Attentive multi-view deep subspace clustering net.
Neurocomputing(2021)
Abstract
In this paper, we propose a novel Attentive Multi-View Deep Subspace Nets (AMVDSN), which deeply explores underlying consistent and view-specific information from multiple views and fuse them by considering each view's dynamic contribution obtained by attention mechanism. Unlike most multi-view subspace learning methods that they directly reconstruct data points on raw data or only consider consistency or complementarity when learning representation in deep or shallow space, our proposed method seeks to find a joint latent representation that explicitly considers both consensus and view-specific information among multiple views, and then performs subspace clustering on learned joint latent representation.Besides, different views contribute differently to representation learning, we therefore introduce attention mechanism to derive dynamic weight for each view, which performs much better than previous fusion methods in the field of multi-view subspace clustering. The proposed algorithm is intuitive and can be easily optimized just by using Stochastic Gradient Descent (SGD) because of the neural network framework, which also provides strong non-linear characterization capability compared with traditional subspace clustering approaches. The experimental results on seven real-world data sets have demonstrated the effectiveness of our proposed algorithm against some state-of-the-art subspace learning approaches.
MoreTranslated text
Key words
Multi-view learning,Subspace clustering,Deep learning,Attention
AI Read Science
Must-Reading Tree
Example
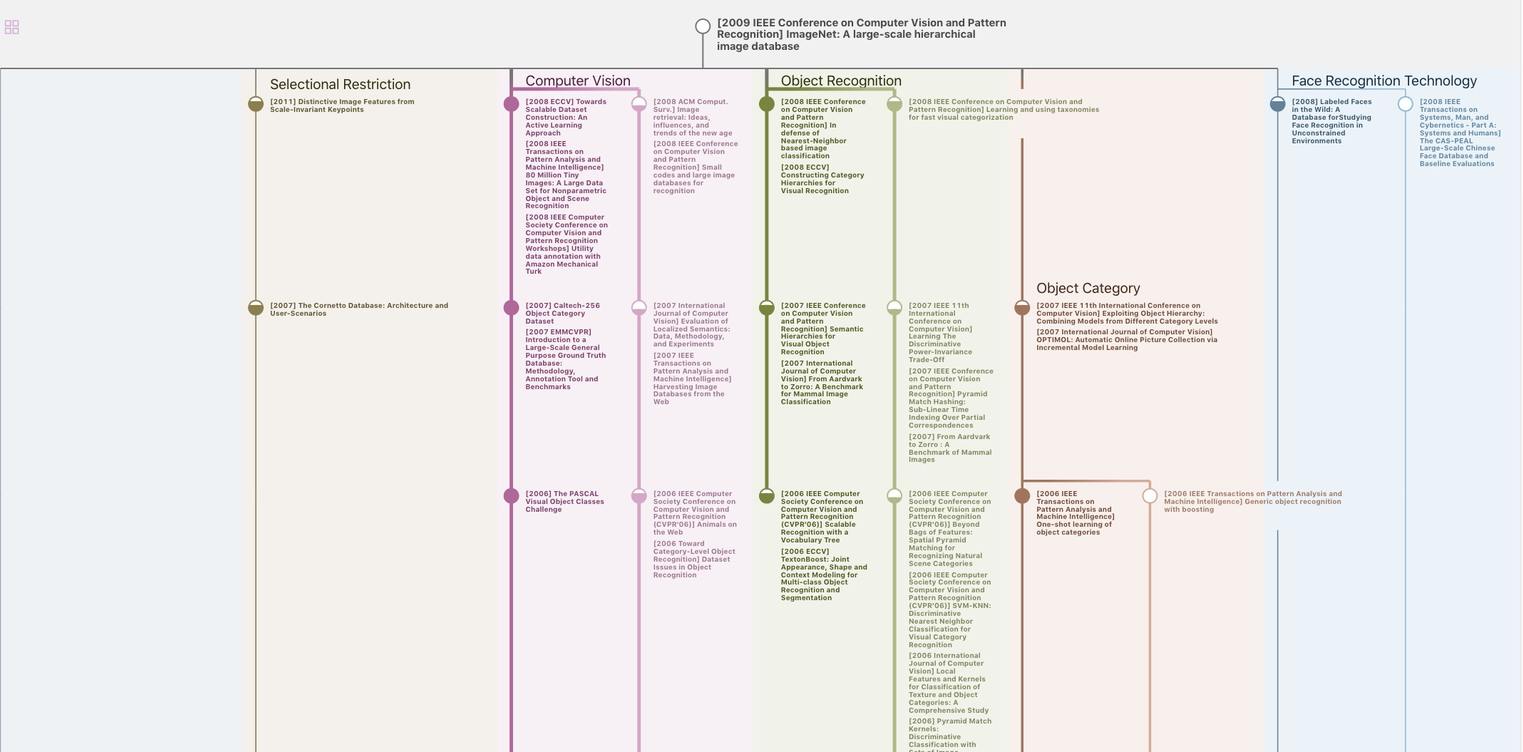
Generate MRT to find the research sequence of this paper
Chat Paper
Summary is being generated by the instructions you defined