Analysis Of Classical And Machine Learning Based Short-Term And Mid-Term Load Forecasting For Smart Grid
INTERNATIONAL JOURNAL OF SUSTAINABLE ENERGY(2021)
摘要
The evolution of advanced metering infrastructure (AMI) has increased the electricity consumption data in real-time manifolds. Using this massive data, the load forecasting methods have undergone numerous transformations. In this paper, short-term and mid-term load forecasting (STLF and MTLF) is proposed using smart-metered data acquired from a real-life distribution grid at the NIT Patna campus with different classical and machine learning methods. Data pre-processing is done to transform the raw data into an appropriate format by removing the outliers present in the datasets. The influential meteorological variables obtained by correlation analysis along with the past load are used to train the load forecasting model. The proposed support vector regression (SVR) produces the best forecasting performance for the test system with a minimum mean absolute percentage error (MAPE) and root mean square error (RMSE). The proposed method outperforms the existing approaches for STLF and MTLF by an average MAPE of 3.60.
更多查看译文
关键词
Smart metering, short-term and mid-term load forecasting, MLR, ANN, optimised Holt's method, SVR
AI 理解论文
溯源树
样例
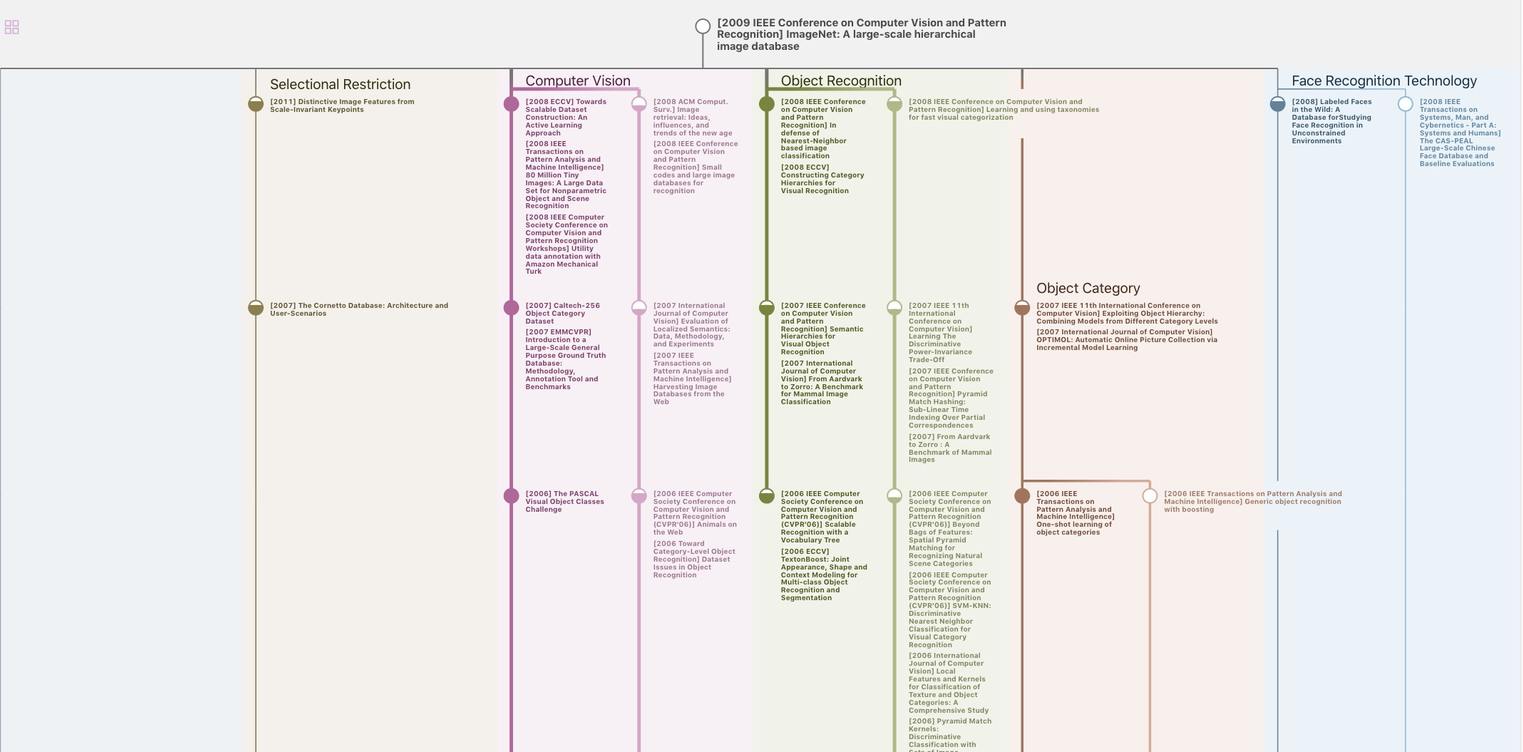
生成溯源树,研究论文发展脉络
Chat Paper
正在生成论文摘要