Attention-Based Sequence-to-Sequence Learning for Online Structural Response Forecasting Under Seismic Excitation
IEEE Transactions on Systems, Man, and Cybernetics: Systems(2022)
摘要
In structural health monitoring (SHM), measuring and evaluating structural dynamic responses are critical for safety management of civil infrastructures. Particularly, online forecasting of the structural responses under extreme external loading conditions (e.g., earthquakes) takes a significant role in SHM to provide early warning and ensure safe operation. In practice, complex causality and intrinsic interactions between seismic excitation and structural response make it challenging to establish a reliable predictive scheme. The present paper proposes a novel deep recurrent neural network (RNN) model implemented in the architecture of a time-series attention-based RNN encoder–decoder (TSA-RNN-ED), for predictive analysis of structural responses under seismic excitation. In the proposed data-driven model, upcoming sequential responses are predicted through sequence-to-sequence learning from historical multivariate time-series signals. A time-series attention mechanism is proposed to exploit the heterogeneous, but directly related, hidden features between the seismic loads and the corresponding structural responses. The proposed architecture can reliably regress excitation-response interactions to predict dynamic responses subjected to future earthquakes while satisfying the need of real-time forecasting for on-site practical implementation. This article systematically evaluates the proposed model by using two real-world structural cases: 1) the tallest building in China, the Shanghai Tower and 2) a woodframe classroom on a shake table at the University of British Columbia in Vancouver, Canada. The experimental results demonstrate the accurate and efficient performance of the proposed methodology in forecasting the seismic responses of the structures under investigation.
更多查看译文
关键词
Earthquake ground motion,real-time analytics,recurrent neural network (RNN) encoder–decoder,sequence prediction,structural response forecasting,time-series attention mechanism
AI 理解论文
溯源树
样例
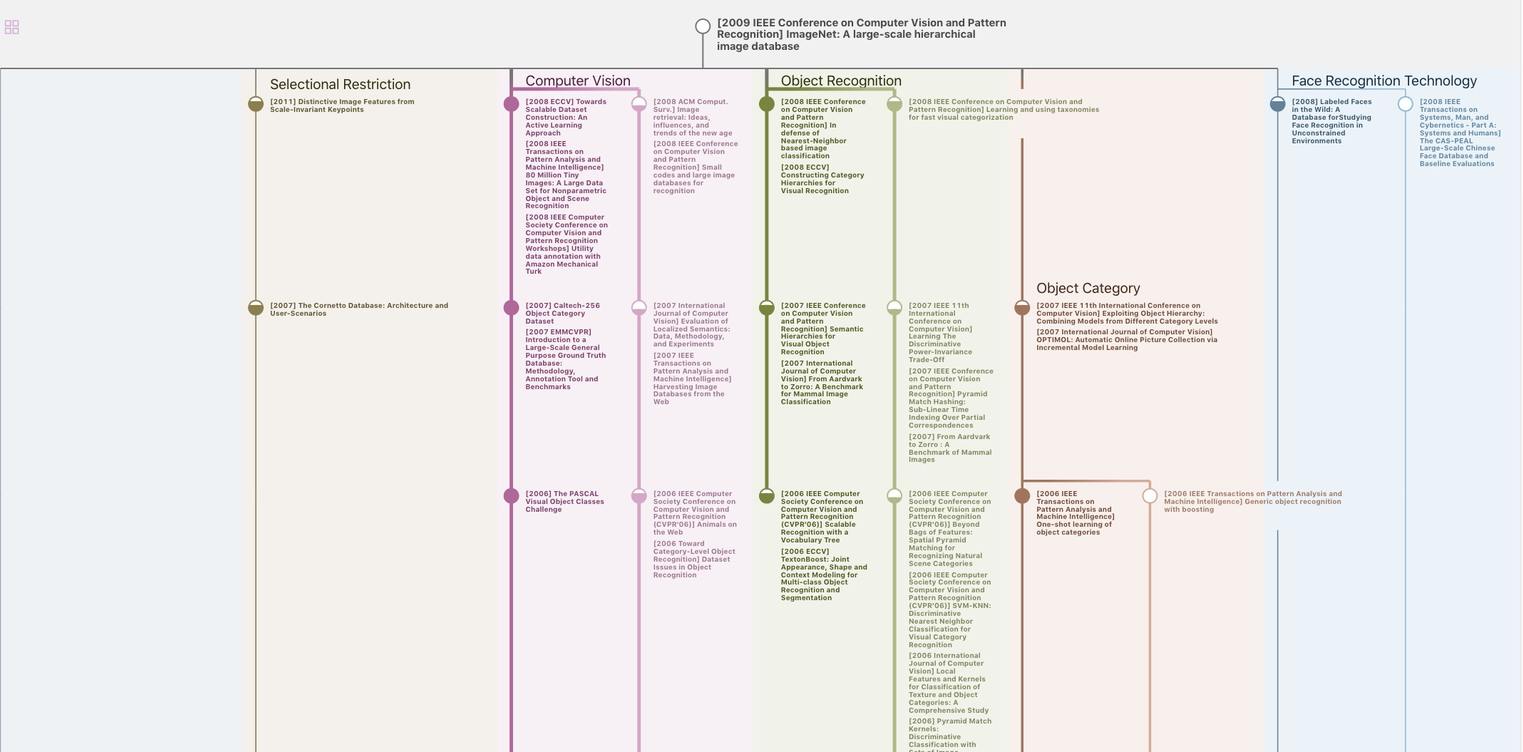
生成溯源树,研究论文发展脉络
Chat Paper
正在生成论文摘要