In Situ Learning in Hardware Compatible Multilayer Memristive Spiking Neural Network
IEEE Transactions on Cognitive and Developmental Systems(2022)
摘要
As one of the most promising methods in the next generation of neuromorphic systems, memristor-based spiking neural networks (SNNs) show great advantages in terms of power efficiency, integration density, and biological plausibility. However, because of the nondifferentiability of discrete spikes, it is difficult to train SNNs with gradient descent and error backpropagation online. In this article, we propose an improved training algorithm for multilayer memristive SNN (MSNN) with three methods spontaneously, supporting
in situ
learning in hardware: 1) temporal order encoding is applied to generate different pulse trains in neurons; 2) a simplified homeostasis is realized by the activation state and refractory period to regulate hidden neurons spontaneously; and 3) spiking-timing-dependent plasticity (STDP) in memristive synapses is adopted to update weights
in situ
. Correspondingly, we provide a circuitry example and verify it in LTSPICE. Then the MSNN is benchmarked with the MNIST data set and analyzed with visualization methods, showing better recognition accuracy (95.15%) than existing SNNs with comparable scales and bio-inspired learning rules. We also consider some nonideal effects in memristor crossbar array and peripheral circuits. Evaluation results show that the proposed MSNN is robust to finite resolution, circuit noise and writing noise; and larger network scale will help the MSNN alleviate the negative impacts of other nonideal factors, including yield and device-to-device variation. Moreover, the energy efficiency of a MSNN system is estimated to achieve 7.6TOPS/W, showing great potential in low-power applications.
更多查看译文
关键词
Homeostasis,in situ,memristive SNN (MSNN),neuromorphic system,nonideal effect,spiking-timing-dependent plasticity (STDP),temporal order encoding
AI 理解论文
溯源树
样例
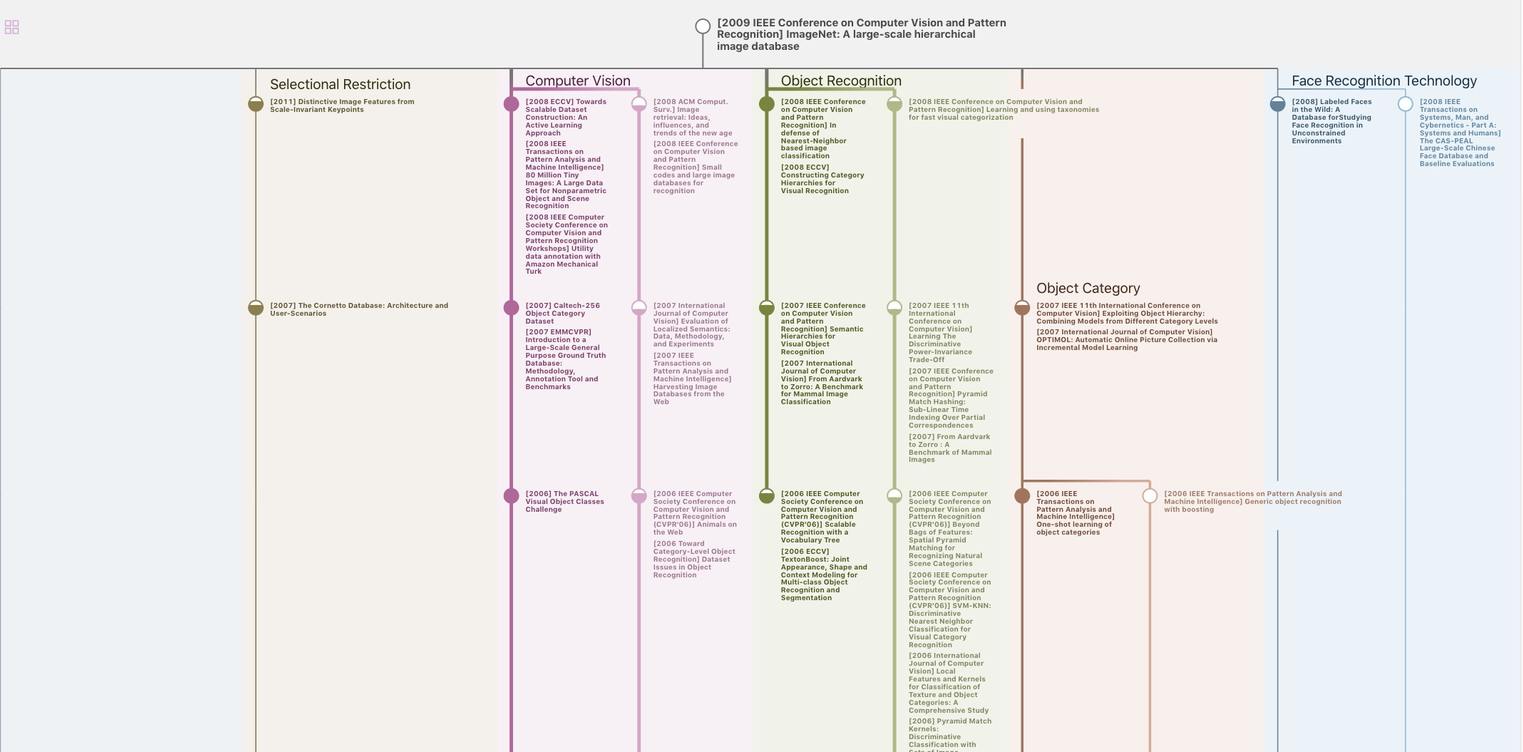
生成溯源树,研究论文发展脉络
Chat Paper
正在生成论文摘要