Image Inpainting Using Scene Constraints
SIGNAL PROCESSING-IMAGE COMMUNICATION(2021)
Abstract
Recent deep learning-based inpainting methods have shown significant improvements and generate plausible images. However, most of these methods may either synthesis unrealistic and blurry texture details or fail to capture object semantics. Furthermore, they employ huge models with inefficient mechanisms such as attention. Motivated by these observations, we propose a new end-to-end generative-based multi-stage architecture for image inpainting. Specifically, our model exploits the segmentation labels predictions to robustly reconstruct the object boundaries and avoid blurry or semantically incorrect images. Meanwhile, it employs edges predictions to recover the image structure. Different than previous approaches, we do not predict the segmentation labels/edges from the corrupted image. Instead, we employ a coarse image that contains more valuable global structure data. We conduct a set of extensive experiments to investigate the impact of merging these auxiliary pieces of information. Experiments show that our computationally efficient model achieves competitive qualitative and quantitative results compared to the state-of-the-art methods on multiple datasets.
MoreTranslated text
Key words
Image inpainting, Deep learning, Generative adversarial networks
AI Read Science
Must-Reading Tree
Example
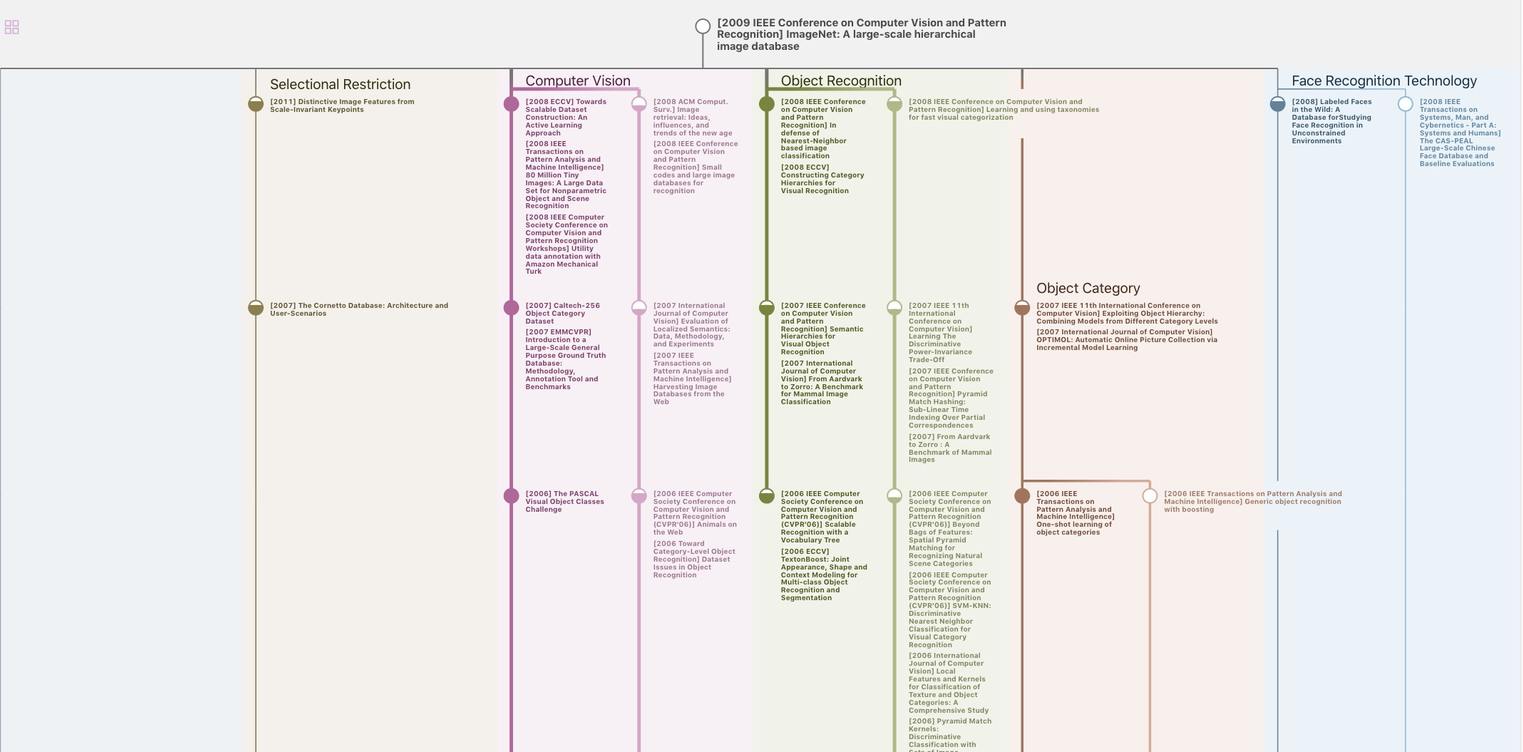
Generate MRT to find the research sequence of this paper
Chat Paper
Summary is being generated by the instructions you defined