An efficient modified Hyperband and trust-region-based mode-pursuing sampling hybrid method for hyperparameter optimization
ENGINEERING OPTIMIZATION(2022)
Abstract
Although deep learning algorithms have been widely used, their performance depends heavily on a good set of hyperparameters. This article presents an efficient Hyperband and trust-region-based mode-pursuing sampling hybrid method for hyperparameter optimization. First, Hyperband is modified and used to select the optimum quickly from a large number of random sampling points to construct a trust region. Secondly, mode-pursuing sampling is performed in the trust region to generate more points systematically around the minimum, and the location or size of the trust region is dynamically adjusted to accelerate its convergence. Thirdly, the process of selection and sampling is repeated until a termination criterion is met. Numerical examples are presented to verify the effectiveness of the hybrid method, the results of which are compared with those of five well-known algorithms. Comparison results show that better optimal solutions are obtained through the hybrid method, with a higher efficiency.
MoreTranslated text
Key words
Hyperparameter optimization, Hyperband, mode-pursuing sampling, deep learning, trust region
AI Read Science
Must-Reading Tree
Example
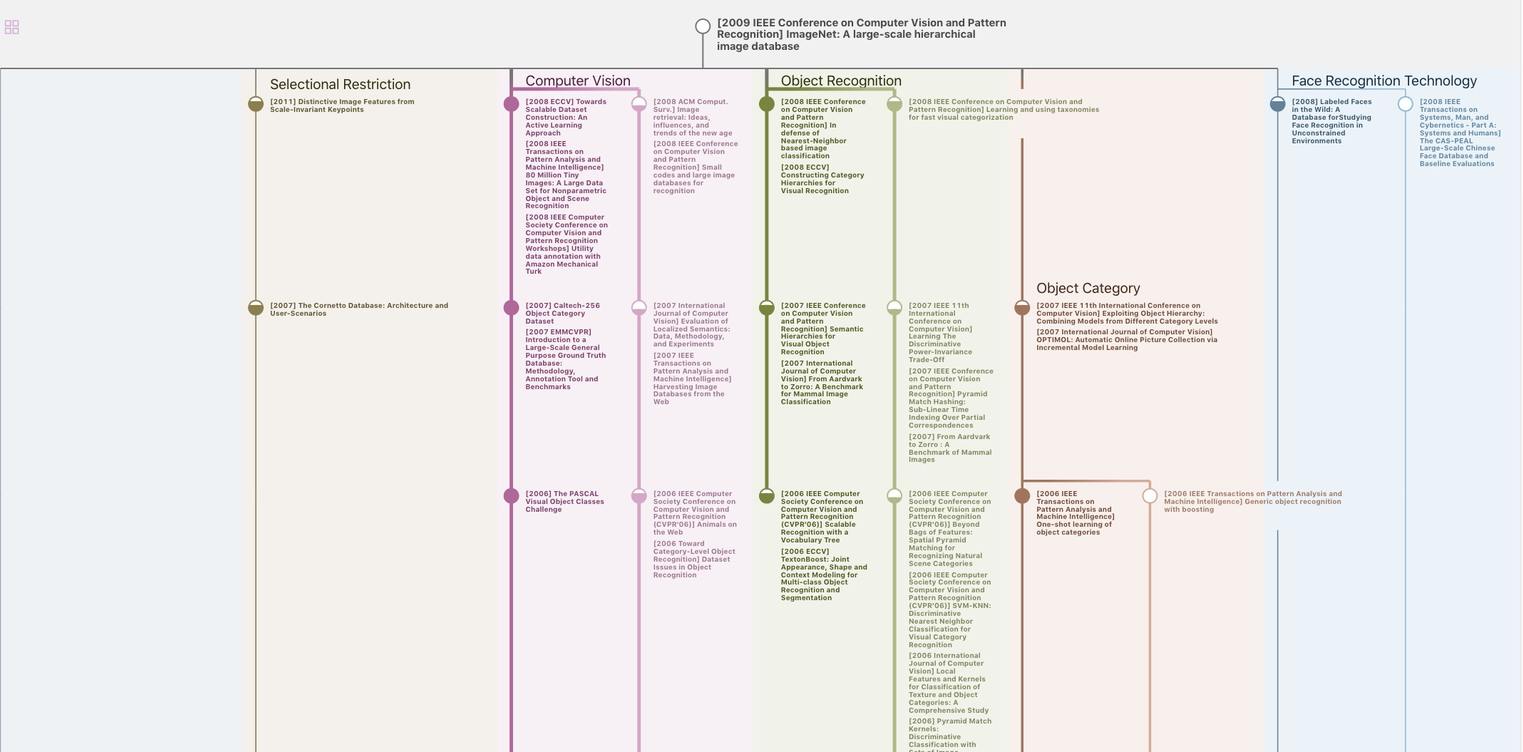
Generate MRT to find the research sequence of this paper
Chat Paper
Summary is being generated by the instructions you defined