emission rates, Traffic emissions, Highway, Emission factors, Dispersion modeling, Background subtraction
AI 理解论文
溯源树
样例
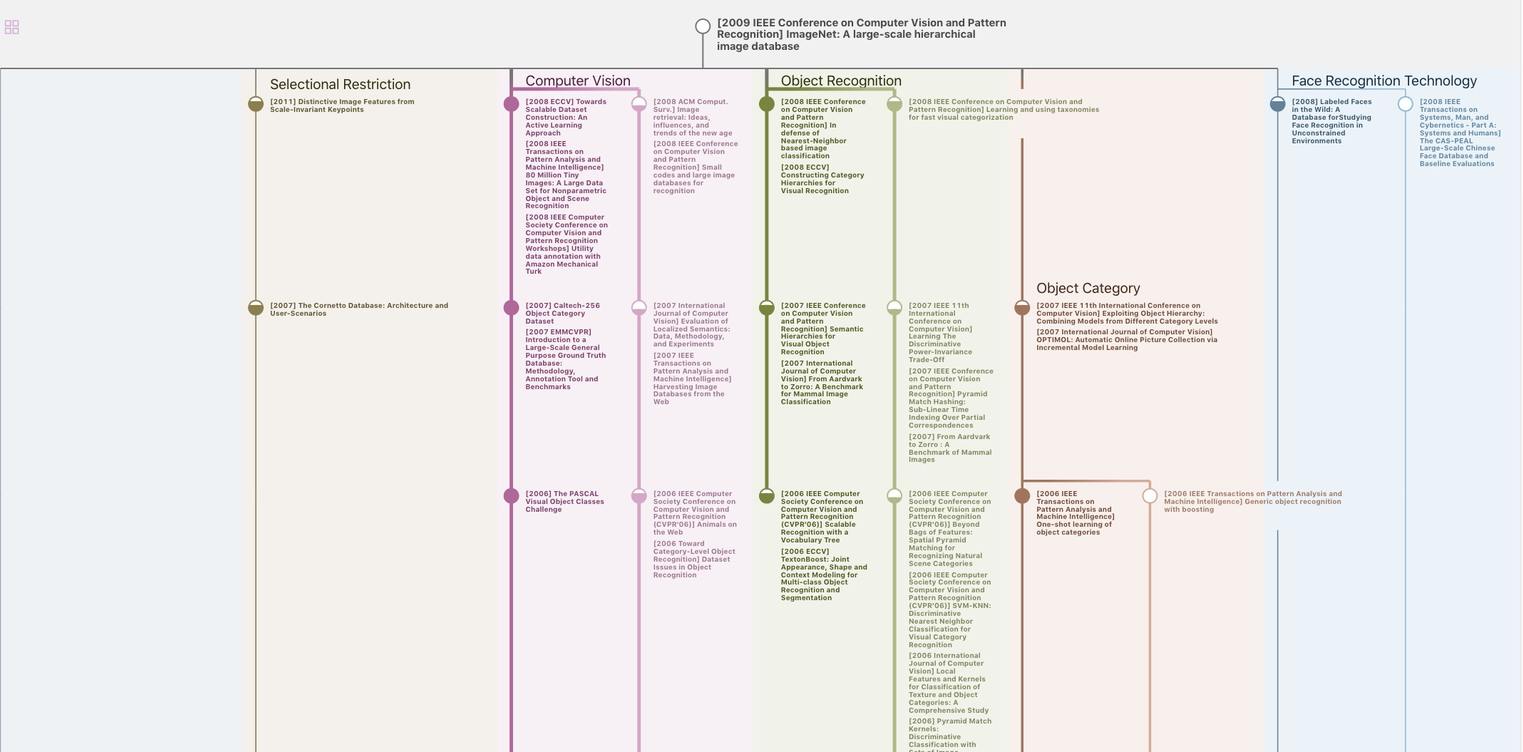
生成溯源树,研究论文发展脉络
Chat Paper
正在生成论文摘要