Partial Least Squares Improved Multivariate Adaptive Regression Splines for Visible and Near-Infrared-Based Soil Organic Matter Estimation Considering Spatial Heterogeneity
APPLIED SCIENCES-BASEL(2021)
摘要
Under the influence of complex environmental conditions, the spatial heterogeneity of soil organic matter (SOM) is inevitable, and the relationship between SOM and visible and near-infrared (VNIR) spectra has the potential to be nonlinear. However, conventional VNIR-based methods for soil organic matter estimation cannot simultaneously consider the potential nonlinear relationship between the explanatory variables and predictors and the spatial heterogeneity of the relationship. Thus, the regional application of existing VNIR spectra-based SOM estimation methods is limited. This study combines the proposed partial least squares-based multivariate adaptive regression spline (PLS-MARS) method and a regional multi-variable associate rule mining and Rank-Kennard-Stone method (MVARC-R-KS) to construct a nonlinear prediction model to realize local optimality considering spatial heterogeneity. First, the MVARC-R-KS method is utilized to select representative samples and alleviate the sample global underrepresentation caused by spatial heterogeneity. Second, the PLS-MARS method is proposed to construct a nonlinear VNIR spectra-based estimation model with local optimization based on selected representative samples. PLS-MARS combined with the MVARC-R-KS method is illustrated and validated through a case study of Jianghan Plain in Hubei Province, China. Results showed that the proposed method far outweighs some available methods in terms of accuracy and robustness, suggesting the reliability of the proposed prediction model.
更多查看译文
关键词
soil organic matter,near-infrared spectroscopy,spatial heterogeneity,multivariate adaptive regression splines,partial least squares regression
AI 理解论文
溯源树
样例
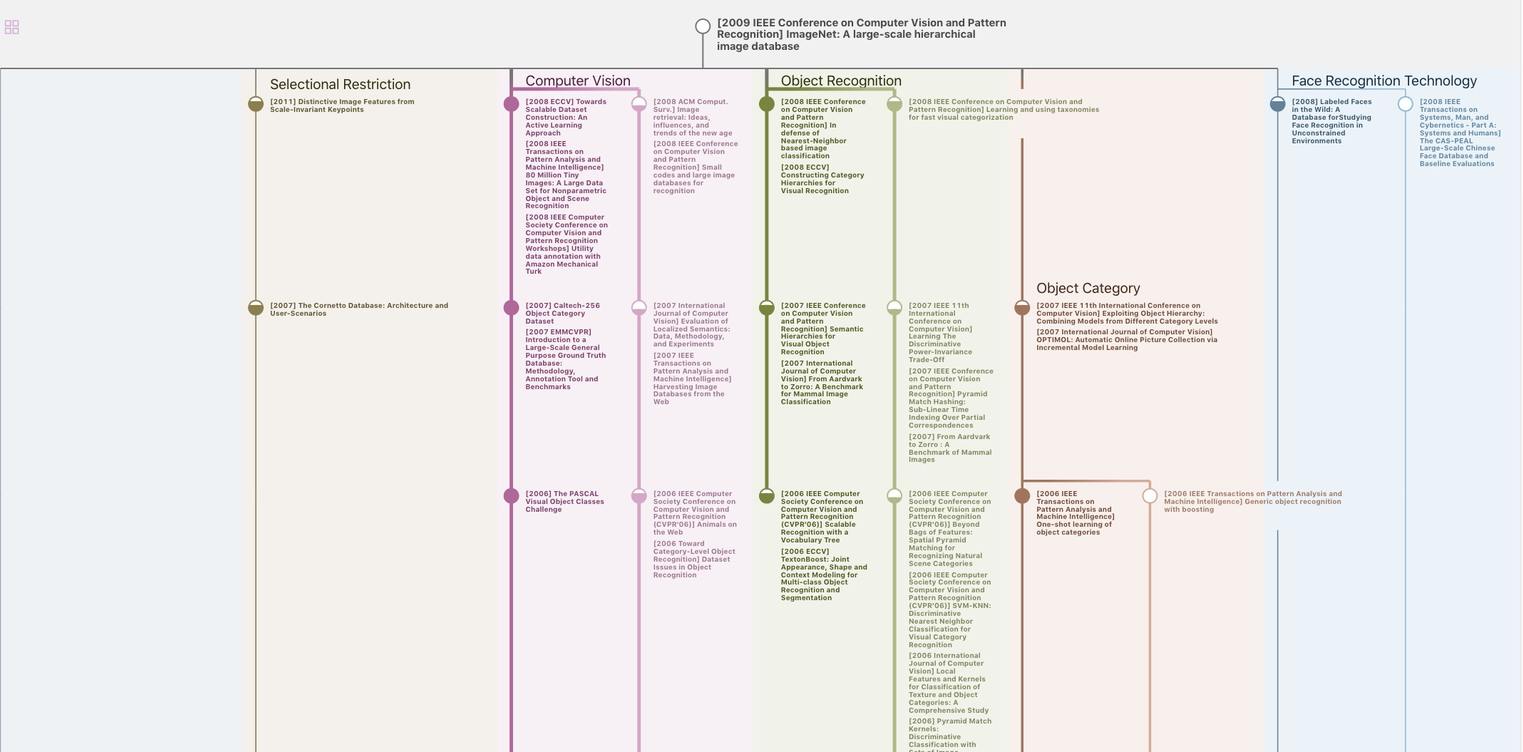
生成溯源树,研究论文发展脉络
Chat Paper
正在生成论文摘要