Optimal model averaging forecasting in high-dimensional survival analysis
International Journal of Forecasting(2021)
摘要
This article considers ultrahigh-dimensional forecasting problems with survival response variables. We propose a two-step model averaging procedure for improving the forecasting accuracy of the true conditional mean of a survival response variable. The first step is to construct a class of candidate models, each with low-dimensional covariates. For this, a feature screening procedure is developed to separate the active and inactive predictors through a marginal Buckley–James index, and to group covariates with a similar index size together to form regression models with survival response variables. The proposed screening method can select active predictors under covariate-dependent censoring, and enjoys sure screening consistency under mild regularity conditions. The second step is to find the optimal model weights for averaging by adapting a delete-one cross-validation criterion, without the standard constraint that the weights sum to one. The theoretical results show that the delete-one cross-validation criterion achieves the lowest possible forecasting loss asymptotically. Numerical studies demonstrate the superior performance of the proposed variable screening and model averaging procedures over existing methods.
更多查看译文
关键词
Health forecasting,Simulation,Feature screening,Model averaging,Survival analysis,Right-censored data,Ultra-high dimensional data
AI 理解论文
溯源树
样例
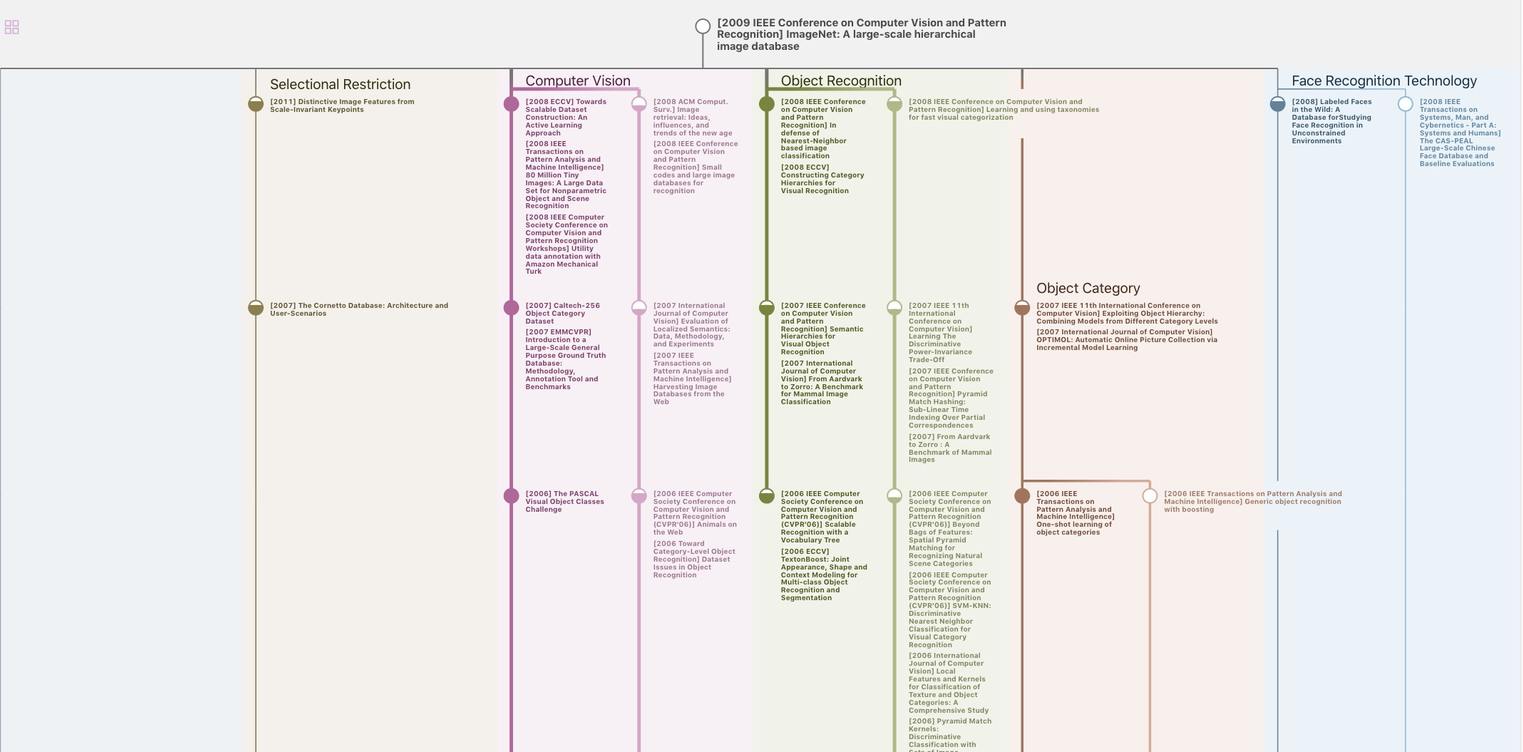
生成溯源树,研究论文发展脉络
Chat Paper
正在生成论文摘要