Semi-Skipping Layered Gated Unit And Efficient Network: Hybrid Deep Feature Selection Method For Edge Computing In Eeg-Based Emotion Classification
IEEE ACCESS(2021)
Abstract
In this article, we propose a novel feature selection method based on hybrid neural networks for emotional classification avoiding expensive computation. With the development of neural networks for machine learning tools, the Electroencephalogram (EEG)-based classification of human emotions is increasingly important in providing health-care for Edge Computing in support of multiple Internet of Things (IoT) applications. However, classifying emotions through EEG signals is very challenging due to the low temporal boundaries and non-linear nature of EEG signals. Regarding non-linearity, we propose a hierarchical Semi-skipping Layered Gated Unit (SLGU) besides Efficient Network (ENet) for feature extraction. For faster processing, we have introduced a semi-skipping layer for Gated Recurrent Units (GRU) in Recurrent Neural Networks (RNN). The entered layer automatically skips the divergent factor during network training. Preprocessed EEG signals are sent to the hybrid SLGU-ENet model for deep feature extraction. To overcome the computational cost, an optimal function reduction method called the Bag of Visualized Characteristics (BoVC) is used. The entire facility is verified against two publicly available datasets. The results show that the classification performance of the proposed model achieves superior classification precision in a short processing time compared to the state-of-the-art models. The proposed algorithm required around 1.2-5 seconds, suitable for real-time IoT applications.
MoreTranslated text
Key words
Electroencephalography, Feature extraction, Brain modeling, Internet of Things, Human computer interaction, Entropy, Emotion recognition, Edge computing, efficient network, electroencephalogram (EEG), emotion classification, Internet of Things, recurrent neural network
AI Read Science
Must-Reading Tree
Example
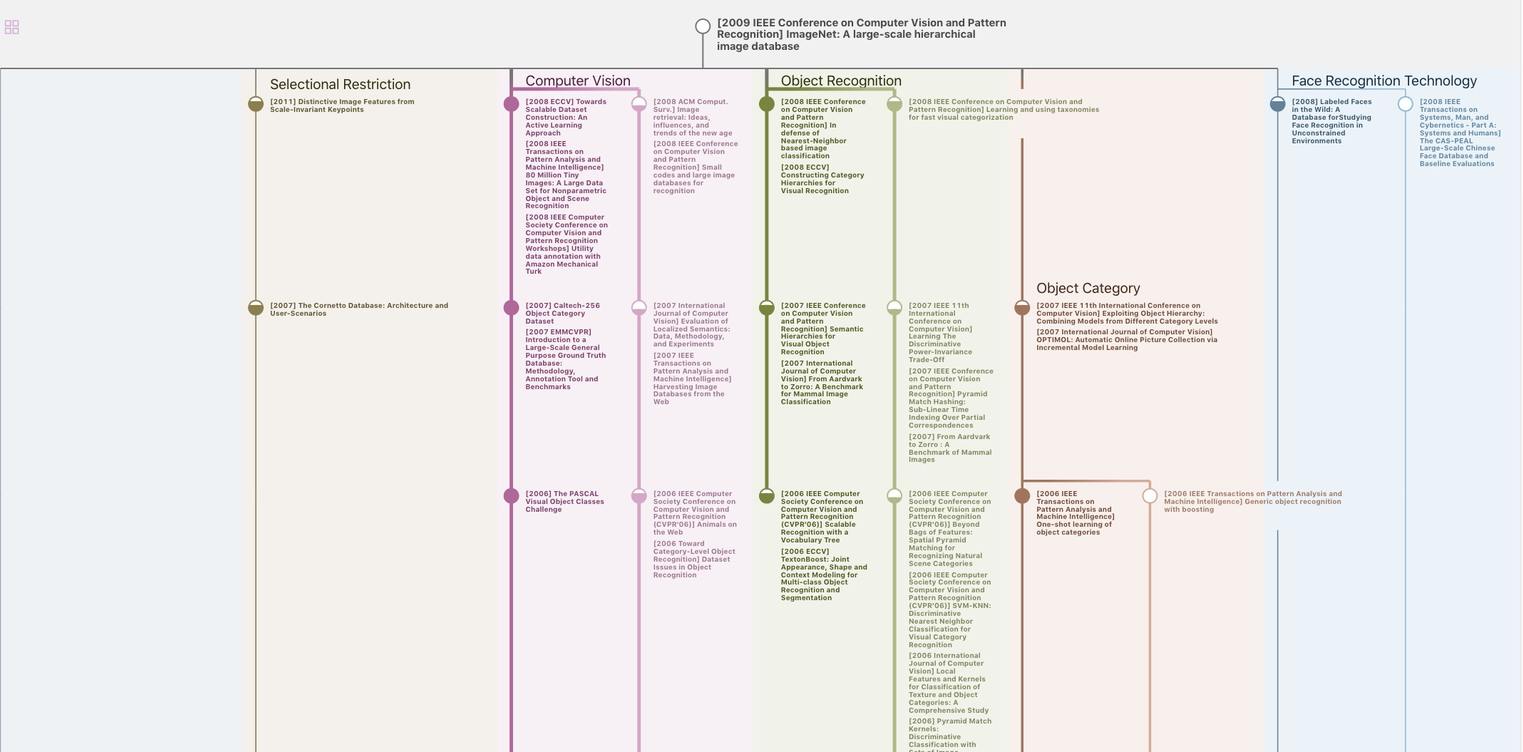
Generate MRT to find the research sequence of this paper
Chat Paper
Summary is being generated by the instructions you defined