Combining Graph-Cut Clustering with Object-Based Stem Detection for Tree Segmentation in Highly Dense Airborne Lidar Point Clouds
ISPRS JOURNAL OF PHOTOGRAMMETRY AND REMOTE SENSING(2021)
关键词
Single tree segmentation,Stem detection,Graph-cut,Stopping criterion,Dense point cloud,Lidar
AI 理解论文
溯源树
样例
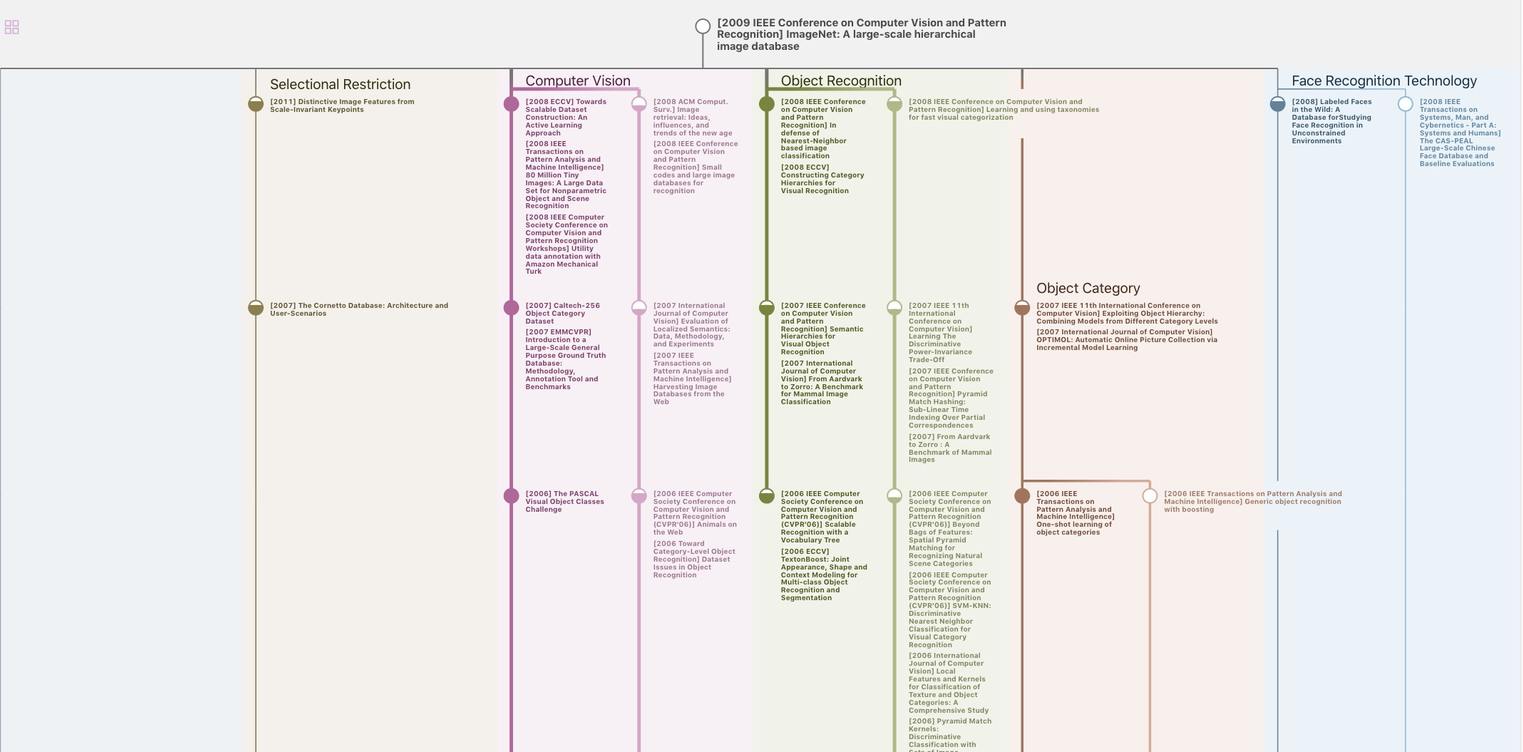
生成溯源树,研究论文发展脉络
Chat Paper
正在生成论文摘要