An Uncertainty-Driven and Observability-Based State Estimator for Nonholonomic Robots
IEEE TRANSACTIONS ON INSTRUMENTATION AND MEASUREMENT(2021)
摘要
The problem addressed in this article is the localization of a mobile robot using a combination of onboard sensors and ultrawideband (UWB) beacons. By using a discrete-time formulation of the system's kinematics, we identify the geometric conditions that make the system globally observable and cast the state estimation problem into the framework of least-squares optimization. The observability filter thus obtained is remarkably different from classic Bayesian filters, such as the Kalman Filter, since it does not need a priori stochastic models of process and measurement uncertainty contributions and thus proves to have better performance than the Kalman filters if such contributions are partly unknown or differ from the expected values. The second important outcome of this work is the analytical study of uncertainty propagation. The effectiveness of the designed filter, the validity of the theoretical analysis of estimation uncertainties, and the comparisons with a state-of-the-art extended Kalman filter (EKF) are corroborated by extensive simulations and validated experimentally.
更多查看译文
关键词
Extended Kalman filter (EKF), indoor localization, observability filter design, ranging measurements, uncertainty analysis
AI 理解论文
溯源树
样例
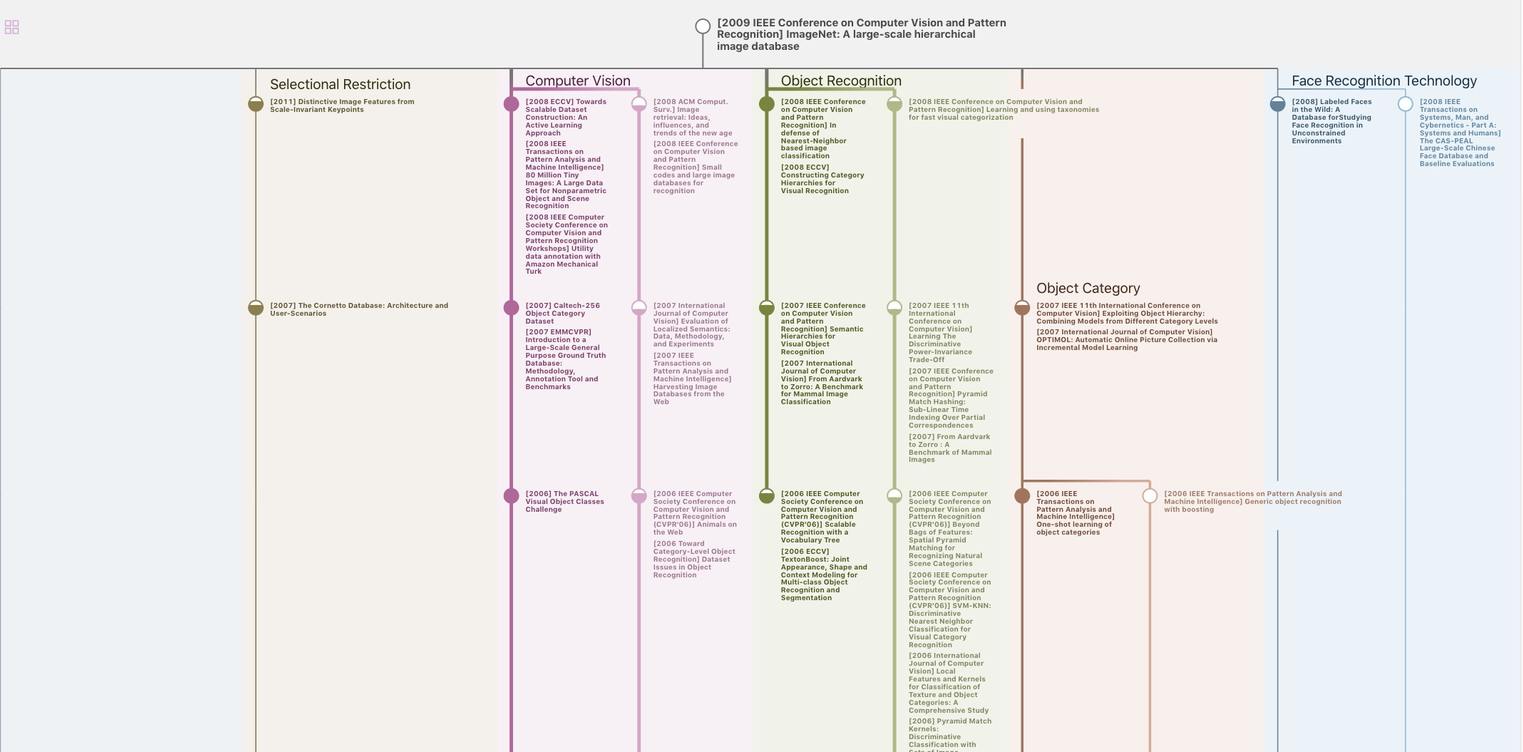
生成溯源树,研究论文发展脉络
Chat Paper
正在生成论文摘要